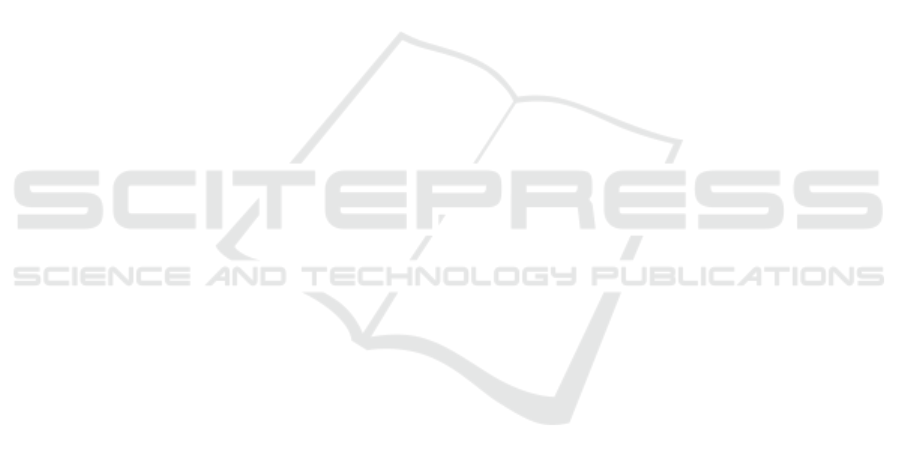
must be done to investigate if the claims made here
are more broadly applicable.
6 CONCLUSION & FUTURE
WORK
This paper seeks to answer the research question:
“How can the process of identifying yellow flags and
their signal terms in physiotherapeutic consultation
transcripts be automated?” The answer to this ques-
tion is that it is possible to automatically mark yel-
low flags and their signal terms in Physio Therapeutic
consultation transcripts by using an automated identi-
fication tool. Even though the tool constructed for this
paper was only able to mark yellow flags and signal
terms with a total precision of 13%, it reaches a recall
score of 72%. It can be said that the tool already does
well on retrieving real yellow flags and signal terms,
but it currently also retrieves too many irrelevant ones.
Due to time constraints the scope of this research was
narrow; more research needs to be conducted. Since
natural language is very ambiguous, more research
must be conducted to embed Natural Language Pro-
cessing (NLP) in the tool, to up the recall and the
precision scores. Also, more training data like ex-
amples of yellow flags and signal terms are needed,
and a standardized format must be established, stating
what components yellow flags consist of. The OS-
PRO, MPQ, PCS and HADS assessment guidelines
might be able to contribute to achieve this.
To conclude, it is safe to assume that automated iden-
tification of yellow flags and signal terms is possible
using the tool described in this research. However,
this is just the beginning, and much more research
must be done in the future to further enhance the tool,
aiming to improve the accuracy metrics. The recall
score of 72% can be improved, but the focus must be
on improving the precision score of 13%.
ACKNOWLEDGEMENTS
We want to thank Psychology researcher Wim van
Lankveld from the HAN University of Applied Sci-
ences for the interview, guidance, and providing us
with the transcripts and other relevant information.
Ethical approval for the data collection of this
study was given by the Ethical Research Committee
of the HAN University of Applied Sciences in Ni-
jmegen, the Netherlands (EACO 145.04/19).
REFERENCES
Barron, C. J., Klaber Moffett, J. A., and Potter, M. (2007).
Patient expectations of physiotherapy: definitions,
concepts, and theories. Physiotherapy theory and
practice, 23(1):37–46.
Bukman, B. (2019). ’zorgverleners besteden 40 procent van
hun tijd aan administratie’.
Carballo-Costa, L., Michaleff, Z. A., Costas, R., Quintela-
del R
´
ıo, A., Vivas-Costa, J., and Moseley, A. M.
(2022). Evolution of the thematic structure and main
producers of physical therapy interventions research:
A bibliometric analysis (1986 to 2017). Brazilian
Journal of Physical Therapy, 26(4):100429.
Dalpiaz, F., Van Der Schalk, I., Brinkkemper, S., Aydemir,
F. B., and Lucassen, G. (2019). Detecting terminolog-
ical ambiguity in user stories: Tool and experimenta-
tion. Information and Software Technology, 110:3–16.
Demetros, C. (2021). Signal words: 5 fun ways to explain
these sentence superheroes!
Hoy, D., Brooks, P., Blyth, F., and Buchbinder, R. (2010).
The epidemiology of low back pain. Best practice &
research Clinical rheumatology, 24(6):769–781.
Kendall, N. (1997). Guide to assessing psychosocial yellow
flags in acute low back pain. Risk factors for long-term
disability and work loss.
Leerar, P. J., Boissonnault, W., Domholdt, E., and Roddey,
T. (2007). Documentation of red flags by physical
therapists for patients with low back pain. Journal
of Manual & Manipulative Therapy, 15(1):42–49.
Lentz, T. A., Beneciuk, J. M., Bialosky, J. E., Zeppieri Jr,
G., Dai, Y., Wu, S. S., and George, S. Z. (2016). De-
velopment of a yellow flag assessment tool for or-
thopaedic physical therapists: results from the optimal
screening for prediction of referral and outcome (os-
pro) cohort. journal of orthopaedic & sports physical
therapy, 46(5):327–343.
Luque, C., Luna, J. M., Luque, M., and Ventura, S. (2019).
An advanced review on text mining in medicine. Wiley
Interdisciplinary Reviews: Data Mining and Knowl-
edge Discovery, 9(3):e1302.
Maas, L., Geurtsen, M., Nouwt, F., Schouten, S. F.,
Van De Water, R., Van Dulmen, S., Dalpiaz, F.,
Van Deemter, K., and Brinkkemper, S. (2020). The
care2report system: automated medical reporting as
an integrated solution to reduce administrative burden
in healthcare. In Information Technology in Health-
care: IT Architectures and Implementations in Health-
care Environments. Hawaii International Conference
on System Sciences (HICSS).
Main, C. J. (2013). Yellow flags.
Milojevi
´
c, S., Sugimoto, C. R., Yan, E., and Ding, Y.
(2011). The cognitive structure of library and infor-
mation science: Analysis of article title words. Jour-
nal of the American Society for Information Science
and Technology, 62(10):1933–1953.
Moffett, J. and McLean, S. (2006). The role of physiother-
apy in the management of non-specific back pain and
neck pain. Rheumatology, 45(4):371–378.
Automated Identification of Yellow Flags and Their Signal Terms in Physiotherapeutic Consultation Transcripts
535