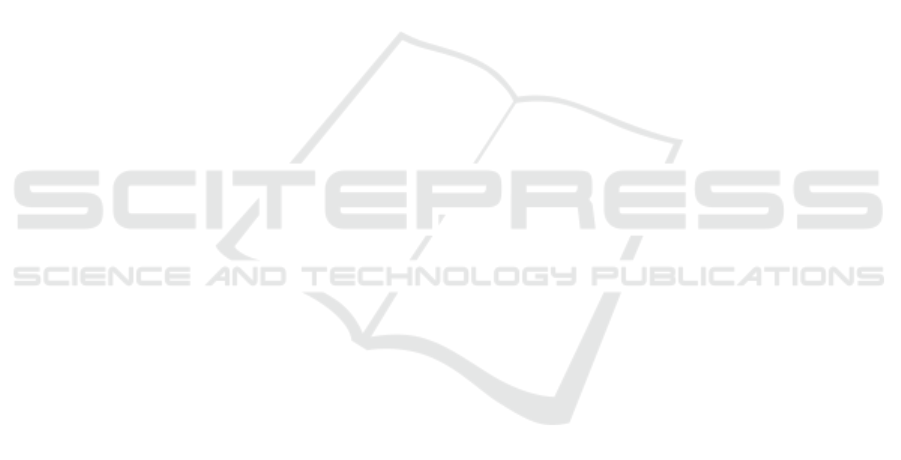
sented are only preliminary. The idea of cherry pick-
ing the most adequate channels among different color
spaces seems to indicate that this approach can lead to
superior results. However a number of further steps
needs to be taken in order to ensure the reproducibil-
ity and levels of accuracy could be ensured. The most
immediate problem is regarding the data. As one can
see in the results section it was identified that there
is a good change the annotation process available in
the used datasets are not necessarily precise leading to
miss-classification. In order to address this issue we
are currently working on a huge multi-spectral dataset
featuring invasive plants. The aforementioned dataset
is been manually annotated by a pool of specialists
and it will be made publicly available as soon as it
is complete and validated. A further development to
ensure the accuracy of the produced groundtruth is to
provide the expects with vegetation index images in
order to aid and further guide them during the anno-
tation process.
ACKNOWLEDGEMENTS
The authors would like to thank the company Sen-
six Inovac¸
˜
oes em Drones Ltda (http://sensix.com.br)
for providing the images used in the tests. Andr
´
e R.
Backes gratefully acknowledges the financial support
of CNPq (National Council for Scientific and Techno-
logical Development, Brazil) (Grant #307100/2021-
9). This study was financed in part by the
Coordenac¸
˜
ao de Aperfeic¸oamento de Pessoal de
N
´
ıvel Superior - Brazil (CAPES) - Finance Code 001.
REFERENCES
Ansari, A. M. and Singh, D. K. (2022). Significance of
color spaces and their selection for image processing:
A survey. Recent Advances in Computer Science and
Communications (Formerly: Recent Patents on Com-
puter Science), 15(7):946–956.
Bi, Z. and Cao, P. (2021). Color space conversion algorithm
and comparison study. Journal of Physics: Confer-
ence Series, 1976(1):012008.
Bucci, G., Bentivoglio, D., and Finco, A. (2018). Pre-
cision agriculture as a driver for sustainable farming
systems: state of art in literature and research. Cali-
tatea, 19(S1):114–121.
Chaurasia, A. and Culurciello, E. (2017). Linknet: Exploit-
ing encoder representations for efficient semantic seg-
mentation. In 2017 IEEE Visual Communications and
Image Processing (VCIP), pages 1–4.
de Carvalho, L. B. (2013). Plantas Daninhas, volume 1. 1st
edition.
Elharrouss, O., Akbari, Y., Almaadeed, N., and Al-
Maadeed, S. (2022). Backbones-review: Feature
extraction networks for deep learning and deep re-
inforcement learning approaches. arXiv preprint
arXiv:2206.08016.
Erunova, M. G., Pisman, T. I., and Shevyrnogov, A. P.
(2021). The technology for detecting weeds in agri-
cultural crops based on vegetation index vari (plan-
etscope). Journal of Siberian Federal University. En-
gineering & Technologies, 14(3):347–353.
Fu, H., Wang, B., Shen, J., Cui, S., Xu, Y., Liu, J., and Shao,
L. (2019). Evaluation of Retinal Image Quality As-
sessment Networks in Different Color-Spaces, pages
48–56.
Hasan, A. S. M. M., Sohel, F., Diepeveen, D., Laga, H., and
Jones, M. G. (2021). A survey of deep learning tech-
niques for weed detection from images. Computers
and Electronics in Agriculture, 184:106067.
Hastings, G. D. and Rubin, A. (2012). Color spaces - a
review of historic and modern colour models. African
Vision and Eye Health, 71(3).
Jafarbiglu, H. and Pourreza, A. (2022). A comprehensive
review of remote sensing platforms, sensors, and ap-
plications in nut crops. Computers and Electronics in
Agriculture, 197:106844.
Mahy, M., Van Mellaert, B., Van Eycken, L., and Oost-
erlinck, A. (1991). The influence of uniform color
spaces on color image-processing-a comparative-
study of cielab, cieluv, and atd. Journal of imaging
technology, 17(5):232–243.
Meil
˘
a, M. (2003). Comparing clusterings by the variation of
information. In Learning theory and kernel machines,
pages 173–187. Springer.
Milioto, A., Lottes, P., and Stachniss, C. (2018). Real-time
semantic segmentation of crop and weed for precision
agriculture robots leveraging background knowledge
in cnns. In 2018 IEEE International Conference on
Robotics and Automation (ICRA), pages 2229–2235.
Molin, J. P. (2017). Agricultura de precis
˜
ao: N
´
umeros do
mercado brasileiro. Technical report, USP.
Monteiro, A. and von Wangenheim, A. (2019). Orthomo-
saic dataset of rgb aerial images for weed mapping.
http://www.lapix.ufsc.br/weed-mapping-sugar-cane.
Nachiluk, K. (2022). Alta na prodduc¸
˜
ao e exportac¸
˜
oes de
ac¸ucar marcam a safra 2020/21 de cana. an
´
alises e in-
dicadores o agroneg
´
ocio. Electronically. v. 16 n. 16.
Osorio, K., Puerto, A., Pedraza, C., Jamaica, D., and
Rodr
´
ıguez, L. (2020). A deep learning approach for
weed detection in lettuce crops using multispectral
images. AgriEngineering, 2(3):471–488.
Packyanathan, G., Veeraraghavalu, R., B.S, S., Kalist, V.,
and Basha, S. (2015). Satellite image segmentation
based on ycbcr color space. Indian Journal of Science
and Technology, 8:35.
Pandit, S., Gupta, S., et al. (2011). A comparative study
on distance measuring approaches for clustering. In-
ternational journal of research in computer science,
2(1):29–31.
Pereira Junior, P. and von Wangenheim, A. (2019). Or-
thomosaic dataset of rgb aerial images for crop rows
Multichannel Analysis in Weed Detection
425