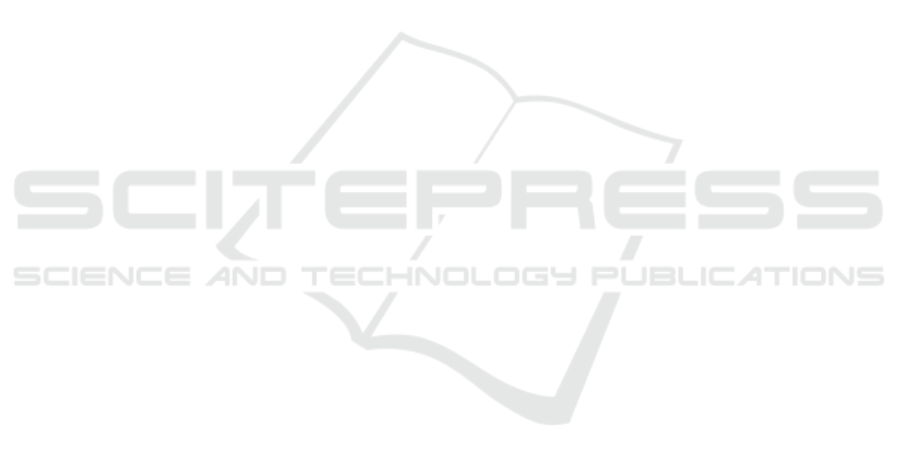
generated agent’s behaviors obtained from our predic-
tive models to assess the quality of the interaction and
the perception of the agent.
REFERENCES
Ashenfelter, K. T., Boker, S. M., Waddell, J. R., and Vi-
tanov, N. (2009). Spatiotemporal symmetry and mul-
tifractal structure of head movements during dyadic
conversation. Journal of Experimental Psychology:
Human Perception and Performance, 35(4):1072.
Bakhshayesh, H., Fitzgibbon, S. P., Janani, A. S., Grum-
mett, T. S., and Pope, K. J. (2019). Detecting syn-
chrony in eeg: A comparative study of functional
connectivity measures. Computers in biology and
medicine, 105:1–15.
Baltrusaitis, T., Zadeh, A., Lim, Y. C., and Morency, L.-
P. (2018). Openface 2.0: Facial behavior analysis
toolkit. In 2018 13th IEEE international conference
on automatic face & gesture recognition (FG 2018),
pages 59–66. IEEE.
Be
ˇ
nu
ˇ
s,
ˇ
S., Gravano, A., and Hirschberg, J. (2011). Prag-
matic aspects of temporal accommodation in turn-
taking. Journal of Pragmatics, 43(12):3001–3027.
Bernieri, F. J., Reznick, J. S., and Rosenthal, R.
(1988). Synchrony, pseudosynchrony, and dissyn-
chrony: measuring the entrainment process in mother-
infant interactions. Journal of personality and social
psychology, 54(2):243.
Bernieri, F. J. and Rosenthal, R. (1991). Interpersonal co-
ordination: Behavior matching and interactional syn-
chrony.
Bernstein, M. J., Sacco, D. F., Brown, C. M., Young, S. G.,
and Claypool, H. M. (2010). A preference for genuine
smiles following social exclusion. Journal of experi-
mental social psychology, 46(1):196–199.
Biancardi, B., Cafaro, A., and Pelachaud, C. (2017). An-
alyzing first impressions of warmth and competence
from observable nonverbal cues in expert-novice in-
teractions. In Proceedings of the 19th acm interna-
tional conference on multimodal interaction, pages
341–349.
Boker, S. M., Rotondo, J. L., Xu, M., and King, K. (2002).
Windowed cross-correlation and peak picking for the
analysis of variability in the association between be-
havioral time series. Psychological methods, 7(3):338.
Burgoon, J. K., Stern, L. A., and Dillman, L. (1995). In-
terpersonal adaptation: Dyadic interaction patterns.
Cambridge University Press.
Cafaro, A., Wagner, J., Baur, T., Dermouche, S., Torres Tor-
res, M., Pelachaud, C., Andr
´
e, E., and Valstar, M.
(2017). The noxi database: multimodal recordings of
mediated novice-expert interactions. In Proceedings
of the 19th ACM International Conference on Multi-
modal Interaction, pages 350–359.
Campbell, N. (2008). Multimodal processing of discourse
information; the effect of synchrony. In 2008 Second
International Symposium on Universal Communica-
tion, pages 12–15. IEEE.
Cappella, J. N. (1997). Behavioral and judged coordina-
tion in adult informal social interactions: Vocal and
kinesic indicators. Journal of personality and social
psychology, 72(1):119.
Chang, W. I. and Lawler, E. L. (1994). Sublinear approxi-
mate string matching and biological applications. Al-
gorithmica, 12(4):327–344.
Chartrand, T. L. and Bargh, J. A. (1999). The chameleon
effect: the perception–behavior link and social inter-
action. Journal of personality and social psychology,
76(6):893.
Chartrand, T. L. and Lakin, J. L. (2013). The antecedents
and consequences of human behavioral mimicry. An-
nual review of psychology, 64:285–308.
Condon, W. S. and Ogston, W. D. (1966). Sound film
analysis of normal and pathological behavior patterns.
Journal of nervous and mental disease.
Condon, W. S. and Ogston, W. D. (1967). A segmen-
tation of behavior. Journal of psychiatric research,
5(3):221–235.
Condon, W. S. and Sander, L. W. (1974). Neonate move-
ment is synchronized with adult speech: Interac-
tional participation and language acquisition. Science,
183(4120):99–101.
Cuddy, A. J., Glick, P., and Beninger, A. (2011). The dy-
namics of warmth and competence judgments, and
their outcomes in organizations. Research in orga-
nizational behavior, 31:73–98.
Delaherche, E. and Chetouani, M. (2010). Multimodal co-
ordination: exploring relevant features and measures.
In Proceedings of the 2nd international workshop on
Social signal processing, pages 47–52.
Delaherche, E., Chetouani, M., Mahdhaoui, A., Saint-
Georges, C., Viaux, S., and Cohen, D. (2012). In-
terpersonal synchrony: A survey of evaluation meth-
ods across disciplines. IEEE Transactions on Affective
Computing, 3(3):349–365.
Dermouche, S. and Pelachaud, C. (2019). Engagement
modeling in dyadic interaction. In 2019 interna-
tional conference on multimodal interaction, pages
440–445.
Ekman, P. (1992). An argument for basic emotions. Cogni-
tion & emotion, 6(3-4):169–200.
Ekman, P. and Friesen, W. (1982). Felt, false, miserable
smiles. Journal of Nonverbal Behavior, 6(4):238–251.
Hale, J., Ward, J. A., Buccheri, F., Oliver, D., and Hamilton,
A. F. d. C. (2020). Are you on my wavelength? inter-
personal coordination in dyadic conversations. Jour-
nal of nonverbal behavior, 44(1):63–83.
Heimerl, A., Baur, T., Lingenfelser, F., Wagner, J., and
Andr
´
e, E. (2019). Nova - a tool for explainable co-
operative machine learning. In 2019 8th International
Conference on Affective Computing and Intelligent
Interaction (ACII), 3-6 September 2019, Cambridge,
UK, pages 109 – 115.
Hess, U., Beaupr
´
e, M. G., Cheung, N., et al. (2002). Who
to whom and why–cultural differences and similarities
ICAART 2023 - 15th International Conference on Agents and Artificial Intelligence
124