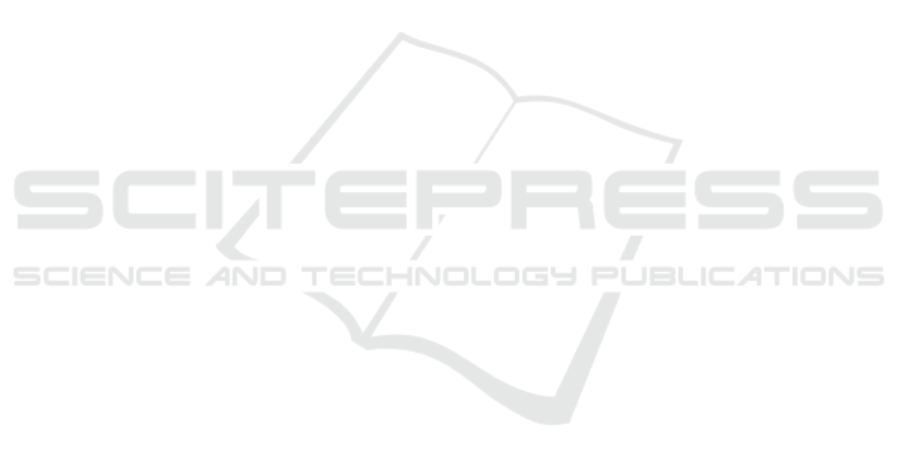
of patients enrolled in the study and send text mes-
sages to patients who are not enrolled in the study.
ACKNOWLEDGEMENTS
The authors would like to acknowledge the cardio-
thoracic service of the Santa Marta Hospital for their
contributions to carry out the study. This research has
been supported by the project DSAIPA/AI/0094/2020
from the Fundac¸
˜
ao para a Ci
ˆ
encia e Tecnologia AI 4
COVID-19 Program.
REFERENCES
Akinosun, A. S., Polson, R., Diaz Skeete, Y., De Kock,
J. H., Carragher, L., Leslie, S., Grindle, M., and
Gorely, T. (2021). Digital technology interventions
for risk factor modification in patients with cardiovas-
cular disease: Systematic review and meta-analysis.
JMIR mHealth and uHealth, 9(3):e21061.
Bauer, U. E., Briss, P. A., Goodman, R. A., and Bowman,
B. A. (2014). Prevention of chronic disease in the 21st
century: elimination of the leading preventable causes
of premature death and disability in the USA. The
Lancet, 384(9937):45–52.
Brug, J. (2008). Determinants of healthy eating: motiva-
tion, abilities and environmental opportunities. Family
Practice, 25:i50–i55.
Cadilhac, D. A., Andrew, N. E., Busingye, D., Cameron,
J., Thrift, A. G., Purvis, T., Li, J. C., Kneebone, I.,
Thijs, V., Hackett, M. L., Lannin, N. A., Kilkenny,
M. F., and on behalf of the ReCAPS investigators
(2020). Pilot randomised clinical trial of an eHealth,
self-management support intervention (iVERVE) for
stroke: feasibility assessment in survivors 12–24
months post-event. Pilot and Feasibility Studies,
6(1):172.
Carey, R. N., Connell, L. E., Johnston, M., Rothman, A. J.,
de Bruin, M., Kelly, M. P., and Michie, S. (2018). Be-
havior change techniques and their mechanisms of ac-
tion: A synthesis of links described in published inter-
vention literature. Annals of Behavioral Medicine.
Cena, H. and Calder, P. C. (2020). Defining a healthy diet:
Evidence for the role of contemporary dietary patterns
in health and disease. Nutrients, 12(2):334.
Chaix, B., Bibault, J.-E., Pienkowski, A., Delamon, G.,
Guillemass
´
e, A., Nectoux, P., and Brouard, B. (2019).
When chatbots meet patients: One-year prospective
study of conversations between patients with breast
cancer and a chatbot. JMIR Cancer, 5(1):e12856.
Chokshi, N. P., Adusumalli, S., Small, D. S., Morris, A.,
Feingold, J., Ha, Y. P., Lynch, M. D., Rareshide, C.
A. L., Hilbert, V., and Patel, M. S. (2018). Loss-
framed financial incentives and personalized goal-
setting to increase physical activity among ischemic
heart disease patients using wearable devices: The
ACTIVE REWARD randomized trial. Journal of the
American Heart Association, 7(12):e009173.
Fitzpatrick, K. K., Darcy, A., and Vierhile, M. (2017). De-
livering cognitive behavior therapy to young adults
with symptoms of depression and anxiety using a fully
automated conversational agent (woebot): A random-
ized controlled trial. JMIR Mental Health, 4(2):e19.
Gardner, G., Elliott, D., Gill, J., Griffin, M., and Crawford,
M. (2005). Patient experiences following cardiotho-
racic surgery: An interview study. European Journal
of Cardiovascular Nursing, 4(3):242–250.
Ghandeharioun, A., McDuff, D., Czerwinski, M., and
Rowan, K. (2019). EMMA: An emotion-aware well-
being chatbot. In 2019 8th International Confer-
ence on Affective Computing and Intelligent Interac-
tion (ACII), pages 1–7. IEEE.
Grimmett, C., Corbett, T., Brunet, J., Shepherd, J., Pinto,
B. M., May, C. R., and Foster, C. (2019). Systematic
review and meta-analysis of maintenance of physical
activity behaviour change in cancer survivors. Inter-
national Journal of Behavioral Nutrition and Physical
Activity, 16(1):37.
Halldorsdottir, H., Thoroddsen, A., and Ingadottir, B.
(2020). Impact of technology-based patient education
on modifiable cardiovascular risk factors of people
with coronary heart disease: A systematic review. Pa-
tient Education and Counseling, 103(10):2018–2028.
Hevner, March, Park, and Ram (2004). Design science
in information systems research. MIS Quarterly,
28(1):75.
Horner, G. N., Agboola, S., Jethwani, K., Tan-McGrory, A.,
and Lopez, L. (2017). Designing patient-centered text
messaging interventions for increasing physical activ-
ity among participants with type 2 diabetes: Qualita-
tive results from the text to move intervention. JMIR
mHealth and uHealth, 5(4):e54.
Johnston, M., Carey, R. N., Connell Bohlen, L. E., John-
ston, D. W., Rothman, A. J., de Bruin, M., Kelly,
M. P., Groarke, H., and Michie, S. (2021). Devel-
opment of an online tool for linking behavior change
techniques and mechanisms of action based on trian-
gulation of findings from literature synthesis and ex-
pert consensus. Translational Behavioral Medicine,
11(5):1049–1065.
Kelly, J. T., Conley, M., Hoffmann, T., Craig, J. C., Tong,
A., Reidlinger, D. P., Reeves, M. M., Howard, K., Kr-
ishnasamy, R., Kurtkoti, J., Palmer, S. C., Johnson,
D. W., and Campbell, K. L. (2020). A coaching pro-
gram to improve dietary intake of patients with CKD:
ENTICE-CKD. Clinical Journal of the American So-
ciety of Nephrology, 15(3):330–340.
Kontis, V., Mathers, C. D., Rehm, J., Stevens, G. A., Shield,
K. D., Bonita, R., Riley, L. M., Poznyak, V., Beagle-
hole, R., and Ezzati, M. (2014). Contribution of six
risk factors to achieving the 25x25 non-communicable
disease mortality reduction target: a modelling study.
The Lancet, 384(9941):427–437.
Londral, A., Azevedo, S., Dias, P., Ramos, C., Santos, J.,
Martins, F., Silva, R., Semedo, H., Vital, C., Gualdino,
A., Falc
˜
ao, J., Lap
˜
ao, L. V., Coelho, P., and Fragata,
Designing a Digital Personal Coach to Promote a Healthy Diet and Physical Activity Among Patients After Cardiothoracic Surgery
601