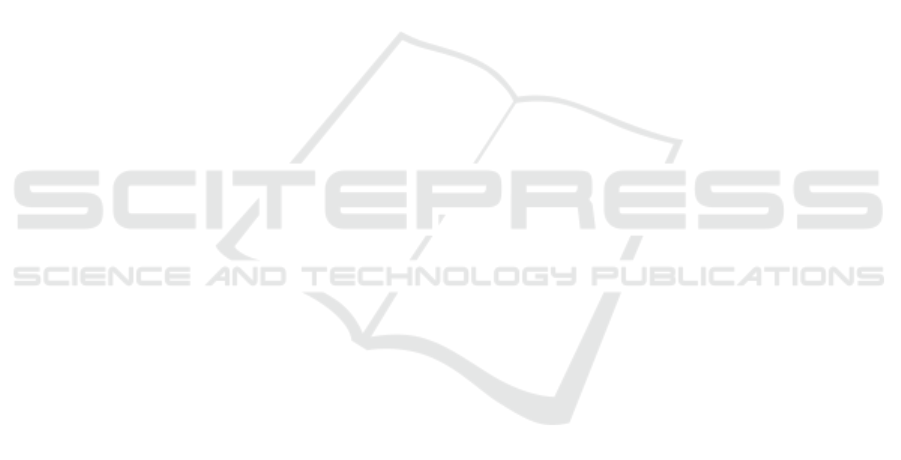
present, and future. Resuscitation, 165(May):101–
109.
Research, C. I. o. H. (2019). Research program intended to
help canadians survive sudden cardiac arrest expands
to all 10 canadian provinces - CIHR.
Riou, M., Ball, S., O’Halloran, K. L., Whiteside, A.,
Williams, T. A., Finn, J., O’Halloran, K. L., White-
side, A., Williams, T. A., and Finn, J. (2018a). Hi-
jacking the dispatch protocol: When callers pre-empt
their reason-for-the-call in emergency calls about car-
diac arrest. Discourse Studies, 20(5):666–687.
Riou, M., Ball, S., Williams, T. A., Whiteside, A., Cameron,
P., Fatovich, D. M., Perkins, G. D., Smith, K., Bray,
J., Inoue, M., O’Halloran, K. L., Bailey, P., Brink, D.,
and Finn, J. (2018b). ‘she’s sort of breathing’: What
linguistic factors determine call-taker recognition of
agonal breathing in emergency calls for cardiac arrest?
Resuscitation, 122:92–98.
Riou, M., Ball, S., Williams, T. A., Whiteside, A.,
O’Halloran, K. L., Bray, J., Perkins, G. D., Smith,
K., Cameron, P., Fatovich, D. M., Inoue, M., Bailey,
P., Brink, D., and Finn, J. (2017). ‘tell me exactly
what’s happened’: When linguistic choices affect the
efficiency of emergency calls for cardiac arrest. Re-
suscitation, 117:58–65.
Sanfridsson, J., Sparrevik, J., Hollenberg, J., Nordberg,
P., Dj
¨
arv, T., Ringh, M., Svensson, L., Forsberg, S.,
Nord, A., Andersson-Hagiwara, M., and Claesson, A.
(2019). Drone delivery of an automated external de-
fibrillator - a mixed method simulation study of by-
stander experience. Scandinavian Journal of Trauma,
Resuscitation and Emergency Medicine, 27(1).
Sanko, S., Feng, S., Lane, C., and Eckstein, M. (2021).
Comparison of emergency medical dispatch systems
for performance of telecommunicator-assisted car-
diopulmonary resuscitation among 9-1-1 callers with
limited english proficiency. JAMA Network Open,
4(6).
Sanko, S., Kashani, S., Lane, C., and Eckstein, M.
(2020). Implementation of the los angeles tiered
dispatch system is associated with an increase
in telecommunicator-assisted cpr. Resuscitation,
155:74–81.
Scquizzato, T. (2018). Cardiac arrest detection through arti-
ficial intelligence-based surveillance camera: A work-
ing prototype. Resuscitation, 130:e114.
Sevil, H., Bastan, V., G
¨
ult
¨
urk, E., El Majzoub, I., and
G
¨
oksu, E. (2021). Effect of smartphone applications
on cardiopulmonary resuscitation quality metrics in a
mannequin study: A randomized trial. Turkish Jour-
nal of Emergency Medicine, 21(2):56–61.
Shirane, T. (2020). A systematic review of effectiveness of
automated external defibrillators delivered by drones.
Global Journal of Health Science, 12(12):101.
Smith, C. M., Griffiths, F., Fothergill, R. T., Vlaev, I.,
and Perkins, G. D. (2020). Identifying and over-
coming barriers to automated external defibrillator
use by goodsam volunteer first responders in out-of-
hospital cardiac arrest using the theoretical domains
framework and behaviour change wheel: A qualita-
tive study. BMJ Open, 10(3).
Sondergaard, K. B., Hansen, S. M., Pallisgaard, J. L., Gerds,
T. A., Wissenberg, M., Karlsson, L., Lippert, F. K.,
Gislason, G. H., Torp-Pedersen, C., and Folke, F.
(2018). Out-of-hospital cardiac arrest: Probability of
bystander defibrillation relative to distance to near-
est automated external defibrillator. Resuscitation,
124:138–144.
Srinivasan, S., Salerno, J., Hajari, H., Weiss, L. S., and Sal-
cido, D. D. (2017). Modeling a novel hypothetical use
of postal collection boxes as automated external defib-
rillator access points. Resuscitation, 120:26–30.
Stieglis, R., Zijlstra, J. A., Riedijk, F., Smeekes, M., van der
Worp, W. E., and Koster, R. W. (2020). Aed and text
message responders density in residential areas for
rapid response in out-of-hospital cardiac arrest. Re-
suscitation, 150:170–177.
Takahashi, H., Sagisaka, R., Natsume, Y., Tanaka, S.,
Takyu, H., and Tanaka, H. (2018). Does dispatcher-
assisted cpr generate the same outcomes as sponta-
neously delivered bystander cpr in japan? American
Journal of Emergency Medicine, 36(3):384–391.
Tanaka, S., Rodrigues, W., Sotir, S., Sagisaka, R., and
Tanaka, H. (2017). Cpr performance in the presence of
audiovisual feedback or football shoulder pads. BMJ
Open Sport and Exercise Medicine, 3(1).
Telec, W., Baszko, A., Da¸browski, M., Da¸browska, A., Sip,
M., Puslecki, M., Kłosiewicz, T., Potyrala, P., Jurczyk,
W., Maciejewski, A., Zalewski, R., Witt, M., Ladny,
J. R., and Szarpak, Ł. (2018). Automated external de-
fibrillator use in public places: A study of acquisition
time. Kardiologia Polska, 76(1):181–185.
Tsukigase, K., Takyu, H., Inoue, H., and Tanaka, H. (2019).
‘where and who” did deliver public access defibrilla-
tions for ohca in japan. Resuscitation, 142:e50–e51.
Valenzuela, T. D., Roe, D. J., Cretin, S., Spaite, D. W., and
Larsen, M. P. (1997). Estimating effectiveness of car-
diac arrest interventions. Circulation, 96(10):3308–
3313.
Van de Voorde, P., Gautama, S., Momont, A., Ionescu,
C. M., De Paepe, P., and Fraeyman, N. (2017). The
drone ambulance [A-UAS]: golden bullet or just a
blank? Resuscitation, 116:46–48.
Vaughan, N., John, N., and Rees, N. (2019). Cpr virtual
reality training simulator for schools. Proceedings -
2019 International Conference on Cyberworlds, CW
2019, pages 25–28.
White, A., Wah, W., Jalil, N., Lum, N., EKX, N., Kua,
P., and Ong, M. (2018). 11 Quality of bystander
CPR by lay first responders: training versus real-
world use of a novel CPR feedback device in singa-
pore. bmjopen.bmj.com.
White, A. E., Ng, H. X., Ng, W. Y., Ng, E. K. X., Fook-
Chong, S., Kua, P. H. J., and Ong, M. E. H. (2017).
Measuring the effectiveness of a novel cprcard
TM
feedback device during simulated chest compressions
by non-healthcare workers. Singapore Medical Jour-
nal, 58(7):438–445.
Zoll (2021). Real cpr help
R
: Quality technology to im-
prove cpr - zoll medical.
HEALTHINF 2023 - 16th International Conference on Health Informatics
488