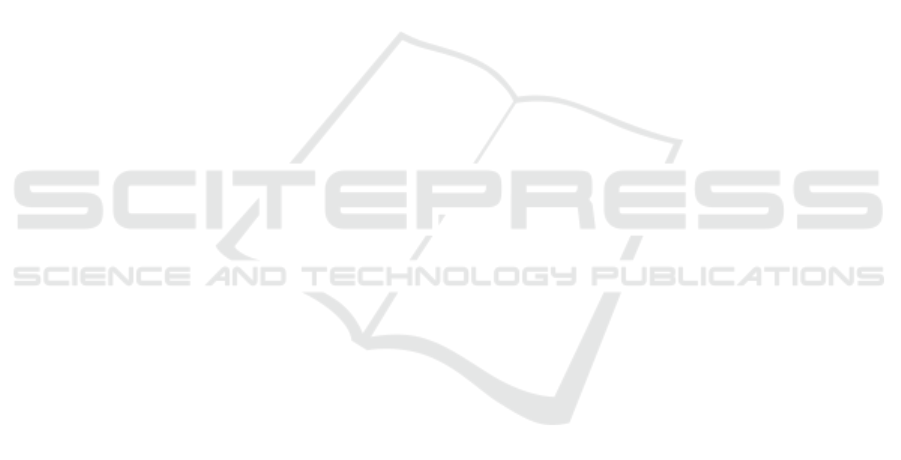
available for new researchers and can be downloaded
at https://github.com/lifeseniorproject/profile.
For future work, we plan to collect more data from
users and include more older people, which was not
included in this article due to the difficulty of recruit-
ing them. Increasing this dataset enables us to use
deep learning algorithms.
ACKNOWLEDGMENT
This study was financed in part by the Coordina-
tion for the Improvement of Higher Education Per-
sonnel - Brazil (CAPES) - Finance Code 001, Na-
tional Council for Scientific and Technological Devel-
opment (CNPq) and Financier of Studies and Projects
(FINEP).
REFERENCES
Aziz, O. and Robinovitch, S. N. (2011). An analy-
sis of the accuracy of wearable sensors for classify-
ing the causes of falls in humans. IEEE transac-
tions on neural systems and rehabilitation engineer-
ing, 19(6):670–676.
Blain, S., Mihailidis, A., and Chau, T. (2008). Assessing
the potential of electrodermal activity as an alterna-
tive access pathway. Medical Engineering & Physics,
30(4):498–505.
Bourke, A., O’Brien, J., and Lyons, G. (2007). Evaluation
of a threshold-based tri-axial accelerometer fall detec-
tion algorithm. Gait & Posture, 26(2):194–199.
Bourke, A. K. and Lyons, G. M. (2008). A threshold-based
fall-detection algorithm using a bi-axial gyroscope
sensor. Medical engineering & physics, 30(1):84–90.
Bruno, B., Mastrogiovanni, F., and Sgorbissa, A. (2014). A
public domain dataset for adl recognition using wrist-
placed accelerometers. In The 23rd IEEE Interna-
tional Symposium on Robot and Human Interactive
Communication, pages 738–743.
Casilari, E., Santoyo-Ram
´
on, J. A., and Cano-Garc
´
ıa, J. M.
(2017). Umafall: A multisensor dataset for the re-
search on automatic fall detection. Procedia Com-
puter Science, 110:32–39. 14th International Con-
ference on Mobile Systems and Pervasive Computing
(MobiSPC 2017) / 12th International Conference on
Future Networks and Communications (FNC 2017) /
Affiliated Workshops.
Garcia-Ceja, E., Thambawita, V., Hicks, S. A., Jha, D.,
Jakobsen, P., Hammer, H. L., Halvorsen, P., and
Riegler, M. A. (2021). Htad: A home-tasks activities
dataset with wrist-accelerometer and audio features.
In Loko
ˇ
c, J., Skopal, T., Schoeffmann, K., Mezaris,
V., Li, X., Vrochidis, S., and Patras, I., editors, Multi-
Media Modeling, pages 196–205, Cham. Springer In-
ternational Publishing.
Holtzclaw, B. J. (1993). Monitoring body temperature. Ad-
vances in cardiac care limiting care, 4(1):44–55.
Jarchi, D., Salvi, D., Tarassenko, L., and Clifton, D. A.
(2018). Validation of instantaneous respiratory rate
using reflectance ppg from different body positions.
Sensors.
Kamal, A., Harness, J., Irving, G., and Mearns, A. (1989).
Skin photoplethysmography — a review. Computer
Methods and Programs in Biomedicine, 28(4):257–
269.
Kurylyak, Y., Lamonaca, F., and Grimaldi, D. (2013). A
neural network-based method for continuous blood
pressure estimation from a ppg signal. 2013 IEEE In-
ternational Instrumentation and Measurement Tech-
nology Conference (I2MTC), pages 280–283.
Li, H., Shrestha, A., Heidari, H., Le Kernec, J., and Fio-
ranelli, F. (2019). Bi-lstm network for multimodal
continuous human activity recognition and fall detec-
tion. IEEE Sensors Journal, 20(3):1191–1201.
Mauldin, T. R., Canby, M. E., Metsis, V., Ngu, A. H., and
Rivera, C. C. (2018). Smartfall: A smartwatch-based
fall detection system using deep learning. Sensors,
18(10):3363.
McCarthy, C., Pradhan, N., Redpath, C., and Adler, A.
(2016). Validation of the empatica e4 wristband. In
2016 IEEE EMBS international student conference
(ISC), pages 1–4. IEEE.
Naschitz, J. E. and Rosner, I. (2007). Orthostatic hypoten-
sion: framework of the syndrome. Postgraduate med-
ical journal, 83(983):568–574.
Oliver, D. and Healy, F. (2009). Falls risk prediction tools
for hospital inpatients: do they work? Nursing Times,
105(7):18–21.
Piccinno, A., Hemmatpour, M., Ferrero, R., Montrucchio,
B., and Rebaudengo, M. (2019). A review on fall pre-
diction and prevention system for personal devices:
Evaluation and experimental results. Advances in
Human-Computer Interaction.
Saleh, M., Abbas, M., and Le Jeann
`
es, R. B. (2021a). Fal-
lalld: An open dataset of human falls and activities
of daily living for classical and deep learning applica-
tions. IEEE Sensors Journal, 21(2):1849–1858.
Saleh, M., Abbas, M., Prudhomm, J., Somme, D., and
Le Bouquin Jeann
`
es, R. (2021b). A reliable fall de-
tection system based on analyzing the physical activ-
ities of older adults living in long-term care facilities.
IEEE Transactions on Neural Systems and Rehabili-
tation Engineering, PP:1–1.
Santos, G. L., Endo, P. T., Monteiro, K. H. d. C., Rocha, E.
d. S., Silva, I., and Lynn, T. (2019). Accelerometer-
based human fall detection using convolutional neural
networks. Sensors, 19(7):1644.
Tamura, T., Maeda, Y., Sekine, M., and Yoshida, M. (2014).
Wearable photoplethysmographic sensors—past and
present. Electronics, 3(2):282–302.
Vassallo, M., Mallela, S. K., Williams, A., Kwan, J., Allen,
S., and Sharma, J. C. (2009). Fall risk factors in el-
derly patients with cognitive impairment on rehabili-
tation wards. Geriatrics & gerontology international,
9(1):41–46.
HEALTHINF 2023 - 16th International Conference on Health Informatics
460