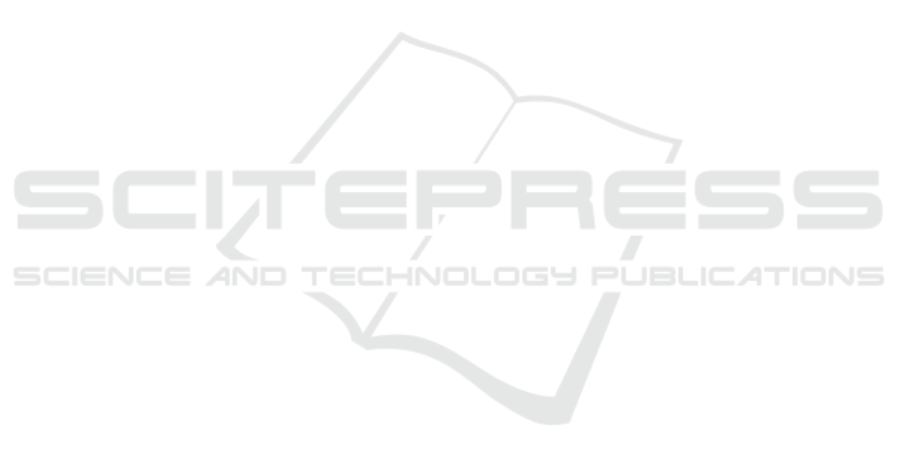
Cordts, M., Omran, M., Ramos, S., Rehfeld, T., Enzweiler,
M., Benenson, R., Franke, U., Roth, S., and Schiele,
B. (2016). The cityscapes dataset for semantic urban
scene understanding. In Proceedings of the IEEE con-
ference on computer vision and pattern recognition,
pages 3213–3223.
French, G., Laine, S., Aila, T., Mackiewicz, M., and Fin-
layson, G. (2019). Semi-supervised semantic seg-
mentation needs strong, varied perturbations. arXiv
preprint arXiv:1906.01916.
Goodfellow, I., Pouget-Abadie, J., Mirza, M., Xu, B.,
Warde-Farley, D., Ozair, S., Courville, A., and Ben-
gio, Y. (2020). Generative adversarial networks. Com-
munications of the ACM, 63(11):139–144.
Hadsell, R., Chopra, S., and LeCun, Y. (2006). Dimen-
sionality reduction by learning an invariant mapping.
In 2006 IEEE Computer Society Conference on Com-
puter Vision and Pattern Recognition (CVPR’06), vol-
ume 2, pages 1735–1742. IEEE.
Hung, W.-C., Tsai, Y.-H., Liou, Y.-T., Lin, Y.-Y., and
Yang, M.-H. (2018). Adversarial learning for semi-
supervised semantic segmentation. arXiv preprint
arXiv:1802.07934.
Lee, D.-H. et al. (2013). Pseudo-label: The simple and effi-
cient semi-supervised learning method for deep neural
networks. In Workshop on challenges in representa-
tion learning, ICML, volume 3, page 896.
Long, J., Shelhamer, E., and Darrell, T. (2015). Fully con-
volutional networks for semantic segmentation. In
Proceedings of the IEEE conference on computer vi-
sion and pattern recognition, pages 3431–3440.
Mittal, S., Tatarchenko, M., and Brox, T. (2019). Semi-
supervised semantic segmentation with high-and low-
level consistency. IEEE transactions on pattern anal-
ysis and machine intelligence, 43(4):1369–1379.
Olsson, V., Tranheden, W., Pinto, J., and Svensson, L.
(2021). Classmix: Segmentation-based data augmen-
tation for semi-supervised learning. In Proceedings of
the IEEE/CVF Winter Conference on Applications of
Computer Vision, pages 1369–1378.
Ouali, Y., Hudelot, C., and Tami, M. (2020). Semi-
supervised semantic segmentation with cross-
consistency training. In Proceedings of the
IEEE/CVF Conference on Computer Vision and
Pattern Recognition, pages 12674–12684.
Papandreou, G., Chen, L.-C., Murphy, K. P., and Yuille,
A. L. (2015). Weakly-and semi-supervised learning of
a deep convolutional network for semantic image seg-
mentation. In Proceedings of the IEEE international
conference on computer vision, pages 1742–1750.
Ronneberger, O., Fischer, P., and Brox, T. (2015). U-net:
Convolutional networks for biomedical image seg-
mentation. In International Conference on Medical
image computing and computer-assisted intervention,
pages 234–241. Springer.
Wang, W., Zhou, T., Yu, F., Dai, J., Konukoglu, E., and
Van Gool, L. (2021). Exploring cross-image pixel
contrast for semantic segmentation. In Proceedings of
the IEEE/CVF International Conference on Computer
Vision, pages 7303–7313.
Zou, Y., Zhang, Z., Zhang, H., Li, C.-L., Bian, X., Huang,
J.-B., and Pfister, T. (2020). Pseudoseg: Design-
ing pseudo labels for semantic segmentation. arXiv
preprint arXiv:2010.09713.
VISAPP 2023 - 18th International Conference on Computer Vision Theory and Applications
714