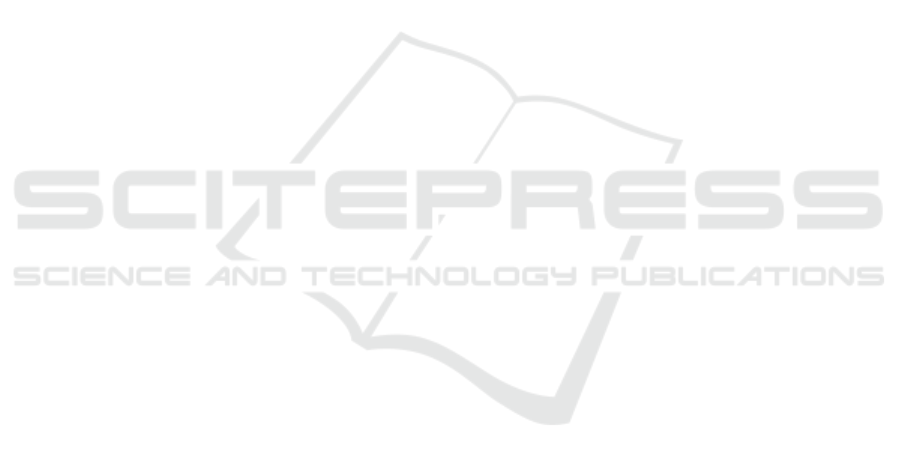
Golubovi
´
c, A. and Risojevi
´
c, V. (2021). Impact of data aug-
mentation on age estimation algorithms. In 2021 20th
International Symposium INFOTEH-JAHORINA (IN-
FOTEH), pages 1–6. IEEE.
Greco, A. (2021). Guess the age 2021: Age estima-
tion from facial images with deep convolutional neu-
ral networks. In Tsapatsoulis, N., Panayides, A.,
Theocharides, T., Lanitis, A., Pattichis, C., and Vento,
M., editors, Computer Analysis of Images and Pat-
terns, pages 265–274.
Grubl, T. and Lallie, H. S. (2022). Applying artificial in-
telligence for age estimation in digital forensic inves-
tigations. arXiv preprint arXiv:2201.03045.
Kang, B., Xie, S., Rohrbach, M., Yan, Z., Gordo, A., Feng,
J., and Kalantidis, Y. (2019). Decoupling representa-
tion and classifier for long-tailed recognition. arXiv
preprint arXiv:1910.09217.
Karras, T., Laine, S., and Aila, T. (2019). A style-based
generator architecture for generative adversarial net-
works. In Proceedings of the IEEE/CVF conference
on computer vision and pattern recognition, pages
4401–4410.
Karras, T., Laine, S., Aittala, M., Hellsten, J., Lehtinen,
J., and Aila, T. (2020). Analyzing and improving
the image quality of stylegan. In Proceedings of the
IEEE/CVF conference on computer vision and pattern
recognition, pages 8110–8119.
Kortli, Y., Jridi, M., Al Falou, A., and Atri, M. (2020). Face
recognition systems: A survey. Sensors, 20(2):342.
Krizhevsky, A., Sutskever, I., and Hinton, G. E. (2012). Im-
agenet classification with deep convolutional neural
networks. Advances in neural information processing
systems, 25.
Liu, X., Zou, Y., Kuang, H., and Ma, X. (2020). Face im-
age age estimation based on data augmentation and
lightweight convolutional neural network. Symmetry,
12(1):146.
Min, R., Hadid, A., and Dugelay, J.-L. (2011). Improv-
ing the recognition of faces occluded by facial acces-
sories. In 2011 IEEE International Conference on
Automatic Face & Gesture Recognition (FG), pages
442–447. IEEE.
Montillo, A. and Ling, H. (2009). Age regression from
faces using random forests. In 2009 16th IEEE In-
ternational Conference on Image Processing (ICIP),
pages 2465–2468. IEEE.
Osman, O. F. and Yap, M. H. (2018). Computational in-
telligence in automatic face age estimation: A survey.
IEEE Transactions on Emerging Topics in Computa-
tional Intelligence, 3(3):271–285.
Rothe, R., Timofte, R., and Van Gool, L. (2015). Dex: Deep
expectation of apparent age from a single image. In
Proceedings of the IEEE international conference on
computer vision workshops, pages 10–15.
Shen, W., Guo, Y., Wang, Y., Zhao, K., Wang, B., and
Yuille, A. (2019). Deep differentiable random forests
for age estimation. IEEE transactions on pattern anal-
ysis and machine intelligence, 43(2):404–419.
Shen, W., Guo, Y., Wang, Y., Zhao, K., Wang, B., and
Yuille, A. L. (2018). Deep regression forests for age
estimation. In Proceedings of the IEEE Conference
on Computer Vision and Pattern Recognition, pages
2304–2313.
Shin, N.-H., Lee, S.-H., and Kim, C.-S. (2022). Moving
window regression: A novel approach to ordinal re-
gression.
Simonyan, K. and Zisserman, A. (2014). Very deep con-
volutional networks for large-scale image recognition.
arXiv preprint arXiv:1409.1556.
Wang, H., Sanchez, V., and Li, C.-T. (2022). Improv-
ing face-based age estimation with attention-based dy-
namic patch fusion. IEEE Transactions on Image Pro-
cessing, 31:1084–1096.
Wang, Z., Tang, X., Luo, W., and Gao, S. (2018). Face ag-
ing with identity-preserved conditional generative ad-
versarial networks. In Proceedings of the IEEE con-
ference on computer vision and pattern recognition,
pages 7939–7947.
Yadav, D., Singh, R., Vatsa, M., and Noore, A. (2014). Rec-
ognizing age-separated face images: Humans and ma-
chines. PloS one, 9(12):e112234.
Yan, C., Meng, L., Li, L., Zhang, J., Wang, Z., Yin, J.,
Zhang, J., Sun, Y., and Zheng, B. (2022). Age-
invariant face recognition by multi-feature fusionand
decomposition with self-attention. ACM Transactions
on Multimedia Computing, Communications, and Ap-
plications (TOMM), 18(1s):1–18.
Yang, T.-Y., Huang, Y.-H., Lin, Y.-Y., Hsiu, P.-C., and
Chuang, Y.-Y. (2018). Ssr-net: A compact soft stage-
wise regression network for age estimation. In Pro-
ceedings of IJCAI-18, pages 1078–1084. IJCAI.
Yao, X., Puy, G., Newson, A., Gousseau, Y., and Hellier,
P. (2021). High resolution face age editing. In 2020
25th International Conference on Pattern Recognition
(ICPR), pages 8624–8631. IEEE.
Ye, L., Li, B., Mohammed, N., Wang, Y., and Liang, J.
(2018). Privacy-preserving age estimation for content
rating. In 2018 IEEE 20th International Workshop
on Multimedia Signal Processing (MMSP), pages 1–
6. IEEE.
Zhang, B. and Bao, Y. (2022). Cross-dataset learning for
age estimation. IEEE Access, 10:24048–24055.
Zhang, K., Zhang, Z., Li, Z., and Qiao, Y. (2016). Joint
face detection and alignment using multitask cascaded
convolutional networks. IEEE Signal Processing Let-
ters, 23(10):1499–1503.
Zhao, Z.-Q., Cheung, Y.-m., Hu, H., and Wu, X. (2016).
Corrupted and occluded face recognition via coop-
erative sparse representation. Pattern Recognition,
56:77–87.
Zhong, Z., Zheng, L., Kang, G., Li, S., and Yang, Y. (2020).
Random erasing data augmentation. In Proceedings
of the AAAI conference on artificial intelligence, vol-
ume 34, pages 13001–13008.
A Data Augmentation Strategy for Improving Age Estimation to Support CSEM Detection
699