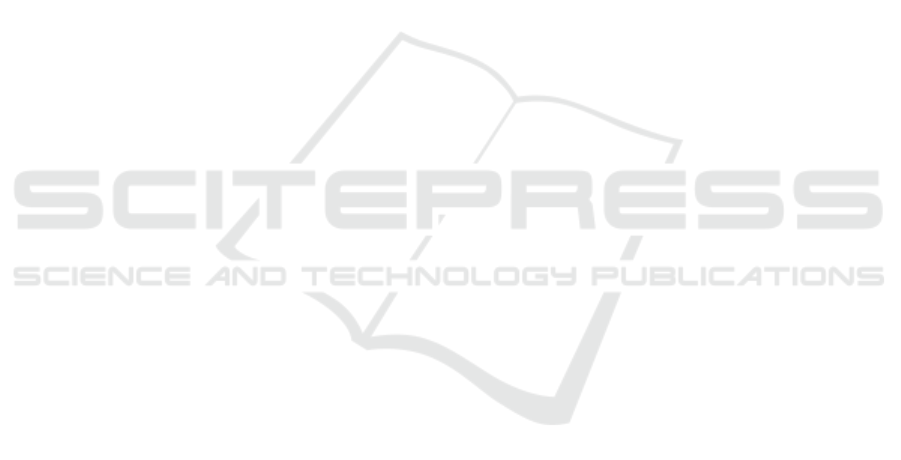
5 CONCLUSION AND FUTURE
DEVELOPMENTS
In this work, multilingual audio gender-based emo-
tion classification has been analyzed. Importantly, we
proposed a SER algorithm offering state-of-art results
while considering the full range of the big six emo-
tional states as expressed in six languages. Interest-
ingly, it has been demonstrated that a gender-based
emotion classifier can outperform a general emotion
classifier.
Future work could assess the performance reached
by such modeling architectures on each language sep-
arately. Moreover, these models could be part of a
more complex system to recognise human emotions
that use biosensors measuring physiological parame-
ters, e.g. heart rate, given the accelerated spread of
IoT devices as stated in the work of Pal (Pal et al.,
2021). Other additional work could investigate the
one-vs-all emotion classification scheme using the
present models; an example is the work of Saitta et
al. (Saitta and Ntalampiras, 2021). An alternative ap-
proach would be adding a language classifier before
emotion detection (with or without gender detection)
to access if it can achieve better results.
REFERENCES
Burkhardt, F., Paeschke, A., Rolfes, M., Sendlmeier, W. F.,
Weiss, B., et al. (2005). A database of german emo-
tional speech. In Interspeech, volume 5, pages 1517–
1520.
Cao, H., Cooper, D. G., Keutmann, M. K., Gur, R. C.,
Nenkova, A., and Verma, R. (2014). Crema-d: Crowd-
sourced emotional multimodal actors dataset. IEEE
transactions on affective computing, 5(4):377–390.
Chachadi, K. and Nirmala, S. R. (2021). Voice-based gen-
der recognition using neural network. In Informa-
tion and Communication Technology for Competitive
Strategies (ICTCS 2020), pages 741–749. Springer
Singapore.
Chen, L., Wang, K., Li, M., Wu, M., Pedrycz, W., and
Hirota, K. (2023). K-means clustering-based kernel
canonical correlation analysis for multimodal emotion
recognition in human–robot interaction. IEEE Trans-
actions on Industrial Electronics, 70(1):1016–1024.
Costantini, G., Iaderola, I., Paoloni, A., and Todisco,
M. (2014). Emovo corpus: an italian emotional
speech database. In International Conference on Lan-
guage Resources and Evaluation (LREC 2014), pages
3501–3504. European Language Resources Associa-
tion (ELRA).
Dair, Z., Donovan, R., and O’Reilly, R. (2021). Lin-
guistic and gender variation in speech emotion
recognition using spectral features. arXiv preprint
arXiv:2112.09596.
Giannakopoulos, T. and Pikrakis, A. (2014). Introduction
to Audio Analysis: A MATLAB Approach. Academic
Press, Inc., USA, 1st edition.
Han, K., Yu, D., and Tashev, I. (2014). Speech emotion
recognition using deep neural network and extreme
learning machine. In Interspeech 2014.
Hota, S. and Pathak, S. (2018). KNN classifier based ap-
proach for multi-class sentiment analysis of twitter
data. International Journal of Engineering and Tech-
nology, 7(3):1372.
James, J., Tian, L., and Watson, C. I. (2018). An open
source emotional speech corpus for human robot inter-
action applications. In INTERSPEECH, pages 2768–
2772.
Latif, S., Qayyum, A., Usman, M., and Qadir, J. (2018).
Cross lingual speech emotion recognition: Urdu vs.
western languages. In 2018 International Conference
on Frontiers of Information Technology (FIT), pages
88–93. IEEE.
Latif, S., Rana, R., Khalifa, S., Jurdak, R., and Schuller,
B. W. (2022). Self supervised adversarial do-
main adaptation for cross-corpus and cross-language
speech emotion recognition. IEEE Transactions on
Affective Computing, pages 1–1.
Livingstone, S. R. and Russo, F. A. (2018). The ryerson
audio-visual database of emotional speech and song
(ravdess): A dynamic, multimodal set of facial and
vocal expressions in north american english. PloS one,
13(5):e0196391.
Miller Jr, H. L. (2016). The Sage encyclopedia of theory in
psychology. SAGE Publications.
Mirsamadi, S., Barsoum, E., and Zhang, C. (2017). Auto-
matic speech emotion recognition using recurrent neu-
ral networks with local attention. In 2017 IEEE Inter-
national Conference on Acoustics, Speech and Signal
Processing (ICASSP), pages 2227–2231. IEEE.
Nezami, O. M., Lou, P. J., and Karami, M. (2019). Shemo: a
large-scale validated database for persian speech emo-
tion detection. Language Resources and Evaluation,
53(1):1–16.
Ntalampiras, S. (2020). Toward language-agnostic speech
emotion recognition. Journal of the Audio Engineer-
ing Society, 68(1/2):7–13.
Ntalampiras, S. (2021). Speech emotion recognition
via learning analogies. Pattern Recognition Letters,
144:21–26.
Pal, S., Mukhopadhyay, S., and Suryadevara, N. (2021).
Development and progress in sensors and tech-
nologies for human emotion recognition. Sensors,
21(16):5554.
Park, J.-S., Kim, J.-H., and Oh, Y.-H. (2009). Feature vec-
tor classification based speech emotion recognition for
service robots. IEEE Transactions on Consumer Elec-
tronics, 55(3):1590–1596.
Pavlovic, V., Sharma, R., and Huang, T. (1997). Visual
interpretation of hand gestures for human-computer
interaction: a review. IEEE Transactions on Pattern
Analysis and Machine Intelligence, 19(7):677–695.
Pichora-Fuller, M. K. and Dupuis, K. (2020). Toronto emo-
tional speech set (TESS). Scholars Portal Dataverse.
ICPRAM 2023 - 12th International Conference on Pattern Recognition Applications and Methods
684