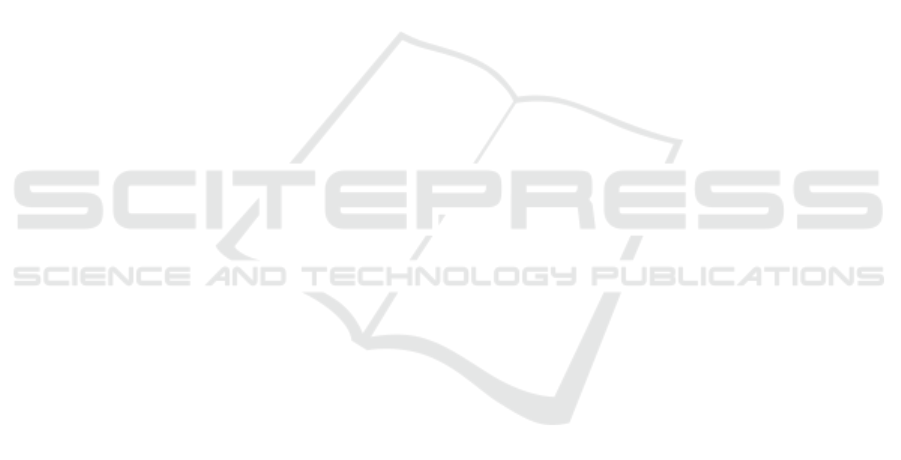
by (Savchenko et al., 2022) fetched the best accuracy
of 78.75%. All methods used a softmax layer or a
linear transformation as the classifier. The models
were trained for at least 50 epochs. The respective
accuracies are reported in table 3 (Deep FER section)
with 10 fold cross validation. These results further
substantiate the usability of our dataset in real-world
practical applications.
5 CONCLUSION & FUTURE
WORK
India being a culturally and ethnically rich coun-
try, a home to about 1.4 billion people with various
racial identities migrating and settling in the subcon-
tinent. In this context, there existed a need for an
India-specific ethnically diverse dataset comprising
all seven basic human facial expressions.
The proposed InFER dataset comprises of 10,200
images & 4,200 videos of seven basic facial expres-
sions with their age, gender, and ethnic labels. The
subject selection done in this regard corroborated that
there should not be any dataset bias with respect to
ethnicity, age, class, or gender. Moreover, since posed
human expressions lack in realistic data, we adopted a
two way collection strategy. Whilst posed expressions
from human subjects were captured; on the contrary,
we also collected realistic spontaneous/acted expres-
sions collected on a crowd-sourced basis from on-
line sources. We also conducted extensive experi-
mentation on baseline models and available state-of-
the-art deep-learning-based models, showing that our
propsed dataset can be deployed for real-world prac-
tical applications. The Multi-Ethnic Indian Facial Ex-
pression Recognition (InFER) dataset would facilitate
researchers to train and validate their algorithms for
real-world practical applications.
REFERENCES
Cai, J., Meng, Z., Khan, A. S., Li, Z., O’Reilly, J., and Tong,
Y. (2018). Island loss for learning discriminative fea-
tures in facial expression recognition. In 2018 13th
IEEE International Conference on Automatic Face &
Gesture Recognition (FG 2018), pages 302–309.
Darwin, C. and Prodger, P. (1998). The expression of the
emotions in man and animals. Oxford University
Press, USA.
Deng, H.-B., Jin, L.-W., Zhen, L.-X., Huang, J.-C.,
et al. (2005). A new facial expression recognition
method based on local gabor filter bank and pca plus
lda. International Journal of Information Technology,
11(11):86–96.
Ekman, P. (1994). Strong evidence for universals in facial
expressions: A reply to russell’s mistaken critique.
Psychological Bulletin, 115:268–287.
Ekman, P. and Friesen, W. V. (1971). Constants across cul-
tures in the face and emotion. Journal of personality
and social psychology, 17(2):124.
Khorrami, P., Paine, T., and Huang, T. (2015). Do deep neu-
ral networks learn facial action units when doing ex-
pression recognition? In Proceedings of the IEEE in-
ternational conference on computer vision workshops,
pages 19–27.
Li, S. and Deng, W. (2020). Deep facial expression recog-
nition: A survey. IEEE transactions on affective com-
puting.
Mehrabian, A. and Russell, J. A. (1974). An approach to
environmental psychology. the MIT Press.
Moore, S. and Bowden, R. (2011). Local binary patterns for
multi-view facial expression recognition. Computer
vision and image understanding, 115(4):541–558.
Ojala, T., Pietikainen, M., and Maenpaa, T. (2002). Mul-
tiresolution gray-scale and rotation invariant texture
classification with local binary patterns. IEEE Trans-
actions on pattern analysis and machine intelligence,
24(7):971–987.
Pramerdorfer, C. and Kampel, M. (2016). Facial expression
recognition using convolutional neural networks: state
of the art. arXiv preprint arXiv:1612.02903.
Risley, H. H. (1891). The study of ethnology in india.
The Journal of the Anthropological Institute of Great
Britain and Ireland, 20:235–263.
Savchenko, A. V., Savchenko, L. V., and Makarov, I.
(2022). Classifying emotions and engagement in on-
line learning based on a single facial expression recog-
nition neural network. IEEE Transactions on Affective
Computing, pages 1–12.
Senechal, T., Rapp, V., Salam, H., Seguier, R., Bailly, K.,
and Prevost, L. (2011). Combining aam coefficients
with lgbp histograms in the multi-kernel svm frame-
work to detect facial action units. In 2011 IEEE In-
ternational Conference on Automatic Face & Gesture
Recognition (FG), pages 860–865. IEEE.
Tian, Y.-I., Kanade, T., and Cohn, J. F. (2001). Recogniz-
ing action units for facial expression analysis. IEEE
Transactions on pattern analysis and machine intelli-
gence, 23(2):97–115.
Viola, P. and Jones, M. (2001). Rapid object detection us-
ing a boosted cascade of simple features. In Proceed-
ings of the 2001 IEEE computer society conference on
computer vision and pattern recognition. CVPR 2001,
volume 1, pages I–I. Ieee.
Wang, S., Liu, Z., Lv, S., Lv, Y., Wu, G., Peng, P., Chen, F.,
and Wang, X. (2010). A natural visible and infrared
facial expression database for expression recognition
and emotion inference. IEEE Transactions on Multi-
media, 12(7):682–691.
Wen, Y., Zhang, K., Li, Z., and Qiao, Y. (2016). A discrim-
inative feature learning approach for deep face recog-
nition. In European conference on computer vision,
pages 499–515. Springer.
InFER: A Multi-Ethnic Indian Facial Expression Recognition Dataset
557