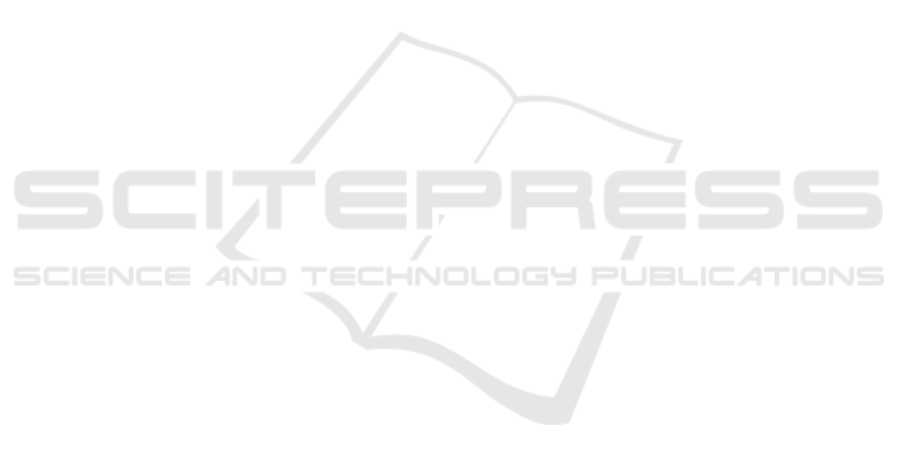
Beniwal, P., Mantini, P., and Shah, S. K. (2022). Image
quality assessment using deep features for object de-
tection. In VISIGRAPP (4: VISAPP), pages 706–714.
Best-Rowden, L. and Jain, A. K. (2017). Automatic
face image quality prediction. arXiv preprint
arXiv:1706.09887.
Chang, J., Lan, Z., Cheng, C., and Wei, Y. (2020). Data un-
certainty learning in face recognition. In Proceedings
of the IEEE/CVF Conference on Computer Vision and
Pattern Recognition, pages 5710–5719.
Chen, K., Lv, Q., Yi, T., and Yi, Z. (2021a). Reliable
probabilistic face embeddings in the wild. ArXiv,
abs/2102.04075.
Chen, K., Yi, T., and Lv, Q. (2021b). Lightqnet:
Lightweight deep face quality assessment for risk-
controlled face recognition. IEEE Signal Processing
Letters, 28:1878–1882.
Grother, P. and Tabassi, E. (2007). Performance of biomet-
ric quality measures. IEEE Transactions on Pattern
Analysis and Machine Intelligence, 29(4):531–543.
He, K., Zhang, X., Ren, S., and Sun, J. (2016). Deep resid-
ual learning for image recognition. In Proceedings of
the IEEE conference on computer vision and pattern
recognition, pages 770–778.
Hernandez-Ortega, J., Galbally, J., Fierrez, J., Haraksim, R.,
and Beslay, L. (2019). Faceqnet: Quality assessment
for face recognition based on deep learning. In 2019
International Conference on Biometrics (ICB), pages
1–8. IEEE.
Kang, L., Ye, P., Li, Y., and Doermann, D. (2014). Convolu-
tional neural networks for no-reference image quality
assessment. In Proceedings of the IEEE conference on
computer vision and pattern recognition, pages 1733–
1740.
Kong, L., Ikusan, A., Dai, R., and Zhu, J. (2019). Blind im-
age quality prediction for object detection. In 2019
IEEE Conference on Multimedia Information Pro-
cessing and Retrieval (MIPR), pages 216–221. IEEE.
Lin, T.-Y., Maire, M., Belongie, S., Hays, J., Perona, P.,
Ramanan, D., Doll
´
ar, P., and Zitnick, C. L. (2014).
Microsoft : Common objects in context. In Euro-
pean Conference on Computer Vision, pages 740–755.
Springer.
Meng, Q., Zhao, S., Huang, Z., and Zhou, F. (2021).
Magface: A universal representation for face recog-
nition and quality assessment. In Proceedings of the
IEEE/CVF Conference on Computer Vision and Pat-
tern Recognition, pages 14225–14234.
Ou, F.-Z., Chen, X., Zhang, R., Huang, Y., Li, S., Li, J., Li,
Y., Cao, L., and Wang, Y.-G. (2021). Sdd-fiqa: unsu-
pervised face image quality assessment with similarity
distribution distance. In Proceedings of the IEEE/CVF
Conference on Computer Vision and Pattern Recogni-
tion, pages 7670–7679.
Pan, D., Shi, P., Hou, M., Ying, Z., Fu, S., and Zhang, Y.
(2018). Blind predicting similar quality map for image
quality assessment. In Proceedings of the IEEE con-
ference on computer vision and pattern recognition,
pages 6373–6382.
Ren, S., He, K., Girshick, R., and Sun, J. (2015). Faster
r-cnn: Towards real-time object detection with region
proposal networks. In Advances in Neural Informa-
tion Processing Systems, pages 91–99.
Schlett, T., Rathgeb, C., Henniger, O., Galbally, J., Fierrez,
J., and Busch, C. (2020). Face image quality assess-
ment: A literature survey. ACM Computing Surveys
(CSUR).
Schroff, F., Kalenichenko, D., and Philbin, J. (2015).
Facenet: A unified embedding for face recognition
and clustering. In Proceedings of the IEEE conference
on computer vision and pattern recognition, pages
815–823.
Shi, Y. and Jain, A. K. (2019). Probabilistic face embed-
dings. In Proceedings of the IEEE/CVF International
Conference on Computer Vision, pages 6902–6911.
Terhorst, P., Kolf, J. N., Damer, N., Kirchbuchner, F., and
Kuijper, A. (2020). Ser-fiq: Unsupervised estimation
of face image quality based on stochastic embedding
robustness. In Proceedings of the IEEE/CVF con-
ference on computer vision and pattern recognition,
pages 5651–5660.
Wallace, G. K. (1992). The jpeg still picture compression
standard. IEEE Transactions on Consumer Electron-
ics, 38(1):xviii–xxxiv.
Wu, Y., Chen, Y., Yuan, L., Liu, Z., Wang, L., Li, H.,
and Fu, Y. (2020). Rethinking classification and lo-
calization for object detection. In Proceedings of the
IEEE/CVF Conference on Computer Vision and Pat-
tern Recognition, pages 10186–10195.
Zhai, G. and Min, X. (2020). Perceptual image quality as-
sessment: a survey. Science China Information Sci-
ences, 63(11):1–52.
VISAPP 2023 - 18th International Conference on Computer Vision Theory and Applications
354