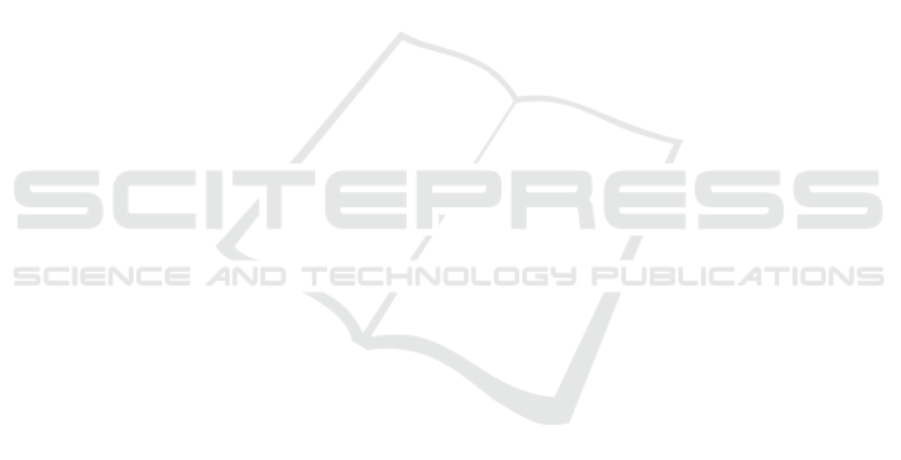
structing the bottom third of a collapsed face before
designing dentures.
When looking for a similar non-collapsed face
which can be used as a reference to the query im-
age that contains a collapsed face, it would be ideal
to have a reference face that is a closest match to the
query image as this would help in more accurate re-
construction procedure of the query image that con-
tains a collapsed face. Since this proposed method is
about finding similar images from the target dataset,
the bigger and diverse the dataset is, the more is the
probability of finding a closer and a similar match to
the query image. However, it should be made sure
that the target dataset does not contain images of peo-
ple with a collapsed face. In addition to age and gen-
der, there can be other factors like race, ethnicity, skin
color, height, weight, etc. that can affect the similar-
ity score and help to find the closest match. It is worth
exploring the similar images in a more wide and di-
verse dataset to check for the similarity scores and
similar images. Also, we would like to collect images
of patients that have collapsed faces to evaluate the
proposed method.
REFERENCES
Alasadi, J., Al Hilli, A., and Singh, V. K. (2019). To-
ward fairness in face matching algorithms. In Pro-
ceedings of the 1st International Workshop on Fair-
ness, Accountability, and Transparency in MultiMe-
dia, FAT/MM ’19, page 19–25, New York, NY, USA.
Association for Computing Machinery.
Bicego, M., Grosso, E., and Tistarelli, M. (2005). On find-
ing differences between faces. In International Con-
ference on Audio-and Video-Based Biometric Person
Authentication, pages 329–338. Springer.
Geitgey, A. (2018). Face recognition library.
kaggle (2020). Human faces dataset.
Karras, T., Laine, S., Aittala, M., Hellsten, J., Lehtinen,
J., and Aila, T. (2020). Analyzing and improving
the image quality of stylegan. In Proceedings of the
IEEE/CVF conference on computer vision and pattern
recognition, pages 8110–8119.
King, D. E. (2009). Dlib-ml: A machine learning toolkit.
Journal of Machine Learning Research, 10:1755–
1758.
Kramer, R. S. and Reynolds, M. G. (2018). Unfamiliar face
matching with frontal and profile views. Perception,
47(4):414–431.
Lamba, H., Sarkar, A., Vatsa, M., Singh, R., and Noore, A.
(2011). Face recognition for look-alikes: A prelimi-
nary study. In 2011 International Joint Conference on
Biometrics (IJCB), pages 1–6.
McCauley, J., Soleymani, S., Williams, B., Dando, J.,
Nasrabadi, N., and Dawson, J. (2021a). Identical
twins as a facial similarity benchmark for human fa-
cial recognition. In 2021 International Conference
of the Biometrics Special Interest Group (BIOSIG),
pages 1–5.
McCauley, J., Soleymani, S., Williams, B., Dando, J.,
Nasrabadi, N., and Dawson, J. (2021b). Identical
twins as a facial similarity benchmark for human fa-
cial recognition. In 2021 International Conference
of the Biometrics Special Interest Group (BIOSIG),
pages 1–5.
Oron, S., Dekel, T., Xue, T., Freeman, W. T., and Avi-
dan, S. (2018). Best-buddies similarity—robust tem-
plate matching using mutual nearest neighbors. IEEE
Transactions on Pattern Analysis and Machine Intel-
ligence, 40(8):1799–1813.
O’Toole, A. J., Jonathon Phillips, P., Jiang, F., Ayyad, J.,
Penard, N., and Abdi, H. (2007). Face recognition al-
gorithms surpass humans matching faces over changes
in illumination. IEEE Transactions on Pattern Analy-
sis and Machine Intelligence, 29(9):1642–1646.
Ramanathan, N., Chellappa, R., and Roy Chowdhury, A.
(2004). Facial similarity across age, disguise, illumi-
nation and pose. In 2004 International Conference on
Image Processing, 2004. ICIP ’04., volume 3, pages
1999–2002 Vol. 3.
R
¨
ottcher, A., Scherhag, U., and Busch, C. (2020). Finding
the suitable doppelg
¨
anger for a face morphing attack.
In 2020 IEEE international joint conference on bio-
metrics (IJCB), pages 1–7. IEEE.
Ruys, K. I., Spears, R., Gordijn, E. H., and de Vries, N. K.
(2006). Two faces of (dis) similarity in affective judg-
ments of persons: Contrast or assimilation effects re-
vealed by morphs. Journal of Personality and Social
Psychology, 90(3):399.
Sadovnik, A., Gharbi, W., Vu, T., and Gallagher, A. (2018).
Finding your lookalike: Measuring face similarity
rather than face identity. In Proceedings of the IEEE
Conference on Computer Vision and Pattern Recogni-
tion (CVPR) Workshops.
Santini, S. and Jain, R. (1996). Similarity matching. In
Asian conference on computer vision, pages 571–580.
Springer.
Schroff, F., Treibitz, T., Kriegman, D., and Belongie, S.
(2011). Pose, illumination and expression invariant
pairwise face-similarity measure via doppelg
¨
anger list
comparison. In 2011 International Conference on
Computer Vision, pages 2494–2501.
Torun, R. B., Yurdakul, M., and Duygulu, P. (2009). Find-
ing similar faces. In 2009 IEEE 17th Signal Process-
ing and Communications Applications Conference,
pages 960–963.
VISAPP 2023 - 18th International Conference on Computer Vision Theory and Applications
904