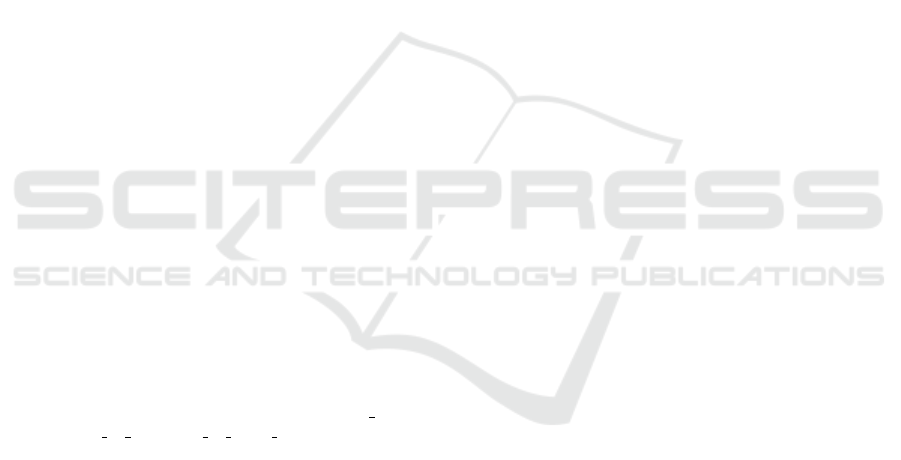
blood pressure, chronic kidney disease, fever, cough,
shortness of breath, fatigue, lung disease, among oth-
ers.
ACKNOWLEDGEMENTS
This study was financed in part by the Coordenac¸
˜
ao
de Aperfeic¸oamento de Pessoal de N
´
ıvel Superior –
Brasil (CAPES) – Finance Code 001. The authors
thank the National Council for Scientific and Techno-
logical Development of Brazil (CNPq - Conselho Na-
cional de Desenvolvimento Cient
´
ıfico e Tecnol
´
ogico)
and the Foundation for Research Support of the Minas
Gerais State (FAPEMIG). The work was developed
at the Pontifical Catholic University of Minas Gerais,
PUC Minas.
REFERENCES
Albuquerque, C. (2021). Pandemia diminui n
´
umero e muda
perfil de internac¸
˜
oes no sus em 2020. Dispon
´
ıvel
em: https://observatoriohospitalar.fiocruz.br/debates-
e-opinioes/pandemia-diminui-numero-e-muda-perfil-
de-internacoes-no-sus-em-2020. Acesso em:01 de
julho de 2022.
Armstrong, R., Kane, A., and Cook, T. (2020). Out-
comes from intensive care in patients with covid-19: a
systematic review and meta-analysis of observational
studies. Anaesthesia, 75(10):1340–1349.
Ciaburro, G., Ayyadevara, V. K., and Perrier, A. (2018).
Hands-on machine learning on google cloud plat-
form: Implementing smart and efficient analytics us-
ing cloud ml engine. Packt Publishing Ltd.
da S
´
aude do Brasil, M. (2021). Guia de vigil
ˆ
ancia
epidemiol
´
ogica para covid-19. Dispon
´
ıvel em:
https://coronavirus.saude.mg.gov.br/images/1
2021/17-
03-Guia de vigilancia da covid 16marc2021.pdf.
Acesso em:01 de julho de 2022.
Feitoza, T. M. O., Chaves, A. M., Muniz, G. T. S., da Cruz,
M. C. C., and Junior, I. d. F. C. (2020). Comorbidades
e covid-19. Revista Interfaces: Sa
´
ude, Humanas e
Tecnologia, 8(3):711–723.
Gao, J., Yang, C., Heintz, J., Barrows, S., Albers, E.,
Stapel, M., Warfield, S., Cross, A., Sun, J., et al.
(2022). Medml: Fusing medical knowledge and
machine learning models for early pediatric covid-
19 hospitalization and severity prediction. Iscience,
25(9):104970.
Ilyas, I. F. and Rekatsinas, T. (2022). Machine learning and
data cleaning: Which serves the other? ACM Journal
of Data and Information Quality (JDIQ), 14(3):1–11.
Jehi, L., Ji, X., Milinovich, A., Erzurum, S., Merlino, A.,
Gordon, S., Young, J. B., and Kattan, M. W. (2020).
Development and validation of a model for individual-
ized prediction of hospitalization risk in 4,536 patients
with covid-19. PloS one, 15(8):e0237419.
Lana, R. M., Freitas, L. P., Codec¸o, C. T., Pacheco, A. G.,
Carvalho, L. M. F. d., Villela, D. A. M., Coelho, F. C.,
Cruz, O. G., Niquini, R. P., Porto, V. B. G., et al.
(2021). Identificac¸
˜
ao de grupos priorit
´
arios para a
vacinac¸
˜
ao contra covid-19 no brasil. Cadernos de
Sa
´
ude P
´
ublica, 37.
Maciel, J. A. C., Castro-Silva, I. I., and Farias, M. R. d.
(2020). An
´
alise inicial da correlac¸
˜
ao espacial entre a
incid
ˆ
encia de covid-19 e o desenvolvimento humano
nos munic
´
ıpios do estado do cear
´
a no brasil. Revista
brasileira de epidemiologia, 23:e200057.
Murtas, R., Morici, N., Cogliati, C., Puoti, M., Omazzi, B.,
Bergamaschi, W., Voza, A., Querini, P. R., Stefanini,
G., Manfredi, M. G., et al. (2021). Algorithm for indi-
vidual prediction of covid-19–related hospitalization
based on symptoms: Development and implementa-
tion study. JMIR Public Health and Surveillance,
7(11):e29504.
Organization, W. H. et al. (2020). Oxygen sources and dis-
tribution for covid-19 treatment centres: interim guid-
ance, 4 april 2020. Technical report, World Health
Organization.
Paim, J., Travassos, C., Almeida, C., Bahia, L., and
Macinko, J. (2011). The brazilian health system:
history, advances, and challenges. The Lancet,
377(9779):1778–1797.
Portela, M. C., de Aguiar Pereira, C. C., Lima, S. M. L.,
de Andrade, C. L. T., and Martins, M. (2021). Patterns
of hospital utilization in the unified health system in
six brazilian capitals: comparison between the year
before and the first six first months of the covid-19
pandemic. BMC Health Services Research, 21(1):1–
13.
Ranzani, O. T., Bastos, L. S., Gelli, J. G. M., Marchesi,
J. F., Bai
˜
ao, F., Hamacher, S., and Bozza, F. A. (2021).
Characterisation of the first 250 000 hospital admis-
sions for covid-19 in brazil: a retrospective analysis
of nationwide data. The Lancet Respiratory Medicine,
9(4):407–418.
Rothan, H. A. and Byrareddy, S. N. (2020). The epidemi-
ology and pathogenesis of coronavirus disease (covid-
19) outbreak. Journal of autoimmunity, 109:102433.
Santos, L. G., da Silva, R. V., Leal, T. C., Xavier, J. E.,
de Souza Figueiredo, E. V. M., de Paiva, J. P. S.,
da Silva, L. F., de Oliveira Rocha, C. A., Nunes, B. E.
B. R., de Almeida Santana, G. B., et al. (2022a). Im-
pact of the covid-19 pandemic on hospital admissions
and in-hospital lethality from cardiovascular diseases
in brazil: an ecological and time series study covid-
19 and cardiovascular diseases. Current Problems in
Cardiology, page 101216.
Santos, P. P. G. V. d., Oliveira, R. A. D. d., and Albuquerque,
M. V. d. (2022b). Desigualdades da oferta hospitalar
no contexto da pandemia da covid-19 no brasil: uma
revis
˜
ao integrativa. Sa
´
ude em Debate, 46:322–337.
Sciannameo, V., Goffi, A., Maffeis, G., Gianfreda, R.,
Pagliari, D. J., Filippini, T., Mancuso, P., Giorgi-
Rossi, P., Dal Zovo, L. A., Corbari, A., et al. (2022).
A deep learning approach for spatio-temporal fore-
casting of new cases and new hospital admissions of
covid-19 spread in reggio emilia, northern italy. Jour-
nal of Biomedical Informatics, 132:104132.
The Use of Machine Learning to Predict Hospitalization of Covid-19: A Case Study in the State of Minas Gerais - Brazil
399