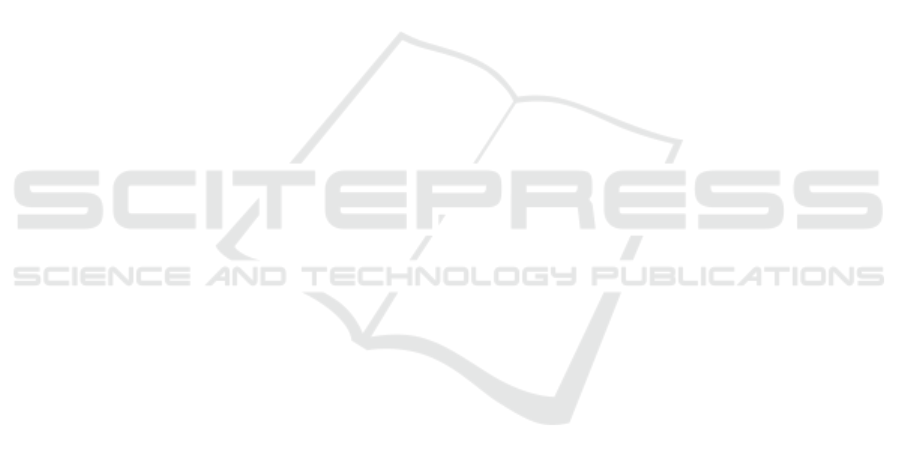
shape bias improves accuracy and robustness. arXiv
preprint arXiv:1811.12231.
Greenberg, S., Aladjem, M., and Kogan, D. (2002). Finger-
print image enhancement using filtering techniques.
Real-Time Imaging, 8(3):227–236.
Hong, L., Wan, Y., and Jain, A. (1998). Fingerprint image
enhancement: Algorithm and performance evaluation.
IEEE transactions on pattern analysis and machine
intelligence, 20(8):777–789.
Hsieh, C.-T., Lai, E., and Wang, Y.-C. (2003). An effective
algorithm for fingerprint image enhancement based on
wavelet transform. Pattern Recognition, 36(2):303–
312.
Ismael, S. H., Mustafa, F. M., and Ok
¨
um
¨
us, I. T. (2016).
A new approach of image denoising based on dis-
crete wavelet transform. In 2016 World Symposium on
Computer Applications & Research (WSCAR), pages
36–40. IEEE.
Jain, A. K., Ross, A., and Prabhakar, S. (2004). An intro-
duction to biometric recognition. IEEE Transactions
on circuits and systems for video technology, 14(1):4–
20.
Joshi, I., Anand, A., Vatsa, M., Singh, R., Roy, S. D., and
Kalra, P. (2019). Latent fingerprint enhancement us-
ing generative adversarial networks. In 2019 IEEE
winter conference on applications of computer vision
(WACV), pages 895–903. IEEE.
Kanagaraj, H. and Muneeswaran, V. (2020). Image com-
pression using haar discrete wavelet transform. In
2020 5th International Conference on Devices, Cir-
cuits and Systems (ICDCS). IEEE.
Kim, B.-G., Kim, H.-J., and Park, D.-J. (2002). New en-
hancement algorithm for fingerprint images. In 2002
International Conference on Pattern Recognition, vol-
ume 3, pages 879–882. IEEE.
Kimlyk, M. and Umnyashkin, S. (2018). Image denois-
ing using discrete wavelet transform and edge infor-
mation. In 2018 IEEE Conference of Russian Young
Researchers in Electrical and Electronic Engineering
(EIConRus). IEEE.
Ko, K. (2007). User’s guide to nist biometric image soft-
ware (nbis).
Li, J., Feng, J., and Kuo, C.-C. J. (2018). Deep convolu-
tional neural network for latent fingerprint enhance-
ment. Signal Processing: Image Communication.
Li, Q., Shen, L., Guo, S., and Lai, Z. (2021). Wavecnet:
Wavelet integrated cnns to suppress aliasing effect for
noise-robust image classification. IEEE Transactions
on Image Processing, 30:7074–7089.
Liu, M., Chen, X., and Wang, X. (2014). Latent fingerprint
enhancement via multi-scale patch based sparse repre-
sentation. IEEE Transactions on Information Foren-
sics and Security, 10(1):6–15.
Liu, M. and Qian, P. (2020). Automatic segmentation and
enhancement of latent fingerprints using deep nested
unets. IEEE Transactions on Information Forensics
and Security, 16:1709–1719.
Liu, P., Zhang, H., Lian, W., and Zuo, W. (2019). Multi-
level wavelet convolutional neural networks. IEEE
Access, 7:74973–74985.
Maio, D., Maltoni, D., Cappelli, R., Wayman, J. L., and
Jain, A. K. (2002). Fvc2002: Second fingerprint ver-
ification competition. In 2002 International Confer-
ence on Pattern Recognition, volume 3. IEEE.
Qian, P., Li, A., and Liu, M. (2019). Latent fingerprint en-
hancement based on denseunet. In 2019 International
Conference on Biometrics (ICB), pages 1–6. IEEE.
Rahman, S. M., Ahmad, M. O., and Swamy, M. (2008).
Improved image restoration using wavelet-based de-
noising and fourier-based deconvolution. In 2008 51st
Midwest Symposium on Circuits and Systems, pages
249–252. IEEE.
Ronneberger, O., Fischer, P., and Brox, T. (2015). U-net:
Convolutional networks for biomedical image seg-
mentation. In International Conference on Medical
image computing and computer-assisted intervention,
pages 234–241. Springer.
Sherlock, B., Monro, D., and Millard, K. (1992). Algorithm
for enhancing fingerprint images. Electronics letters,
18(28):1720–1721.
Szu, H. H., Telfer, B. A., and Kadambe, S. L. (1992). Neu-
ral network adaptive wavelets for signal representation
and classification. Optical Engineering, 31(9):1907–
1916.
Tabassi, E., Olsen, M., Bausinger, O., Busch, C., Figlarz,
A., Fiumara, G., Henniger, O., Merkle, J., Ruhland,
T., Schiel, C., and Schwaiger, M. (2021). Nist finger-
print image quality 2.
V, S. A. and Sivaswamy, J. (2018). Fpd-m-net: Fin-
gerprint image denoising and inpainting using m-
net based convolutional neural networks. CoRR,
abs/1812.10191.
Winkler, S. and Susstrunk, S. (2004). Visibility of noise
in natural images. In Human Vision and Electronic
Imaging IX, volume 5292, pages 121–129. SPIE.
Xie, C., Wu, Y., Maaten, L. v. d., Yuille, A. L., and He, K.
(2019). Feature denoising for improving adversarial
robustness. In Proceedings of the IEEE/CVF confer-
ence on computer vision and pattern recognition.
Yang, J., Liu, L., and Jiang, T. (2002). Improved method
for extraction of fingerprint features. In Second Inter-
national Conference on Image and Graphics, volume
4875, pages 552–558. SPIE.
Zhang, L., Zhang, L., Mou, X., and Zhang, D. (2012).
A comprehensive evaluation of full reference image
quality assessment algorithms. In 2012 19th IEEE In-
ternational Conference on Image Processing. IEEE.
Zhang, Q. and Benveniste, A. (1992). Wavelet networks.
IEEE transactions on Neural Networks, 3(6).
Zhang, W.-P., Wang, Q.-R., and Tang, Y. Y. (2002). A
wavelet-based method for fingerprint image enhance-
ment. In Proceedings. International Conference on
Machine Learning and Cybernetics, volume 4. IEEE.
Zhao, H., Gallo, O., Frosio, I., and Kautz, J. (2016).
Loss functions for image restoration with neural net-
works. IEEE Transactions on computational imaging,
3(1):47–57.
Zhao, X., Huang, P., and Shu, X. (2022). Wavelet-attention
cnn for image classification. Multimedia Systems,
28(3):915–924.
VISAPP 2023 - 18th International Conference on Computer Vision Theory and Applications
316