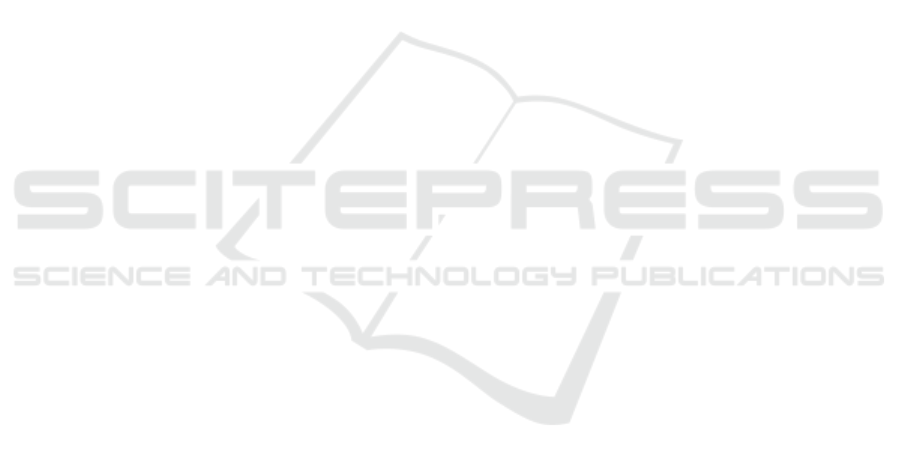
Question Answering. In CVPR, pages 6904–6913.
Hu, R., Andreas, J., Darrell, T., and Saenko, K. (2018). Ex-
plainable neural computation via stack neural module
networks. In ECCV, pages 53–69.
Hudson, D. A. and Manning, C. D. (2018). Compositional
attention networks for machine reasoning. In ICLR.
Hudson, D. A. and Manning, C. D. (2019). GQA: A new
dataset for real-world visual reasoning and compo-
sitional question answering. In CVPR, pages 6693–
6702.
Johnson, J., Hariharan, B., van der Maaten, L., Fei-Fei,
L., Lawrence Zitnick, C., and Girshick, R. (2017a).
CLEVR: A diagnostic dataset for compositional lan-
guage and elementary visual reasoning. In CVPR,
pages 2901–2910.
Johnson, J., Hariharan, B., van der Maaten, L., Hoffman, J.,
Fei-Fei, L., Zitnick, C. L., and Girshick, R. (2017b).
Inferring and executing programs for visual reasoning.
In ICCV.
Kamath, A., Singh, M., LeCun, Y., Synnaeve, G., Misra, I.,
and Carion, N. (2021). MDETR-modulated detection
for end-to-end multi-modal understanding. In ICCV,
pages 1780–1790.
Krishna, R., Zhu, Y., Groth, O., Johnson, J., Hata,
K., Kravitz, J., Chen, S., Kalantidis, Y., Li, L.-
J., Shamma, D. A., et al. (2017). Visual genome:
Connecting language and vision using crowdsourced
dense image annotations. Int. Journal of Computer
Vision, 123(1):32–73.
Lake, B. and Baroni, M. (2018). Generalization with-
out systematicity: On the compositional skills of
sequence-to-sequence recurrent networks. In ICML,
pages 2873–2882.
Li, L. H., Yatskar, M., Yin, D., Hsieh, C.-J., and Chang, K.-
W. (2020). What does BERT with vision look at? In
ACL, pages 5265–5275.
Liu, Y., Ott, M., Goyal, N., Du, J., Joshi, M., Chen,
D., Levy, O., Lewis, M., Zettlemoyer, L., and
Stoyanov, V. (2019). RoBERTa: A robustly opti-
mized BERT pretraining approach. arXiv preprint
arXiv:1907.11692.
Lu, J., Batra, D., Parikh, D., and Lee, S. (2019). ViL-
BERT: Pretraining task-agnostic visiolinguistic repre-
sentations for vision-and-language tasks. In NeurIPS,
pages 13–23.
Ruis, L., Andreas, J., Baroni, M., Bouchacourt, D., and
Lake, B. M. (2020). A benchmark for systematic gen-
eralization in grounded language understanding. In
NeurIPS, pages 19861–19872.
Sharma, P., Ding, N., Goodman, S., and Soricut, R. (2018).
Conceptual captions: A cleaned, hypernymed, image
alt-text dataset for automatic image captioning. In
ACL, volume 1, pages 2556–2565.
Shi, J., Zhang, H., and Li, J. (2019). Explainable and ex-
plicit visual reasoning over scene graphs. In CVPR,
pages 8376–8384.
Tan, H. and Bansal, M. (2019). LXMERT: Learning cross-
modality encoder representations from transformers.
In EMNLP and Proc. of the Conference on Empirical
Methods in Natural Language Processing and ICNLP,
pages 5100–5111.
Vaswani, A., Shazeer, N., Parmar, N., Uszkoreit, J., Jones,
L., Gomez, A. N., Kaiser, Ł., and Polosukhin, I.
(2017). Attention is all you need. In NeurIPS, pages
5998–6008.
Yamada, M., D’Amario, V., Takemoto, K., Boix, X., and
Sasaki, T. (2022). Transformer module networks for
systematic generalization in visual question answer-
ing. arXiv preprint arXiv:2201.11316.
Yi, K., Wu, J., Gan, C., Torralba, A., Kohli, P., and Tenen-
baum, J. (2018). Neural-symbolic VQA: Disentan-
gling reasoning from vision and language understand-
ing. In NeurIPS, pages 1039–1050.
Yu, L., Poirson, P., Yang, S., Berg, A. C., and Berg, T. L.
(2016). Modeling context in referring expressions. In
ECCV, pages 69–85.
VISAPP 2023 - 18th International Conference on Computer Vision Theory and Applications
606