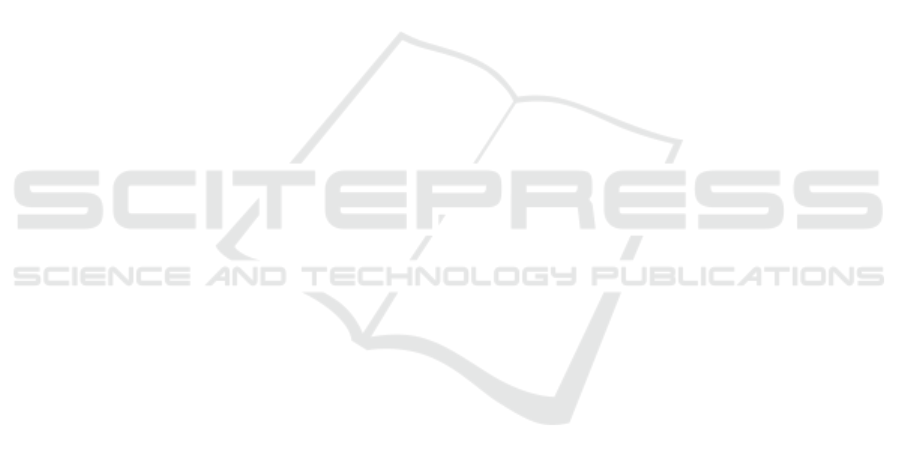
LeCun, Y., Bengio, Y., and Hinton, G. (2015). Deep learn-
ing. In Nature 521, pages 436–444.
Lee, S., Seon, J., Kyeong, C., Kim, S., Sun, Y., and Kim, J.
(2021). Novel energy trading system based on deep-
reinforcement learning in microgrids. In Energies,
pages 1–14. https://doi.org/10.3390/en14175515.
Lima, E., Gorski, E., Loures, E. F. R., Santos, E. A. P.,
and Deschamps, F. (2019). Applying machine learn-
ing to ahp multicriteria decision making method to as-
sets prioritization in the context of industrial mainte-
nance 4.0. In IFAC Papers On Line, pages 2152–2157.
https://doi.org/10.1016/j.ifacol.2019.11.524.
Liu, Y.-J., Cheng, S.-M., and Hsueh, Y.-L. (2017). enb se-
lection for machine type communications using rein-
forcement learning based markov decision process. In
eNB Selection for Machine Type Communications Us-
ing Reinforcement Learning Based Markov Decision
Process, pages 11330–11338.
L
´
opez, C. E., Cunningham, J., Ashour, O., and Tucker, C. S.
(2020). Deep reinforcement learning for procedural
content generation of 3d virtual environments. In J.
Comput. Inf. Sci. Eng., pages 1–9. https://doi.org/10.
1115/1.4046293.
Mirhoseini, A., Goldie, A., Yazgan, M., Jiang, J. W.,
Songhori, E., Wang, S., Lee, Y.-J., Johnson, E.,
Pathak, O., Nazi, A., Pak, J., Tong, A., Srinivasa, K.,
Hang, W., Tuncer, E., Le, Q. V., Laudon, J., Ho, R.,
Carpenter, R., and Dean, J. (2021). A graph place-
ment methodology for fast chip design. pages 207–
212. https://doi.org/10.1038/s41586-021-03544-w.
Mnih, V., Kavukcuoglu, K., Silver, D., Rusu, A. A., Ve-
ness, J., Bellemare, M. G., Graves, A., Riedmiller,
M., Fidjeland, A. K., Ostrovski, G., Petersen, S.,
Beattie, C., Sadik, A., Antonoglou, I., King, H., Ku-
maran, D., Wierstra, D., Legg, S., and Hassabis, D.
(2015). Human-level control through deep reinforce-
ment learning. In Nature volume 518, pages 529–533.
R.Butz, A. (1969). Convergence with hilbert’s space fill-
ing curve. In Journal of Computer and System
Sciences, pages 128–131. https://doi.org/10.1016/
S0022-0000(69)80010-3.
Silver, D., Huang, A., Maddison, C. J., Guez, A., Sifre, L.,
van den Driessche, G., Schrittwieser, J., Antonoglou,
I., Panneershelvam, V., Lanctot, M., Dieleman, S.,
Grewe, D., Nham, J., Kalchbrenner, N., Sutskever, I.,
Lillicrap, T., Leach, M., Kavukcuoglu, K., Graepel,
T., and Hassabis, D. (2016). Mastering the game of
go with deep neural networks and tree search. In Na-
ture volume 529, pages 484–489.
S.P.Singh and R.R.K.Sharma (2006). A review of differ-
ent approaches to the facility layout problems. In
The International Journal of Advanced Manufactur-
ing Technology, page 425. https://doi.org/10.1007/
s00170-005-0087-9.
Tam, K. Y. (1992). Genetic algorithms, function optimiza-
tion, and facility layout design. In European Jour-
nal of Operational Research, pages 322–330. https:
//doi.org/10.1016/0377-2217(92)90034-7.
Thengade, A. and Dondal, R. (2012). Genetic algorithm
– survey paper. In MPGI National Multi Conference
2012, pages 25–29. ISSN:0975-8887.
Vargas-Pardo, L. F. and Giraldo-Ramos, F. N. (2021). Fire-
fly algorithm for facility layout problemoptimization.
In Visi
´
on electr
´
onica, pages 33–38. https://doi.org/10.
14483/issn.2248-4728.
Verma, R., Saikia, S., Khadilkar, H., Agarwal, P., Shrof,
G., and Srinivasan, A. (2019). A reinforcement learn-
ing framework for container selection and ship load
sequencing in ports. In AAMAS, pages 2250–2252.
Wang, J. and Wang, L. (2020). Mobile edge comput-
ing task distribution and offloading algorithm based
on deep reinforcement learning in internet of vehi-
cles. In Journal of Ambient Intelligence and Human-
ized Computing, pages 1–7. https://doi.org/10.1007/
s12652-021-03458-5.
Wang, K., Ding, L., Dailami, F., and Matthews, J.
(2022). Ga-ahp method to support robotic polishing
process planning. In Digital Manufacturing Tech-
nology, pages 23–31. http://doi.org/10.37256/dmt.
2220221513.
WATKINS, C. J. and DAYAN, P. (1992). Q-learning. In
Machine Learning, pages 279–292. https://doi.org/10.
1007/BF00992698.
Yamashita, R., Nishio, M., Do, R. K. G., and Togashi,
K. (2018). Convolutional neural networks: an
overview and application in radiology. In Insights
Imaging 9, pages 611–620. https://doi.org/10.1007/
s13244-018-0639-9.
Yang, T., Zhang, S., and Li, C. (2021). A multi-objective
hyper-heuristic algorithm based on adaptive epsilon-
greedy selection. In Complex Intell. Syst., pages 765–
767. https://doi.org/10.1007/s40747-020-00230-8.
Yousefi, H., Ghodusinejad, M. H., and Noorollahi, Y.
(2021). Ga/ahp-based optimal design of a hybrid
cchp system considering economy, energy and emis-
sion. In Manufacturing Letters, pages 309–313. https:
//doi.org/10.1016/j.enbuild.2016.12.048.
Zeng, Y., Liu, W., Liu, Z., Zhang, J., and Niu, W.
(2010). Improved genetic algorithm and ana-
lytic hierarchy process for electric power equip-
ment maintenance schedule optimization. In Power
and Energy Engineering Conference, pages 491–495.
978-1-935068-17-4.
ICAART 2023 - 15th International Conference on Agents and Artificial Intelligence
230