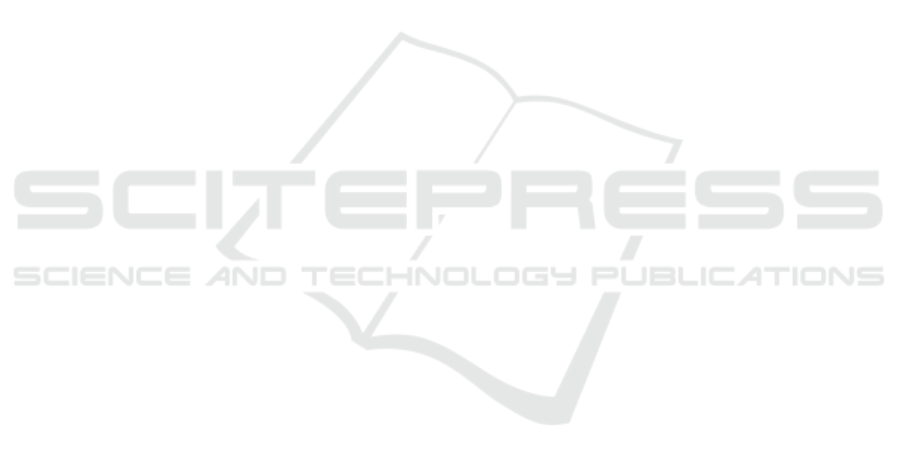
effort and is impractical in real-world scenarios.
In this study, we introduce a novel data-driven
computational method that can classify depression
and estimate its severity level without using a class la-
bel. The proposed model is motivated by the fact that
reduced mobility is an early indication of depression.
At first, a graph-based correlation network is con-
structed using the mobility data collected from wear-
able sensors and employing a clustering algorithm to
extract strongly connected communities (clusters) in
the graph. The advantage of employing the correla-
tion network model is that its underlying graph inher-
ently possesses the potential communities, and they
can be identified by s suitable community detection
clustering algorithm such as MCL. The obtained net-
work also has several graph-theoretic properties that
can be utilized to further analyze the mobility data.
Taking advantage of such properties, we have devel-
oped a new metric, Depression Severity Score index
(DSS), by using graph metrics including inter and
intra-cluster density. The obtained results demon-
strate that the correlation between measured DSS and
clinical depression rating score is high. We envision
that DSS can be used as a supplementary tool for clin-
icians and healthcare professionals in obtaining ob-
jective diagnostic assessment.
REFERENCES
Benesty, J., Chen, J., Huang, Y., and Cohen, I. (2009).
Pearson correlation coefficient. In Noise reduction in
speech processing, pages 1–4. Springer.
Bondy, J. A., Murty, U. S. R., et al. (1976). Graph theory
with applications, volume 290. Macmillan London.
Cai, B.-J., Wang, H.-Y., Zheng, H.-R., and Wang, H. (2010).
Evaluation repeated random walks in community de-
tection of social networks. In 2010 International Con-
ference on Machine Learning and Cybernetics, vol-
ume 4, pages 1849–1854. IEEE.
Emmons, S., Kobourov, S., Gallant, M., and B
¨
orner, K.
(2016). Analysis of network clustering algorithms
and cluster quality metrics at scale. PloS one,
11(7):e0159161.
Garcia-Ceja, E., Riegler, M., Jakobsen, P., Tørresen, J.,
Nordgreen, T., Oedegaard, K. J., and Fasmer, O. B.
(2018). Depresjon: a motor activity database of de-
pression episodes in unipolar and bipolar patients. In
Proceedings of the 9th ACM multimedia systems con-
ference, pages 472–477.
Li, Y., Babcock, S. E., Stewart, S. L., Hirdes, J. P., and
Schwean, V. L. (2021). Psychometric evaluation of
the depressive severity index (dsi) among children and
youth using the interrai child and youth mental health
(chymh) assessment tool. In Child & Youth Care Fo-
rum, volume 50, pages 611–630. Springer.
Mathers, C. D. and Loncar, D. (2006). Projections of global
mortality and burden of disease from 2002 to 2030.
PLoS medicine, 3(11):e442.
Mazza, M. G., De Lorenzo, R., Conte, C., Poletti, S., Vai,
B., Bollettini, I., Melloni, E. M. T., Furlan, R., Ciceri,
F., Rovere-Querini, P., et al. (2020). Anxiety and de-
pression in covid-19 survivors: Role of inflammatory
and clinical predictors. Brain, behavior, and immu-
nity, 89:594–600.
Mclean, C., He, X., Simpson, I. T., and Armstrong, D. J.
(2016). Improved functional enrichment analysis of
biological networks using scalable modularity based
clustering. Journal of Proteomics & Bioinformatics,
9(1):9–18.
Montgomery, S. A. and
˚
Asberg, M. (1979). A new depres-
sion scale designed to be sensitive to change. The
British journal of psychiatry, 134(4):382–389.
The National Institute of Mental Health (NIMH) (2021).
Depression. [Online; accessed 8-August-2021].
Thelagathoti, R. and Ali, H. (2022a). A population analysis
approach using mobility data and correlation networks
for depression episodes detection. Ann Depress Anxi-
ety, 9(1):1112.
Thelagathoti, R. K. and Ali, H. H. (2022b). The compari-
son of various correlation network models in studying
mobility data for the analysis of depression episodes.
In BIOSIGNALS, pages 200–207.
Williams, J. B. (1988). A structured interview guide for the
hamilton depression rating scale. Archives of general
psychiatry, 45(8):742–747.
A Correlation Network Model for Analyzing Mobility Data in Depression Related Studies
423