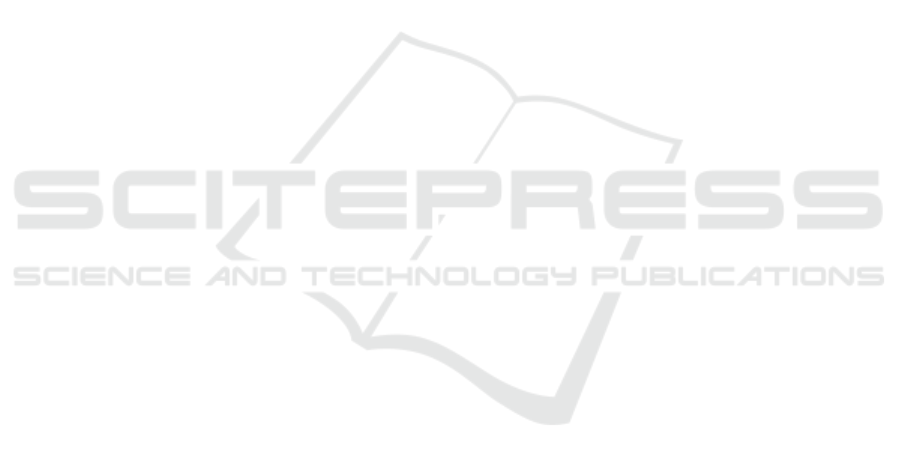
REFERENCES
Aziz, O., Klenk, J., Schwickert, L., Chiari, L., Becker, C.,
Park, E. J., Mori, G., and Robinovitch, S. N. (2017).
Validation of accuracy of svm-based fall detection
system using real-world fall and non-fall datasets.
PLoS one, 12(7):e0180318.
Bagala, F., Becker, C., Cappello, A., Chiari, L., Aminian,
K., Hausdorff, J. M., Zijlstra, W., and Klenk, J.
(2012). Evaluation of accelerometer-based fall de-
tection algorithms on real-world falls. PloS one,
7(5):e37062.
Berlin, S. J. and John, M. (2021). Vision based human fall
detection with siamese convolutional neural networks.
Journal of Ambient Intelligence and Humanized Com-
puting, pages 1–12.
Birku, Y. and Agrawal, H. (2018). Survey on fall detection
systems. International Journal of Pure and Applied
Mathematics, 118(18):2537–2543.
Chan, B. K., Marshall, L. M., Winters, K. M., Faulkner,
K. A., Schwartz, A. V., and Orwoll, E. S. (2007).
Incident fall risk and physical activity and physical
performance among older men: the osteoporotic frac-
tures in men study. American journal of epidemiology,
165(6):696–703.
El Kaid, A., Ba
¨
ına, K., and Ba
¨
ına, J. (2019a). Real
case study of improving elderly person fall video-
detection algorithm. In Conference on Complexity
Analysis of Industrial Systems and Advanced Model-
ing - CAISAM’19, Ben Guerir, Morocco.
El Kaid, A., Ba
¨
ına, K., and Ba
¨
ına, J. (2019b). Reduce false
positive alerts for elderly person fall video-detection
algorithm by convolutional neural network model.
Procedia computer science, 148:2–11.
El Kaid, A., Brazey, D., Barra, V., and Ba
¨
ına, K.
(2022). Top-down system for multi-person 3d abso-
lute pose estimation from monocular videos. Sensors,
22(11):4109.
Feng, W., Liu, R., and Zhu, M. (2014). Fall detection for el-
derly person care in a vision-based home surveillance
environment using a monocular camera. signal, image
and video processing, 8(6):1129–1138.
Galv
˜
ao, Y. M., Albuquerque, V. A., Fernandes, B. J., and
Valenc¸a, M. J. (2017). Anomaly detection in smart
houses: Monitoring elderly daily behavior for fall de-
tecting. In 2017 IEEE Latin American Conference
on Computational Intelligence (LA-CCI), pages 1–6.
IEEE.
Galv
˜
ao, Y. M., Ferreira, J., Albuquerque, V. A., Barros, P.,
and Fernandes, B. J. (2021). A multimodal approach
using deep learning for fall detection. Expert Systems
with Applications, 168:114226.
Grandini, M., Bagli, E., and Visani, G. (2020). Metrics for
multi-class classification: an overview. arXiv preprint
arXiv:2008.05756.
Guti
´
errez, J., Rodr
´
ıguez, V., and Martin, S. (2021). Com-
prehensive review of vision-based fall detection sys-
tems. Sensors, 21(3):947.
Habib, M. A., Mohktar, M. S., Kamaruzzaman, S. B., Lim,
K. S., Pin, T. M., and Ibrahim, F. (2014). Smartphone-
based solutions for fall detection and prevention: chal-
lenges and open issues. Sensors, 14(4):7181–7208.
Ismaili-Alaoui, A., Ba
¨
ına, K., and Benali, K. (2022). Iodep:
Towards an iot-data analysis and event processing ar-
chitecture for business process incident management.
International journal of advanced computer science
and applications (IJACSA), 13(4).
Ismaili-Alaoui, A., Kasmi, O., Ba
¨
ına, A., Ba
¨
ına, K., Benali,
K., and Bellafkih, M. (2019). Priority-based event
management using fuzzy logic for an iot-bpm archi-
tecture. In 2019 IEEE 12th Conference on Service-
Oriented Computing and Applications (SOCA), pages
111–118. IEEE.
Jarraya, S. K. (2018). Computer vision-based fall detection
methods using the kinect camera: A survey. Inter-
national Journal of Computer Science & Information
Technology (IJCSIT) Vol, 10.
Karantonis, D. M., Narayanan, M. R., Mathie, M., Lovell,
N. H., and Celler, B. G. (2006). Implementa-
tion of a real-time human movement classifier us-
ing a triaxial accelerometer for ambulatory monitor-
ing. IEEE transactions on information technology in
biomedicine, 10(1):156–167.
Mubashir, M., Shao, L., and Seed, L. (2013). A survey on
fall detection: Principles and approaches. Neurocom-
puting, 100:144–152.
Noury, N., Fleury, A., Rumeau, P., Bourke, A. K., Laighin,
G., Rialle, V., and Lundy, J.-E. (2007). Fall detection-
principles and methods. In 2007 29th Annual In-
ternational Conference of the IEEE Engineering in
Medicine and Biology Society, pages 1663–1666.
IEEE.
¨
Ozdemir, A. T. and Barshan, B. (2014). Detecting falls with
wearable sensors using machine learning techniques.
Sensors, 14(6):10691–10708.
Rastogi, S. and Singh, J. (2021). A systematic review on
machine learning for fall detection system. Computa-
tional Intelligence, 37(2):951–974.
Vignat, J. (2001). Aspects psychiques de la chute. Neurol
Psychiatr Geriatr, 1:12–6.
Vishwakarma, V., Mandal, C., and Sural, S. (2007). Au-
tomatic detection of human fall in video. In Interna-
tional conference on pattern recognition and machine
intelligence, pages 616–623. Springer.
Wild, D., Nayak, U., and Isaacs, B. (1981). How dangerous
are falls in old people at home? Br Med J (Clin Res
Ed), 282(6260):266–268.
World Health Organization. Falls. https://www.who.int/en/
news-room/fact-sheets/detail/falls.
Xu, T., Zhou, Y., and Zhu, J. (2018). New advances and
challenges of fall detection systems: A survey. Ap-
plied Sciences, 8(3):418.
Yu, X. (2008). Approaches and principles of fall detection
for elderly and patient. In HealthCom 2008-10th In-
ternational Conference on e-health Networking, Ap-
plications and Services, pages 42–47. IEEE.
VISAPP 2023 - 18th International Conference on Computer Vision Theory and Applications
582