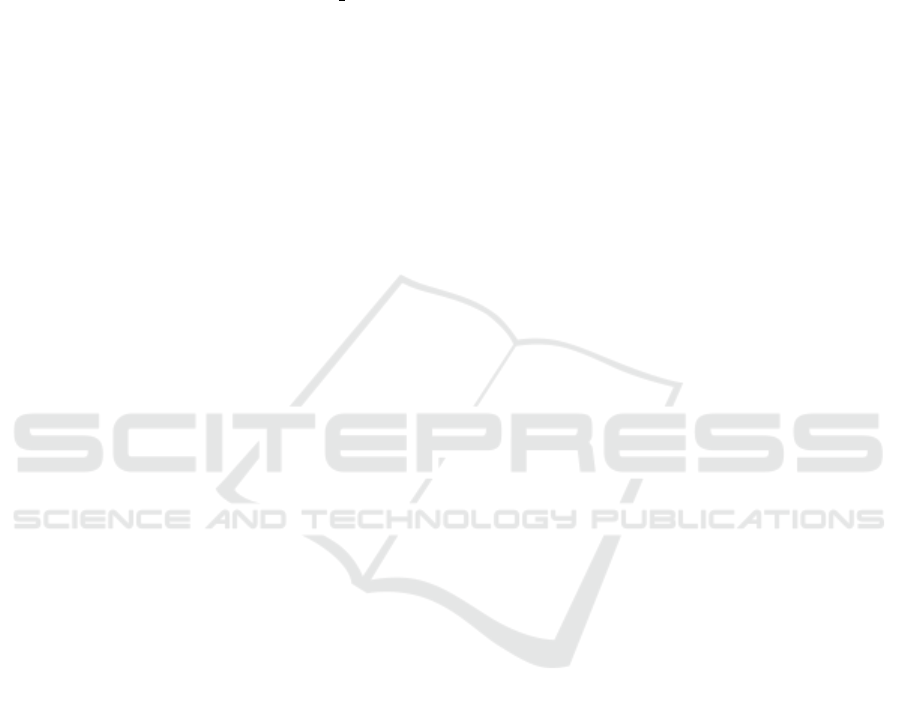
ACKNOWLEDGEMENTS
This work was supported by the EU Hori-
zon 2020 program MSCA-101007666; by
MCIN/AEI/10.13039/501100011033 and by the
NextGeneration-EU/PRTR under Grants PDC2021-
120846-C41 & PID2021-126061OB-C44, and by the
Government of Aragon (Grant Group T36 20R).
REFERENCES
Al-nasheri, A., Muhammad, G., Alsulaiman, M., Ali,
Z., Mesallam, T. A., Farahat, M., Malki, K. H.,
and Bencherif, M. A. (2016). An Investiga-
tion of Multidimensional Voice Program Parame-
ters in Three Different Databases for Voice Pathol-
ogy Detection and Classification. Journal of Voice,
31(1):113.e9–113.e18.
Aldraimli, M., Soria, D., Parkinson, J., Thomas, E., Bell, J.,
Dwek, M., and Chaussalet, T. (2020). Machine learn-
ing prediction of susceptibility to visceral fat associ-
ated diseases. Health and Technology, 10:925–944.
Ali, Z., Alsulaiman, M., Elamvazuthi, G. M. I., Al-Nasheri,
A., Mesallam, T., Farahat, M., and Malki, K. (2017a).
Intra- and Inter-Database Study for Arabic, English,
and German Databases: Do Conventional Speech Fea-
tures Detect Voice Pathology? Journal of Voice,
31:386.e1–386.e8.
Ali, Z., Muhammad, G., and Alhamid, M. F. (2017b). An
automatic health monitoring system for patients suf-
fering from voice complications in smart cities. IEEE
Access, 5:3900–3908.
Barche, P., Gurugubelli, K., and Vuppala, A. K. (2020).
Towards automatic assessment of voice disorders: A
clinical approach. In Proc. Interspeech 2018, pages
2537–2541.
Bhattacharyya, N. (2014). The prevalence of voice prob-
lems among adults in the United States. The Laryngo-
scope, 124:2359–2362.
Chawla, N. V., Bowyer, K. W., Hall, L. O., and Kegelmeyer,
W. P. (2002). Smote: Synthetic minority over-
sampling technique. Journal of Artificial Intelligence
Research, 16(1):321–357.
Chui, K. T., Lytras, M. D., and Vasant, P. (2020). Com-
bined generative adversarial network and fuzzy c-
means clustering for multi-class voice disorder detec-
tion with an imbalanced dataset. Applied Sciences,
10(13).
Fan, Z., Wu, Y., Zhou, C., Zhang, X., and Tao, Z. (2021).
Class-imbalanced voice pathology detection and clas-
sification using fuzzy cluster oversampling method.
Applied Sciences, 11(8).
Har
´
ar, P., Alonso, J., Mekyska, J., Gal
´
a
ˇ
z, Z., Burget, R., and
Smekal, Z. (2017). Voice pathology detection using
deep learning: a preliminary study. pages 1–4.
He, H., Bai, Y., Garcia, E. A., and Li, S. (2008). Adasyn:
Adaptive synthetic sampling approach for imbalanced
learning. In 2008 IEEE International Joint Confer-
ence on Neural Networks (IEEE World Congress on
Computational Intelligence), pages 1322–1328.
He, H. and Ma, Y. (2013). Imbalanced Learning: Foun-
dations, Algorithms, and Applications. Wiley-IEEE
Press.
Huckvale, M. and Buciuleac, C. (2021). Automated De-
tection of Voice Disorder in the Saarbr
¨
ucken Voice
Database: Effects of Pathology Subset and Audio Ma-
terials. In Interspeech, pages 1399–1403.
Jesus, L. M., Belo, I., Machado, J., and Hall, A. (2017). The
advanced voice function assessment databases (av-
fad): Tools for voice clinicians and speech research.
In Fernandes, F. D. M., editor, Advances in Speech-
language Pathology, chapter 14. IntechOpen, Rijeka.
Mohammed, M. A., Abdulkareem, K. H., Mostafa, S. A.,
Khanapi Abd Ghani, M., Maashi, M. S., Garcia-
Zapirain, B., Oleagordia, I., Alhakami, H., and AL-
Dhief, F. T. (2020). Voice pathology detection
and classification using convolutional neural network
model. Applied Sciences, 10(11).
Nguyen, H. M., Cooper, E. W., and Kamei, K. (2011).
Borderline over-sampling for imbalanced data clas-
sification. Int. J. Knowl. Eng. Soft Data Paradigm.,
3(1):4–21.
Priya, E., S, J. P., Reshma, P. S., and S, S. (2022). Tem-
poral and spectral features based gender recognition
from audio signals. In 2022 International Conference
on Communication, Computing and Internet of Things
(IC3IoT), pages 1–5.
P
¨
utzer, M. and Koreman, J. (1997). A German database of
pathological vocal fold vibration. pages 143–153.
Roy, N., Merrill, R. M., Thibeault, S., Parsa, R. A., Gray,
S. D., and Smith, E. M. (2004). Prevalence of Voice
Disorders in Teachers and the General Population.
Journal of Speech Language and Hearing Research,
47:281–293.
Sarika Hegde, Surendra Shetty, S. R. and Dodderi, T.
(2019). A Survey on Machine Learning Approaches
for Automatic Detection of Voice Disorders. Journal
of Voice, 33(6):947.e11–947.e33.
Sch
¨
olkopf, B. and Smola, A. J. (2002). Learning with ker-
nels : support vector machines, regularization, opti-
mization, and beyond. Adaptive computation and ma-
chine learning. MIT Press.
Teixeira, J. P., Oliveira, C., and Lopes, C. (2013). Vocal
acoustic analysis – jitter, shimmer and hnr parameters.
Procedia Technology, 9(Complete):1112–1122.
Verde, L., Pietro, G. D., Alrashoud, M., Ghoneim, A., Al-
Mutib, K. N., and Sannino, G. (2019). Leveraging
artificial intelligence to improve voice disorder identi-
fication through the use of a reliable mobile app. IEEE
Access, 7:124048–124054.
Weninger, F., Eyben, F., Schuller, B., Mortillaro, M., and
Scherer, K. R. (2013). On the acoustics of emo-
tion in audio: What speech, music, and sound have
in common. Frontiers in Psychology, 4(292). ID:
unige:97889.
On the Problem of Data Availability in Automatic Voice Disorder Detection
337