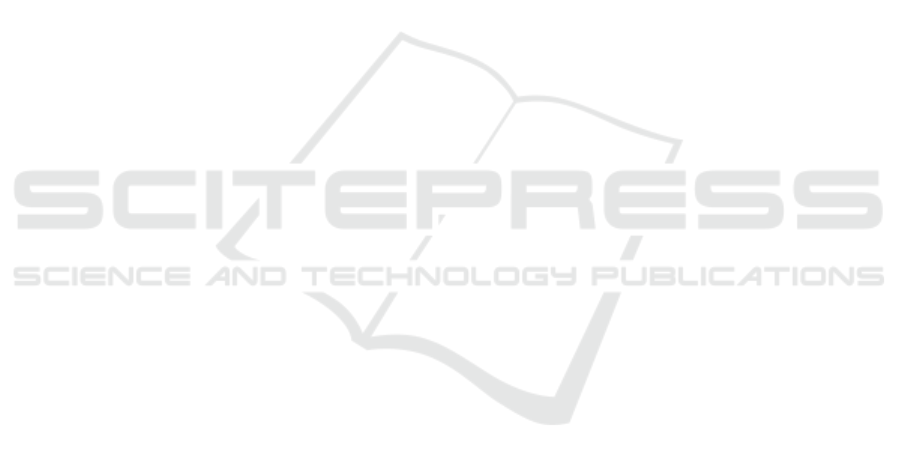
process. By using ground points, we do not need to
make 3D back-projections to perform the matching,
and by comparing only one point per person, we can
obtain the corresponding poses in two views. Besides
that, instead of comparing the 2D poses with MSE,
we use a smooth L
1
loss. The results show a huge po-
tential for using unsupervised losses instead of super-
vised ones based on 3D annotations. In future work,
we intend to do experiments on more datasets and to
refine the loss using other regularizers such as Jensen-
Shanon (Fuglede and Topsoe, 2004).
REFERENCES
Belagiannis, V., Amin, S., Andriluka, M., Schiele, B.,
Navab, N., and Ilic, S. (2014a). 3d pictorial structures
for multiple human pose estimation. In Proceedings of
the IEEE Conference on Computer Vision and Pattern
Recognition, pages 1669–1676.
Belagiannis, V., Amin, S., Andriluka, M., Schiele, B.,
Navab, N., and Ilic, S. (2015). 3d pictorial structures
revisited: Multiple human pose estimation. IEEE
transactions on pattern analysis and machine intelli-
gence, 38(10):1929–1942.
Belagiannis, V., Wang, X., Schiele, B., Fua, P., Ilic, S.,
and Navab, N. (2014b). Multiple human pose estima-
tion with temporally consistent 3d pictorial structures.
In European Conference on Computer Vision, pages
742–754. Springer.
Brynte, L. and Kahl, F. (2020). Pose proposal critic: Robust
pose refinement by learning reprojection errors. arXiv
preprint arXiv:2005.06262.
de Franc¸a Silva, D. W., do Monte Lima, J. P. S., Mac
ˆ
edo,
D., Zanchettin, C., Thomas, D. G. F., Uchiyama, H.,
and Teichrieb, V. (2022). Unsupervised multi-view
multi-person 3d pose estimation using reprojection er-
ror. In International Conference on Artificial Neural
Networks, pages 482–494. Springer.
Dong, J., Jiang, W., Huang, Q., Bao, H., and Zhou, X.
(2019). Fast and robust multi-person 3d pose esti-
mation from multiple views. In Proceedings of the
IEEE/CVF Conference on Computer Vision and Pat-
tern Recognition, pages 7792–7801.
Fuglede, B. and Topsoe, F. (2004). Jensen-shannon diver-
gence and hilbert space embedding. In International
Symposium onInformation Theory, 2004. ISIT 2004.
Proceedings., page 31. IEEE.
Girshick, R. (2015). Fast r-cnn. In Proceedings of the IEEE
international conference on computer vision, pages
1440–1448.
Hartley, R. and Zisserman, A. (2003). Multiple view geom-
etry in computer vision. Cambridge university press.
Huang, C., Jiang, S., Li, Y., Zhang, Z., Traish, J., Deng,
C., Ferguson, S., and Xu, R. Y. D. (2020). End-to-
end dynamic matching network for multi-view multi-
person 3d pose estimation. In European Conference
on Computer Vision, pages 477–493. Springer.
Ionescu, C., Papava, D., Olaru, V., and Sminchisescu, C.
(2013). Human3. 6m: Large scale datasets and pre-
dictive methods for 3d human sensing in natural envi-
ronments. IEEE transactions on pattern analysis and
machine intelligence, 36(7):1325–1339.
Kuhn, H. W. (1955). The hungarian method for the as-
signment problem. Naval research logistics quarterly,
2(1-2):83–97.
Li, Z., Ye, J., Song, M., Huang, Y., and Pan, Z. (2021). On-
line knowledge distillation for efficient pose estima-
tion. In Proceedings of the IEEE/CVF International
Conference on Computer Vision, pages 11740–11750.
Lima, J. P., Roberto, R., Figueiredo, L., Simoes, F., and
Teichrieb, V. (2021). Generalizable multi-camera 3d
pedestrian detection. In Proceedings of the IEEE/CVF
conference on computer vision and pattern recogni-
tion, pages 1232–1240.
Lin, J. and Lee, G. H. (2021). Multi-view multi-person 3d
pose estimation with plane sweep stereo. In Proceed-
ings of the IEEE/CVF Conference on Computer Vision
and Pattern Recognition, pages 11886–11895.
Lin, T.-Y., Maire, M., Belongie, S., Hays, J., Perona, P.,
Ramanan, D., Doll
´
ar, P., and Zitnick, C. L. (2014).
Microsoft coco: Common objects in context. In Euro-
pean conference on computer vision, pages 740–755.
Springer.
Sun, C., Thomas, D., and Kawasaki, H. (2021). Unsuper-
vised 3d human pose estimation in multi-view-multi-
pose video. In 2020 25th International Conference on
Pattern Recognition (ICPR), pages 5959–5964. IEEE.
Sun, K., Xiao, B., Liu, D., and Wang, J. (2019). Deep high-
resolution representation learning for human pose es-
timation. In Proceedings of the IEEE/CVF conference
on computer vision and pattern recognition, pages
5693–5703.
Tu, H., Wang, C., and Zeng, W. (2020). Voxelpose: To-
wards multi-camera 3d human pose estimation in wild
environment. In European Conference on Computer
Vision, pages 197–212. Springer.
Wang, J., Tan, S., Zhen, X., Xu, S., Zheng, F., He, Z., and
Shao, L. (2021). Deep 3d human pose estimation: A
review. Computer Vision and Image Understanding,
210:103225.
Xiu, Y., Li, J., Wang, H., Fang, Y., and Lu, C. (2018). Pose
Flow: Efficient online pose tracking. In BMVC.
VISAPP 2023 - 18th International Conference on Computer Vision Theory and Applications
614