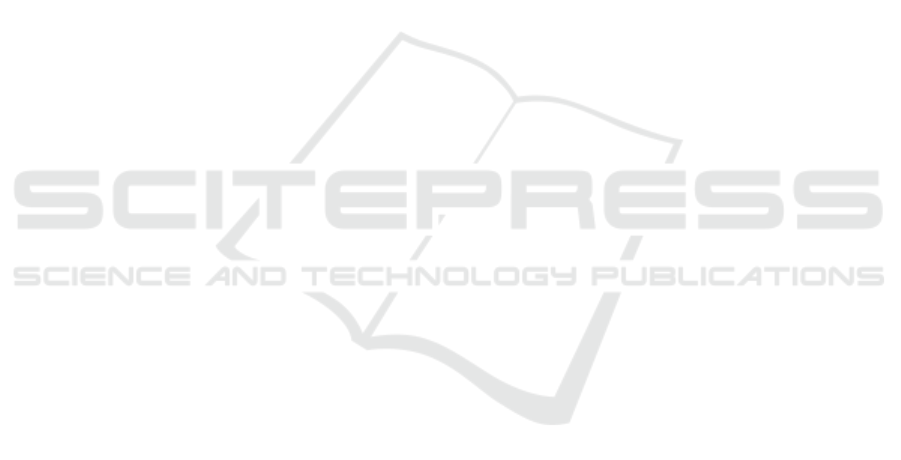
the relevant instances that were missed, both for Sce-
nario A and Scenario B, we can comment that this
happened because these two scenarios require an im-
age as an input, which is analyzed and the Top-3 seg-
mented labels (i.e., Top-3 object labels that are most
likely contained in the image) are considered in order
to find similar images from the KG (see subsection
3.3). Therefore, the missed relevant images contained
Top-3 segmented labels which did not exist in any im-
age in our KG simultaneously.
As for future work, our plan is to enrich the
MindSpaces KG with domain-knowledge from rele-
vant Semantic Web KGs, such as ConceptNet (Speer
et al., 2017) and WordNet (Fellbaum, 2010), and
compare the knowledge in the MindSpaces KG with
other existing KG related to the indoor and urban
adaptive design. Moreover, we will investigate and
expand the quantity of real-life scenarios that the rea-
soning mechanism can support. Finally, we also plan
to create a more friendly user interface, as at the mo-
ment the queries are formulated through SPARQL.
ACKNOWLEDGEMENTS
This work has been supported by the EC-funded
project MindSpaces (H2020-825079)
REFERENCES
Andreadis, S., Moumtzidou, A., Apostolidis, K., Gkoun-
takos, K., Galanopoulos, D., Michail, E., Gialam-
poukidis, I., Vrochidis, S., Mezaris, V., and Kompat-
siaris, I. (2020). Verge in vbs 2020. In International
Conference on Multimedia Modeling, pages 778–783.
Springer.
Asim, M. N., Wasim, M., Khan, M. U. G., Mahmood, N.,
and Mahmood, W. (2019). The use of ontology in
retrieval: a study on textual, multilingual, and multi-
media retrieval. IEEE Access, 7:21662–21686.
Berners-Lee, T., Hendler, J., and Lassila, O. (2001). The
semantic web. Scientific american, 284(5):34–43.
Chi, N.-W., Jin, Y.-H., and Hsieh, S.-H. (2019). Developing
base domain ontology from a reference collection to
aid information retrieval. Automation in Construction,
100:180–189.
Fellbaum, C. (2010). Wordnet. In Theory and applications
of ontology: computer applications, pages 231–243.
Springer.
Hyv
¨
onen, E. (2012). Publishing and using cultural heritage
linked data on the semantic web. Synthesis lectures on
the semantic web: theory and technology, 2(1):1–159.
Kumar, V. R. S., Khamis, A., Fiorini, S., Carbonera, J. L.,
Alarcos, A. O., Habib, M., Goncalves, P., Li, H., and
Olszewska, J. I. (2019). Ontologies for industry 4.0.
The Knowledge Engineering Review, 34.
Lopes, D. M. (2007). Shikinen sengu and the ontology of
architecture in japan. The Journal of aesthetics and
art criticism, 65(1):77–84.
Lynch, K. et al. (1960). The image of the city (vol. 11).
Munir, K. and Anjum, M. S. (2018). The use of ontolo-
gies for effective knowledge modelling and informa-
tion retrieval. Applied Computing and Informatics,
14(2):116–126.
Pistola, T., Georgakopoulou, N., Shvets, A., Chatzis-
tavros, K., Xefteris, V.-R., Garc
´
ıa, A. T., Koulalis, I.,
Diplaris, S., Wanner, L., Vrochidis, S., et al. (2022).
Imageability-based multi-modal analysis of urban en-
vironments for architects and artists. In International
Conference on Image Analysis and Processing, pages
198–209. Springer.
Qiu, W., Li, W., Liu, X., and Huang, X. (2021). Subjective
street scene perceptions for shanghai with large-scale
application of computer vision and machine learning.
Technical report, EasyChair.
Raven, D., de Boer, V., Esmeijer, E., and Oomen, J. (2020).
Modeling ontologies for individual artists. Vrije Uni-
versiteit Amsterdam.
Schneider, A. (2020). Alternatives: World ontologies and
dialogues between contemporary arts and anthropolo-
gies. In Alternative Art and Anthropology, pages 1–
26. Routledge.
Speer, R., Chin, J., and Havasi, C. (2017). Conceptnet 5.5:
An open multilingual graph of general knowledge. In
Thirty-first AAAI conference on artificial intelligence.
Su
´
arez-Figueroa, M. C., G
´
omez-P
´
erez, A., and Villaz
´
on-
Terrazas, B. (2009). How to write and use the
ontology requirements specification document. In
OTM Confederated International Conferences” On
the Move to Meaningful Internet Systems”, pages
966–982. Springer.
Vassiliades, A., Bassiliades, N., Gouidis, F., and Patkos, T.
(2020). A knowledge retrieval framework for house-
hold objects and actions with external knowledge. In
International Conference on Semantic Systems, pages
36–52. Springer, Cham.
Wagner, A. and R
¨
uppel, U. (2019). Bpo: The building
product ontology for assembled products. In Proceed-
ings of the 7th Linked Data in Architecture and Con-
struction workshop (LDAC 2019)’, Lisbon, Portugal,
page 12.
Yu, B. (2019). Research on information retrieval model
based on ontology. EURASIP Journal on Wireless
Communications and Networking, 2019(1):1–8.
The MindSpaces Knowledge Graph: Applied Logic and Semantics on Indoor and Urban Adaptive Design
341