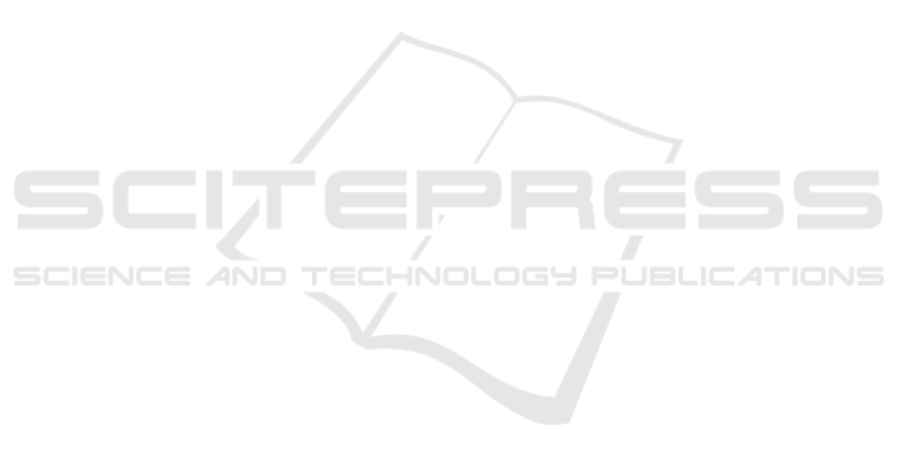
Conneau, A., Khandelwal, K., Goyal, N., Chaudhary, V.,
Wenzek, G., Guzm
´
an, F., Grave, E., Ott, M., Zettle-
moyer, L., and Stoyanov, V. (2019). Unsupervised
cross-lingual representation learning at scale. arXiv
preprint arXiv:1911.02116.
Cortiz, D. (2021). Exploring transformers in emotion recog-
nition: a comparison of bert, distillbert, roberta, xlnet
and electra. arXiv preprint arXiv:2104.02041.
Devlin, J., Chang, M.-W., Lee, K., and Toutanova, K.
(2018). Bert: Pre-training of deep bidirectional trans-
formers for language understanding. arXiv preprint
arXiv:1810.04805.
Dixon, L., Li, J., Sorensen, J., Thain, N., and Vasserman,
L. (2018). Measuring and mitigating unintended bias
in text classification. In Proceedings of the 2018
AAAI/ACM Conference on AI, Ethics, and Society,
pages 67–73.
Garrido-Mu
˜
noz, I., Montejo-R
´
aez, A., Mart
´
ınez-Santiago,
F., and Ure
˜
na-L
´
opez, L. A. (2021). A survey on bias
in deep nlp. Applied Sciences, 11(7):3184.
He, P., Liu, X., Gao, J., and Chen, W. (2020). Deberta:
Decoding-enhanced bert with disentangled attention.
arXiv preprint arXiv:2006.03654.
Huang, Q. and Xiao, Y. (2015). Geographic situational
awareness: mining tweets for disaster preparedness,
emergency response, impact, and recovery. ISPRS
International Journal of Geo-Information, 4(3):1549–
1568.
Kumar, A., Singh, J. P., and Saumya, S. (2019). A com-
parative analysis of machine learning techniques for
disaster-related tweet classification. In 2019 IEEE
R10 Humanitarian Technology Conference (R10-
HTC)(47129), pages 222–227. IEEE.
Kumar, P. and Dhinesh Babu, L. (2019). Novel text prepro-
cessing framework for sentiment analysis. In Smart
intelligent computing and applications, pages 309–
317. Springer.
Lan, Z., Chen, M., Goodman, S., Gimpel, K., Sharma,
P., and Soricut, R. (2019). Albert: A lite bert for
self-supervised learning of language representations.
arXiv preprint arXiv:1909.11942.
Li, H., Caragea, D., Caragea, C., and Herndon, N. (2018).
Disaster response aided by tweet classification with a
domain adaptation approach. Journal of Contingen-
cies and Crisis Management, 26(1):16–27.
Liu, J., Singhal, T., Blessing, L. T., Wood, K. L., and Lim,
K. H. (2021). Crisisbert: a robust transformer for cri-
sis classification and contextual crisis embedding. In
Proceedings of the 32nd ACM Conference on Hyper-
text and Social Media, pages 133–141.
Liu, Y., Ott, M., Goyal, N., Du, J., Joshi, M., Chen, D.,
Levy, O., Lewis, M., Zettlemoyer, L., and Stoyanov,
V. (2019). Roberta: A robustly optimized bert pre-
training approach. arXiv preprint arXiv:1907.11692.
Murayama, T., Wakamiya, S., and Aramaki, E. (2021).
Mitigation of diachronic bias in fake news detection
dataset. arXiv preprint arXiv:2108.12601.
Neri, F., Aliprandi, C., Capeci, F., Cuadros, M., and By, T.
(2012). Sentiment analysis on social media. In 2012
IEEE/ACM international conference on advances in
social networks analysis and mining, pages 919–926.
IEEE.
Nguyen, D. T., Al Mannai, K. A., Joty, S., Sajjad, H., Im-
ran, M., and Mitra, P. (2017). Robust classification
of crisis-related data on social networks using con-
volutional neural networks. In Eleventh international
AAAI conference on web and social media.
Nikolov, A. and Radivchev, V. (2019). Nikolov-radivchev
at semeval-2019 task 6: Offensive tweet classification
with bert and ensembles. In Proceedings of the 13th
international workshop on semantic evaluation, pages
691–695.
Olteanu, A., Castillo, C., Diaz, F., and Vieweg, S. (2014).
Crisislex: A lexicon for collecting and filtering mi-
croblogged communications in crises. In Eighth in-
ternational AAAI conference on weblogs and social
media.
Sanh, V., Debut, L., Chaumond, J., and Wolf, T. (2019).
Distilbert, a distilled version of bert: smaller, faster,
cheaper and lighter. arXiv preprint arXiv:1910.01108.
Schwartz, R., Dodge, J., Smith, N. A., and Etzioni, O.
(2020). Green ai. Communications of the ACM,
63(12):54–63.
Strubell, E., Ganesh, A., and McCallum, A. (2019). En-
ergy and policy considerations for deep learning in
nlp. arXiv preprint arXiv:1906.02243.
Uysal, A. K. and Gunal, S. (2014). The impact of prepro-
cessing on text classification. Information processing
& management, 50(1):104–112.
Vaswani, A., Shazeer, N., Parmar, N., Uszkoreit, J., Jones,
L., Gomez, A. N., Kaiser, Ł., and Polosukhin, I.
(2017). Attention is all you need. Advances in neural
information processing systems, 30.
Zahra, K., Imran, M., and Ostermann, F. O. (2020). Auto-
matic identification of eyewitness messages on twitter
during disasters. Information processing & manage-
ment, 57(1):102107.
Zhou, B., Zou, L., Mostafavi, A., Lin, B., Yang, M.,
Gharaibeh, N., Cai, H., Abedin, J., and Mandal, D.
(2022). Victimfinder: Harvesting rescue requests in
disaster response from social media with bert. Com-
puters, Environment and Urban Systems, 95:101824.
A Comparative Study of Deep Learning Methods for the Detection and Classification of Natural Disasters from Social Media
327