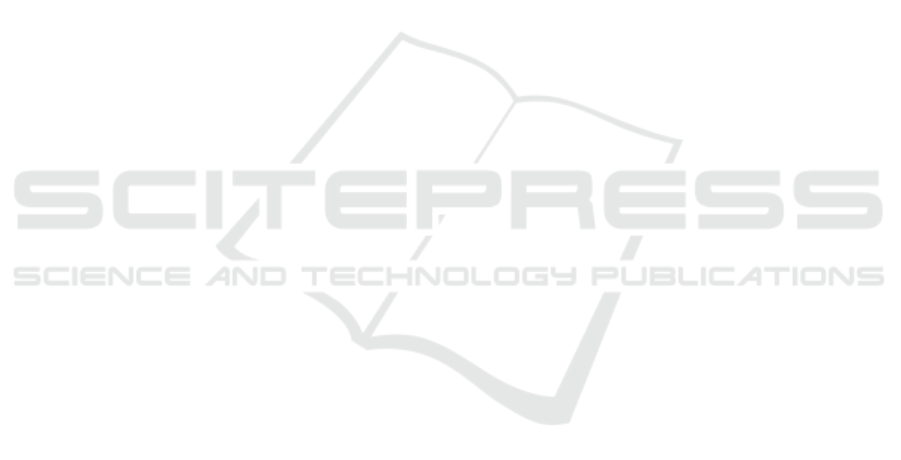
4.2.3 Discussion
In Tables 2 to 5, which are the experimental results,
we evaluate and discuss the accuracy of the learned
traffic signals. Table 2 shows that, compared to the
stable control of FTC, the learned traffic lights, DTC,
reduced waiting time for vehicle by about 74% and in-
creased vehicle waiting time for pedestrians by about
196%, respectively, on average at each density com-
pared to FTC. Based on the wait times on both sides,
DTC’s overall wait time total is up. However, Table
4 and Table 5 shows that, compared to FTC, DTC,
reduced the total waiting time of per vehicle and per
pedestrians by about 12%. This can be thought of
as a reduction in the amount of waiting time incurred
by vehicles and pedestrians in the transportation net-
work as a whole. Table 3 shows that for the two traffic
signals trained, the waiting time for LTC is shorter,
but Table 2 shows that the waiting time of vehicles
at LTC is significantly higher than that at DTC. This
is due to the fact that LTC has placed more empha-
sis on reducing pedestrian waiting time. From Sec-
tion3.4, the importance of the walker in the environ-
ment is adjusted by setting a constant α in the reward
function. This high value the pedestrian learns that
continuing to take actions that reduce the pedestrian’s
waiting time is a simple way to increase the value of
the reward. Therefore, it is necessary to design re-
wards that reduce waiting time for both pedestrians
and vehicles. It can also be inferred that the presence
of the prepared road environment was a factor that in-
creased the overall waiting time including vehicles.
Since the prepared environment is only a crossroad, it
can be said that it is easy to grasp the scale of vehicles
and pedestrians moving in the road. In such an envi-
ronment, it can be judged that DTC, which uses the
current state of the environment, is easier to perform
control that reflects the state of the environment. On
the other hand, LTC can lead to better control in com-
plex road environments where it is difficult to judge
traffic conditions on the spot. Tables 4 and 5 show that
DTC and FTC does not show a significant change in
waiting time per vehicle or per pedestrian with respect
to changes in traffic density. In contrast, the learned
traffic signals show a large change in vehicle wait-
ing times, but no noticeable difference in pedestrian
waiting times. This indicates that the learned traffic
signals work to reduce the waiting time for pedestri-
ans in response to changes in the traffic environment.
Therefore, it can be determined that the learned traffic
signals are superior in terms of control adapted to the
traffic environment.
5 CONCLUSION
The signal condition was verified by using a network
that uses the state of the environment between one
step and a network that uses the state of the environ-
ment between several steps. In this research, traffic
control is performed using traffic signals learned by
deep reinforcement learning in an environment with a
mixture of vehicles and pedestrians. As a result, DTC,
a learned traffic light, led to a reduction in the wait-
ing time experienced by each of the traffic network
as a whole. Furthermore, LTC was not possible to
reduce the waiting time of the entire traffic network,
but it was possible to reduce the pedestrian waiting
time by adapting to changes in traffic volume. In the
future, we will expand the learning and experimental
environment to create a traffic signal system that can
control traffic in a large-scale traffic network. In addi-
tion, we will improve the control of traffic signals us-
ing time series information. We will try to control ap-
propriately by increasing or decreasing the number of
states obtained from the environment and by adjusting
the past states used to select actions and adjusting the
past states used to select actions. On top of that, we
try to control not only simple structures such as cross-
roads, but also complex road environments by using
human flow, such as the size of pedestrian groups and
the direction of movement.
ACKNOWLEDGEMENTS
This work was supported by JSPS KAKENHI Grant
Numbers JP21H03496, JP22K12157.
REFERENCES
Behrisch, M., Lopez, P. A., Bieker-Walz, L., Erdmann, J.,
Flotterrod, Y., Hilbrich, R., Lucken, L., Rummel, J.,
Wagner, P., and WieBner, E. (2018). Microscopic
Traffic Simulation using SUMO. IEEE Intelligent
Transportation Systems Conference (ITSC).
Choe, C., S.Back, Woon, B., and Kong, S. (2018). Deep
Q Learing with LSTM for Traffic Light Control.
2018 24th Asia-Pacific Conference on Communica-
tions(APCC).
Ge, H., Y.Song, Wu, C., Ren, J., and Tan, G. (2019). Co-
operative Deep Q-Learning With Q-Value Transfer for
Multi Intersection Signal Control. 2019 IEEE Access
2907618.
Gers, F. A., Schmidhuber, J., and Cummins, F. (2000).
Learning to forget: continual prediction with LSTM.
Neural Computation 12(10), 2451-2471.
ICAART 2023 - 15th International Conference on Agents and Artificial Intelligence
324