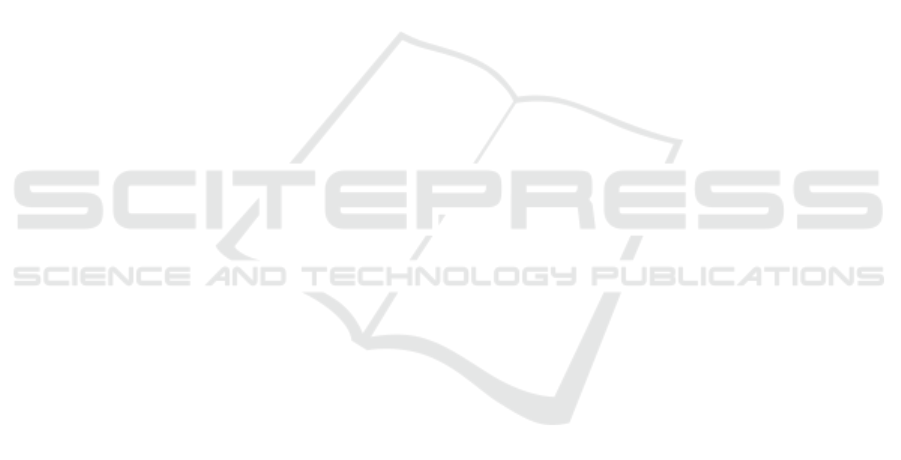
29th IEEE International Conference on Image Pro-
cessing (IEEE ICPR). IEEE.
Khairallah, M. Z., Bonardi, F., Roussel, D., and Bouchafa,
S. (2022b). Pca event-based optical flow: A fast and
accurate 2d motion estimation. In 2022 The 29th IEEE
International Conference on Image Processing (IEEE
ICIP). IEEE.
Kim, H., Handa, A., Benosman, R., Ieng, S.-H., and Davi-
son, A. J. (2008). Simultaneous mosaicing and track-
ing with an event camera. J. Solid State Circ, 43:566–
576.
Kim, H., Leutenegger, S., and Davison, A. J. (2016). Real-
time 3d reconstruction and 6-dof tracking with an
event camera. In European Conference on Computer
Vision, pages 349–364. Springer.
Kueng, B., Mueggler, E., Gallego, G., and Scaramuzza,
D. (2016). Low-latency visual odometry using event-
based feature tracks. In 2016 IEEE/RSJ International
Conference on Intelligent Robots and Systems (IROS),
pages 16–23. IEEE.
Le Gentil, C., Tschopp, F., Alzugaray, I., Vidal-Calleja, T.,
Siegwart, R., and Nieto, J. (2020). Idol: A framework
for imu-dvs odometry using lines. In 2020 IEEE/RSJ
International Conference on Intelligent Robots and
Systems (IROS), pages 5863–5870. IEEE.
Liu, M.-y., Wang, Y., and Guo, L. (2017). 6-dof motion
estimation using optical flow based on dual cameras.
Journal of Central South University, 24(2):459–466.
Longuet-Higgins, H. C. and Prazdny, K. (1980). The in-
terpretation of a moving retinal image. Proceedings
of the Royal Society of London. Series B. Biological
Sciences, 208(1173):385–397.
Lupton, T. and Sukkarieh, S. (2011). Visual-inertial-aided
navigation for high-dynamic motion in built environ-
ments without initial conditions. IEEE Transactions
on Robotics, 28(1):61–76.
Mueggler, E., Gallego, G., Rebecq, H., and Scaramuzza,
D. (2018). Continuous-time visual-inertial odometry
for event cameras. IEEE Transactions on Robotics,
34(6):1425–1440.
Mueggler, E., Huber, B., and Scaramuzza, D. (2014).
Event-based, 6-dof pose tracking for high-speed ma-
neuvers. In 2014 IEEE/RSJ International Conference
on Intelligent Robots and Systems, pages 2761–2768.
IEEE.
Mueggler, E., Rebecq, H., Gallego, G., Delbruck, T., and
Scaramuzza, D. (2017). The event-camera dataset and
simulator: Event-based data for pose estimation, vi-
sual odometry, and slam. The International Journal of
Robotics Research, 36(2):142–149.
Muglikar, M., Gehrig, M., Gehrig, D., and Scaramuzza, D.
(2021). How to calibrate your event camera. 2021
IEEE/CVF Conference on Computer Vision and Pat-
tern Recognition Workshops (CVPRW), pages 1403–
1409.
Rebecq, H., Gallego, G., Mueggler, E., and Scaramuzza, D.
(2018). EMVS: Event-based multi-view stereo—3D
reconstruction with an event camera in real-time. Int.
J. Comput. Vis., 126:1394–1414.
Rebecq, H., Horstschaefer, T., Gallego, G., and Scara-
muzza, D. (2017a). EVO: A geometric approach to
event-based 6-DOF parallel tracking and mapping in
real time. IEEE Robotics and Automation Letters,
2(2):593–600.
Rebecq, H., Horstschaefer, T., and Scaramuzza, D. (2017b).
Real-time visual-inertial odometry for event cameras
using keyframe-based nonlinear optimization. In
BMVC.
Soliman, A., Bonardi, F., Sidib
´
e, D., and Bouchafa, S.
(2022). IBISCape: A simulated benchmark for multi-
modal SLAM systems evaluation in large-scale dy-
namic environments. Journal of Intelligent & Robotic
Systems, 106(3):53.
Vidal, A. R., Rebecq, H., Horstschaefer, T., and Scara-
muzza, D. (2018). Ultimate slam? combining events,
images, and imu for robust visual slam in hdr and
high-speed scenarios. IEEE Robotics and Automation
Letters, 3(2):994–1001.
Weikersdorfer, D., Adrian, D. B., Cremers, D., and Con-
radt, J. (2014). Event-based 3d slam with a depth-
augmented dynamic vision sensor. In 2014 IEEE
international conference on robotics and automation
(ICRA), pages 359–364. IEEE.
Weikersdorfer, D. and Conradt, J. (2012). Event-based par-
ticle filtering for robot self-localization. In 2012 IEEE
International Conference on Robotics and Biomimet-
ics (ROBIO), pages 866–870. IEEE.
Weikersdorfer, D., Hoffmann, R., and Conradt, J. (2013).
Simultaneous localization and mapping for event-
based vision systems. In International Conference on
Computer Vision Systems, pages 133–142. Springer.
Zhou, Y., Gallego, G., and Shen, S. (2021). Event-
based stereo visual odometry. IEEE Transactions on
Robotics, 37(5):1433–1450.
Zihao Zhu, A., Atanasov, N., and Daniilidis, K. (2017).
Event-based visual inertial odometry. In Proceedings
of the IEEE Conference on Computer Vision and Pat-
tern Recognition, pages 5391–5399.
Zucchelli, M. (2002). Optical flow based structure from
motion. PhD thesis, Numerisk analys och datalogi.
Zucchelli, M., Santos-Victor, J., and Christensen, H. I.
(2002). Multiple plane segmentation using optical
flow. In BMVC, volume 2, pages 313–322.
Flow-Based Visual-Inertial Odometry for Neuromorphic Vision Sensors Using non-Linear Optimization with Online Calibration
973