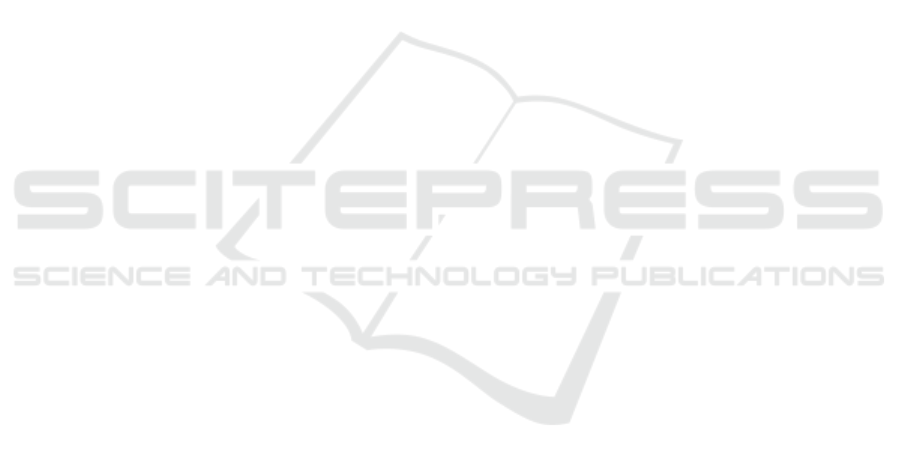
Ballistocardiography and Seismocardiography: A Re-
view of Recent Advances. IEEE Journal of Biomedi-
cal and Health Informatics, 19(4):1414–1427.
Javaid, A. Q., Ashouri, H., Tridandapani, S., and Inan, O. T.
(2016). Elucidating the hemodynamic origin of bal-
listocardiographic forces: Toward improved monitor-
ing of cardiovascular health at home. IEEE Journal
of Translational Engineering in Health and Medicine,
4:1–8.
Jianbo Shi and Tomasi (1994). Good features to track. In
Proceedings of IEEE Conference on Computer Vision
and Pattern Recognition CVPR-94, pages 593–600.
IEEE Comput. Soc. Press.
Jung, H., Kimball, J., Receveur, T., Agdeppa, E., and
Inan, O. T. (2020). Quantification of Posture-Induced
Changes in Bed-Based Ballistocardiogram. Comput-
ing in Cardiology, 2020-Septe:2020–2023.
Kazemi, V. and Sullivan, J. (2014). One millisecond face
alignment with an ensemble of regression trees. Pro-
ceedings of the IEEE Computer Society Conference
on Computer Vision and Pattern Recognition, pages
1867–1874.
L
´
azaro, J., Gil, E., Vergara, J. M., and Laguna, P. (2013).
Pulse rate variability analysis for discrimination of
sleep-apnea-related decreases in the amplitude fluctu-
ations of pulse photoplethysmographic signal in chil-
dren. IEEE journal of biomedical and health infor-
matics, 18(1):240–246.
Lee, H., Cho, A., and Whang, M. (2021). Fusion Method
to Estimate Heart Rate from Facial Videos Based on
RPPG and RBCG. Sensors, 21(20):6764.
Leit
˜
ao, F., Moreira, E., Alves, F., Lourenc¸o, M., Azevedo,
O., Gaspar, J., and Rocha, L. A. (2018). High-
resolution seismocardiogram acquisition and analysis
system. Sensors, 18(10):3441.
Li, F., Zhao, Y., Kong, L., Dong, L., Liu, M., Hui, M., and
Liu, X. (2020). A camera-based ballistocardiogram
heart rate measurement method. Review of Scientific
Instruments, 91(5).
Moc¸o, A. and Verkruysse, W. (2021). Pulse oximetry based
on photoplethysmography imaging with red and green
light. Journal of Clinical Monitoring and Computing,
35(1):123–133.
Molinaro, N., Schena, E., Silvestri, S., Bonotti, F., Aguzzi,
D., Viola, E., Buccolini, F., and Massaroni, C. (2022).
Contactless Vital Signs Monitoring From Videos
Recorded With Digital Cameras: An Overview. Fron-
tiers in Physiology, 13:160.
Pan, J. and Tompkins, W. J. (1985). A real-time qrs de-
tection algorithm. IEEE transactions on biomedical
engineering, (3):230–236.
Pielmus, A.-G., M
¨
uhlstef, J., Bresch, E., Glos, M., Jun-
gen, C., Mieke, S., Orglmeister, R., Schulze, A., Sten-
der, B., Voigt, V., and Zaunseder, S. (2021). Sur-
rogate based continuous noninvasive blood pressure
measurement. Biomedical Engineering / Biomedi-
zinische Technik, 66(3):231–245.
Pinheiro, E., Postolache, O., and Gir
˜
ao, P. (2010). Theory
and Developments in an Unobtrusive Cardiovascular
System Representation: Ballistocardiography. The
Open Biomedical Engineering Journal, 4(1):201–216.
Rasche, S., Huhle, R., Junghans, E., de Abreu, M. G., Ling,
Y., Trumpp, A., and Zaunseder, S. (2020). Associ-
ation of remote imaging photoplethysmography and
cutaneous perfusion in volunteers. Scientific Reports,
10(1):16464.
Sadek, I. (2018). Ballistocardiogram Signal Processing: A
Literature Review. arXiv preprint arXiv:1807.00951.
Shao, D., Tsow, F., Liu, C., Yang, Y., and Tao, N. (2017).
Simultaneous Monitoring of Ballistocardiogram and
Photoplethysmogram Using a Camera. IEEE Transac-
tions on Biomedical Engineering, 64(5):1003–1010.
Shin, S., Mousavi, A., Lyle, S., Jang, E., Yousefian, P.,
Mukkamala, R., Jang, D. G., Kwon, U. K., Kim, Y. H.,
and Hahn, J. O. (2022). Posture-Dependent Variabil-
ity in Wrist Ballistocardiogram-Photoplethysmogram
Pulse Transit Time: Implication to Cuff-Less Blood
Pressure Tracking. IEEE Transactions on Biomedical
Engineering, 69(1):347–355.
Soames, R. W. and Atha, J. (1982). Three-dimensional
ballistocardiographic responses to changes of pos-
ture. Clinical Physics and Physiological Measure-
ment, 3(3):169–177.
Starr, I. (1958). The relation of the ballistocardiogram to
cardiac function. The American Journal of Cardiol-
ogy, 2(6):737–747.
Steinman, J., Barszczyk, A., Sun, H.-S., Lee, K., and Feng,
Z.-P. (2021). Smartphones and Video Cameras: Fu-
ture Methods for Blood Pressure Measurement. Fron-
tiers in Digital Health, 3.
Su, B. Y., Enayati, M., Ho, K. C., Skubic, M., Despins, L.,
Keller, J., Popescu, M., Guidoboni, G., and Rantz, M.
(2019). Monitoring the relative blood pressure using
a hydraulic bed sensor system. IEEE Transactions on
Biomedical Engineering, 66(3):740–748.
Tomasi, C. and Kanade, T. (1991). Detection and Tracking
of Point Features. Technical report, Carnegie Mellon
University, Pittsburgh, PA.
van Gastel, M., Stuijk, S., and de Haan, G. (2016). Robust
respiration detection from remote photoplethysmog-
raphy. Biomedical Optics Express, 7(12):4941.
Viola, P. and Jones, M. (2001). Rapid object detection us-
ing a boosted cascade of simple features. In Proceed-
ings of the 2001 IEEE Computer Society Conference
on Computer Vision and Pattern Recognition. CVPR
2001, volume 1, pages I–511–I–518. IEEE Comput.
Soc.
Zaunseder, S. and Rasche, S. (2022). Chapter 7 - Clin-
ical applications for imaging photoplethysmography.
In Wang, W. and Wang, X., editors, Contactless Vital
Signs Monitoring, pages 149–164. Academic Press.
Zaunseder, S., Trumpp, A., Wedekind, D., and Malberg,
H. (2018). Cardiovascular assessment by imag-
ing photoplethysmography-a review. Biomedizinische
Technik, 63(5):529–535.
Zhang, Y., Zhang, X., Cui, P., Li, S., and Tang, J. (2021).
Key Feature Selection and Model Analysis for Blood
Pressure Estimation from Electrocardiogram, Ballis-
BIOSIGNALS 2023 - 16th International Conference on Bio-inspired Systems and Signal Processing
64