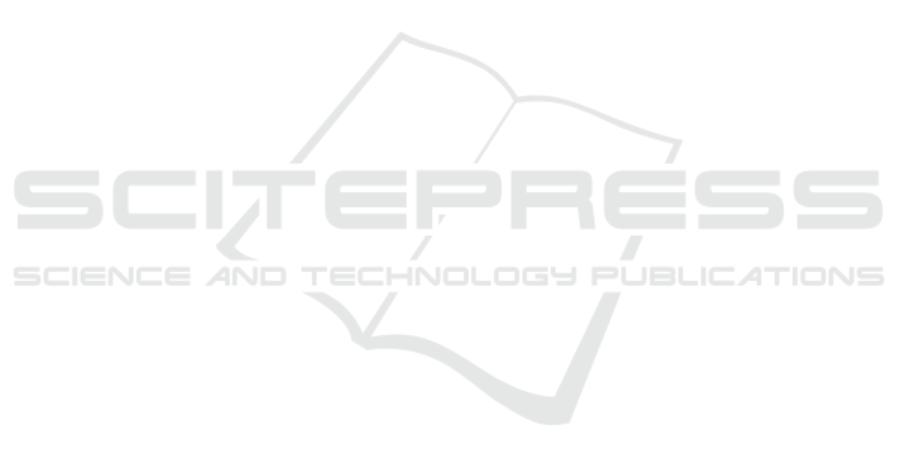
database focusing on valence and normative signifi-
cance. Behav Res Methods, 43(2):468–477.
Dincelli, E. and Yayla, A. (2022). Immersive virtual re-
ality in the age of the metaverse: A hybrid-narrative
review based on the technology affordance perspec-
tive. The Journal of Strategic Information Systems,
31(2):101717. 2021 Review Issue.
Duan, R.-N., Zhu, J.-Y., and Lu, B.-L. (2013). Differential
entropy feature for eeg-based emotion classification.
In 2013 6th International IEEE/EMBS Conference on
Neural Engineering (NER), pages 81–84.
Feldman Barrett, L. and Russell, J. A. (1998). Indepen-
dence and bipolarity in the structure of current af-
fect. Journal of Personality and Social Psychology,
74:967–984.
Gao, Q., Wang, C.-h., Wang, Z., Song, X.-l., Dong, E.-z.,
and Song, Y. (2020). Eeg based emotion recognition
using fusion feature extraction method. Multimedia
Tools and Applications, 79(37):27057–27074.
G
´
omez-Ca
˜
n
´
on, J. S., Guti
´
errez-P
´
aez, N., Porcaro, L.,
Porter, A., Cano, E., Herrera-Boyer, P., Gkiokas, A.,
Santos, P., Hern
´
andez-Leo, D., Karreman, C., and
G
´
omez, E. (2022). Trompa-mer: an open dataset for
personalized music emotion recognition. Journal of
Intelligent Information Systems.
Guti
´
errez-Maldonado, J., Rus-Calafell, M., and Gonz
´
alez-
Conde, J. (2014). Creation of a new set of dynamic
virtual reality faces for the assessment and training
of facial emotion recognition ability. Virtual Reality,
18(1):61–71.
Hosseini, S. A., Khalilzadeh, M. A., Naghibi-Sistani, M. B.,
and Homam, S. M. (2015). Emotional stress recog-
nition using a new fusion link between electroen-
cephalogram and peripheral signals. Iran J Neurol,
14(3):142–151.
Katsigiannis, S. and Ramzan, N. (2018). Dreamer: A
database for emotion recognition through eeg and ecg
signals from wireless low-cost off-the-shelf devices.
IEEE Journal of Biomedical and Health Informatics,
22(1):98–107.
Ko, J., Jang, S.-W., Lee, H. T., Yun, H.-K., and Kim, Y. S.
(2020). Effects of virtual reality and non-virtual real-
ity exercises on the exercise capacity and concentra-
tion of users in a ski exergame: Comparative study.
JMIR Serious Games, 8(4):e16693.
Koelstra, S., Muhl, C., Soleymani, M., Lee, J.-S., Yazdani,
A., Ebrahimi, T., Pun, T., Nijholt, A., and Patras, I.
(2012). Deap: A database for emotion analysis ;using
physiological signals. IEEE Transactions on Affective
Computing, 3(1):18–31.
Kurdi, B., Lozano, S., and Banaji, M. R. (2017). Introduc-
ing the open affective standardized image set (oasis).
Behav Res Methods, 49(2):457–470.
Lausen, A. and Hammerschmidt, K. (2020). Emotion
recognition and confidence ratings predicted by vocal
stimulus type and prosodic parameters. Humanities
and Social Sciences Communications, 7(1):2.
Liu, H., Zhang, Y., Li, Y., and Kong, X. (2021). Review
on emotion recognition based on electroencephalog-
raphy. Frontiers in Computational Neuroscience, 15.
Maithri, M., Raghavendra, U., Gudigar, A., Samanth,
J., Datta Barua, P., Murugappan, M., Chakole, Y.,
and Acharya, U. R. (2022). Automated emotion
recognition: Current trends and future perspectives.
Computer Methods and Programs in Biomedicine,
215:106646.
Marchewka, A.,
˙
Zurawski, Ł., Jednor
´
og, K., and
Grabowska, A. (2014). The nencki affective picture
system (naps): Introduction to a novel, standardized,
wide-range, high-quality, realistic picture database.
Behavior Research Methods, 46(2):596–610.
Marcus, A., editor (2014). Can Virtual Reality Increase
Emotional Responses (Arousal and Valence)? A Pi-
lot Study, Cham. Springer International Publishing.
Martinek, R., Ladrova, M., Sidikova, M., Jaros, R., Be-
hbehani, K., Kahankova, R., and Kawala-Sterniuk,
A. (2021). Advanced bioelectrical signal processing
methods: Past, present and future approach-part ii:
Brain signals. Sensors (Basel), 21(19).
Meuleman, B. and Rudrauf, D. (2021). Induction and profil-
ing of strong multi-componential emotions in virtual
reality. IEEE Transactions on Affective Computing,
12(1):189–202.
Mohammadi, G. and Vuilleumier, P. (2022). A multi-
componential approach to emotion recognition and
the effect of personality. IEEE Transactions on Af-
fective Computing, 13(3):1127–1139.
Namazi, H., Aghasian, E., and Ala, T. S. (2020).
Complexity-based classification of eeg signal in nor-
mal subjects and patients with epilepsy. Technology
and Health Care, 28(1):57–66.
Pan, Z., Cheok, A., Haller, M., Lau, R. W. H., Saito, H., and
Liang, R., editors (2006). Emotion Recognition Using
Physiological Signals, Berlin, Heidelberg. Springer
Berlin Heidelberg.
Parong, J., Pollard, K. A., Files, B. T., Oiknine, A. H., Sina-
tra, A. M., Moss, J. D., Passaro, A., and Khooshabeh,
P. (2020). The mediating role of presence differs
across types of spatial learning in immersive technolo-
gies. Computers in Human Behavior, 107:106290.
Ribeiro, F. S., Santos, F. H., Albuquerque, P. B., and
Oliveira-Silva, P. (2019). Emotional induction
through music: Measuring cardiac and electrodermal
responses of emotional states and their persistence.
Front Psychol, 10:451.
Riegel, M.,
˙
Zurawski, Ł., Wierzba, M., Moslehi, A., Klo-
cek, Ł., Horvat, M., Grabowska, A., Michałowski, J.,
Jednor
´
og, K., and Marchewka, A. (2016). Character-
ization of the nencki affective picture system by dis-
crete emotional categories (naps be). Behavior Re-
search Methods, 48(2):600–612.
Riva, G., Mantovani, F., Capideville, C. S., Preziosa, A.,
Morganti, F., Villani, D., Gaggioli, A., Botella, C., and
Alca
˜
niz, M. (2007). Affective interactions using vir-
tual reality: the link between presence and emotions.
Cyberpsychol Behav, 10(1):45–56.
Roy, Y., Banville, H., Albuquerque, I., Gramfort, A., Falk,
T. H., and Faubert, J. (2019). Deep learning-based
electroencephalography analysis: a systematic review.
Journal of neural engineering, 16(5):051001.
Experimental Setup and Protocol for Creating an EEG-signal Database for Emotion Analysis Using Virtual Reality Scenarios
85