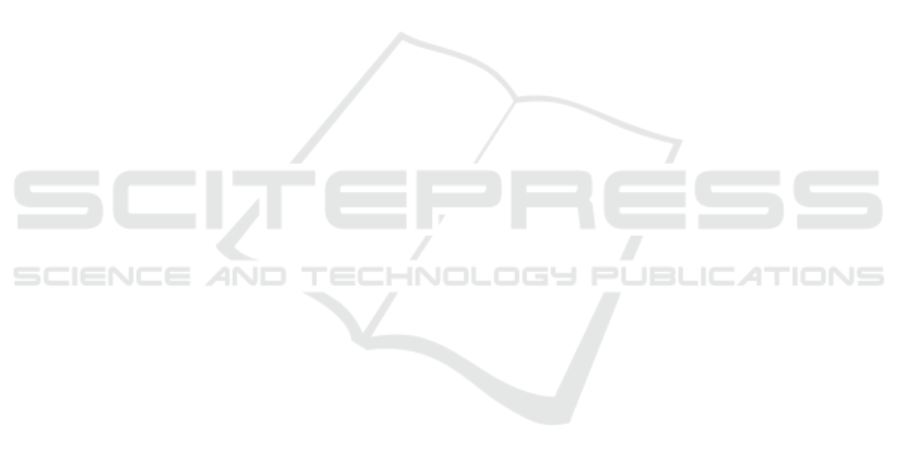
REFERENCES
Agbley, B. L. Y., Li, J., Haq, A. U., Cobbinah, B.,
Kulevome, D. K. B., Agbefu, P. A., and Eleeza, B.
(2020). Wavelet-based cough signal decomposition
for multimodal classification. 2020 ICCWAMTIP,
pages 5–9.
Casiraghi, E., Malchiodi, D., Trucco, G., Frasca, M., Cap-
pelletti, L., Fontana, T., Esposito, A. A., Avola, E.,
Jachetti, A., Reese, J., Rizzi, A., Robinson, P. N.,
and Valentini, G. (2020). Explainable machine learn-
ing for early assessment of covid-19 risk prediction
in emergency departments. IEEE Access, 8:196299–
196325.
Cortes, C. and Vapnik, V. (1995). Support-vector networks.
Machine Learning, 20(3):273–297.
Cozzatti, M., Simonetta, F., and Ntalampiras, S. (2022).
Variational autoencoders for anomaly detection in res-
piratory sounds. In Lecture Notes in Computer Sci-
ence, pages 333–345. Springer Nature Switzerland.
Dietterich, T. G. (2000). Ensemble methods in machine
learning. In Multiple Classifier Systems, LBCS-1857,
pages 1–15. Springer.
Erdo
˘
gan, Y. E. and Narin, A. (2021). COVID-19 de-
tection with traditional and deep features on cough
acoustic signals. Computers in Biology and Medicine,
136:104765.
European Commission (2020). White paper on artificial
intelligence: a european approach to excellence and
trust. Technical report, Brussels.
Fakhry, A., Jiang, X., Xiao, J., Chaudhari, G., Han, A., and
Khanzada, A. (2021). Virufy: A multi-branch deep
learning network for automated detection of COVID-
19. CoRR, abs/2103.01806.
Geurts, P., Ernst, D., and Wehenkel, L. (2006). Extremely
randomized trees. Machine Learning, 63(1):3–42.
Ho, T. K. (1998). The random subspace method for con-
structing decision forests. IEEE Transactions on
PAMI, 20(8):832–844.
Imran, A., Posokhova, I., Qureshi, H. N., Masood, U., Riaz,
M. S., Ali, K., John, C. N., Hussain, M. I., and Nabeel,
M. (2020). AI4covid-19: AI enabled preliminary di-
agnosis for COVID-19 from cough samples via an
app. Informatics in Medicine Unlocked, 20:100378.
Ke, G., Meng, Q., Finley, T., Wang, T., Chen, W., Ma, W.,
Ye, Q., and Liu, T.-Y. (2017). Lightgbm: A highly ef-
ficient gradient boosting decision tree. NIPS’17, page
3149–3157, Red Hook, NY, USA. Curran Associates
Inc.
Lippi, G., Mattiuzzi, C., Bovo, C., and Plebani, M. (2020).
Current laboratory diagnostics of coronavirus disease
2019 (covid-19). Acta Bio Medica Atenei Parmensis,
91(2):137–145.
Nessiem, M. A., Mohamed, M. M., Coppock, H., Gaskell,
A., and Schuller, B. W. (2021). Detecting covid-19
from breathing and coughing sounds using deep neu-
ral networks. In 2021 IEEE CBMS, pages 183–188.
Ning, W., Lei, S., Yang, J., Cao, Y., Jiang, P., Yang, Q.,
Zhang, J., Wang, X., Chen, F., Geng, Z., Xiong, L.,
Zhou, H., Guo, Y., Zeng, Y., Shi, H., Wang, L., Xue,
Y., and Wang, Z. (2020). Open resource of clinical
data from patients with pneumonia for the prediction
of COVID-19 outcomes via deep learning. Nature
Biomedical Engineering, 4(12):1197–1207.
Ntalampiras, S. (2020a). Collaborative framework for auto-
matic classification of respiratory sounds. IET Signal
Processing, 14(4):223–228.
Ntalampiras, S. (2020b). Identification of anomalous
phonocardiograms based on universal probabilistic
modeling. IEEE Letters of the Computer Society,
3(2):50–53.
Ntalampiras, S. and Potamitis, I. (2019). Classification of
sounds indicative of respiratory diseases. In Engineer-
ing Applications of Neural Networks, pages 93–103.
Springer International Publishing.
Orlandic, L., Teijeiro, T., and Atienza, D. (2021). The
COUGHVID crowdsourcing dataset, a corpus for the
study of large-scale cough analysis algorithms. Scien-
tific Data, 8(1):3–2.
Poir
`
e, A. M., Simonetta, F., and Ntalampiras, S. (2022).
Deep feature learning for medical acoustics. In
Lecture Notes in Computer Science, pages 39–50.
Springer Nature Switzerland.
Rao, S., Narayanaswamy, V., Esposito, M., Thiagarajan, J.,
and Spanias, A. (2021). Deep learning with hyper-
parameter tuning for covid-19 cough detection. In
2021 IISA, pages 1–5.
Rumelhart, D. E., Hinton, G. E., and Williams, R. J. (1986).
Learning Representations by Back-propagating Er-
rors. Nature, 323(6088):533–536.
Tena, A., Clari
`
a, F., and Solsona, F. (2022). Automated de-
tection of COVID-19 cough. Biomedical Signal Pro-
cessing and Control, 71:103175.
Xia, T., Spathis, D., Brown, C., Ch, J., Grammenos, A.,
Han, J., Hasthanasombat, A., Bondareva, E., Dang, T.,
Floto, A., Cicuta, P., and Mascolo, C. (2021). COVID-
19 sounds: A large-scale audio dataset for digital
COVID-19 detection. In Thirty-fifth Conference on
Neural Information Processing Systems Datasets and
Benchmarks Track (Round 2).
Ensemble Learning for Cough-Based Subject-Independent COVID-19 Detection
805