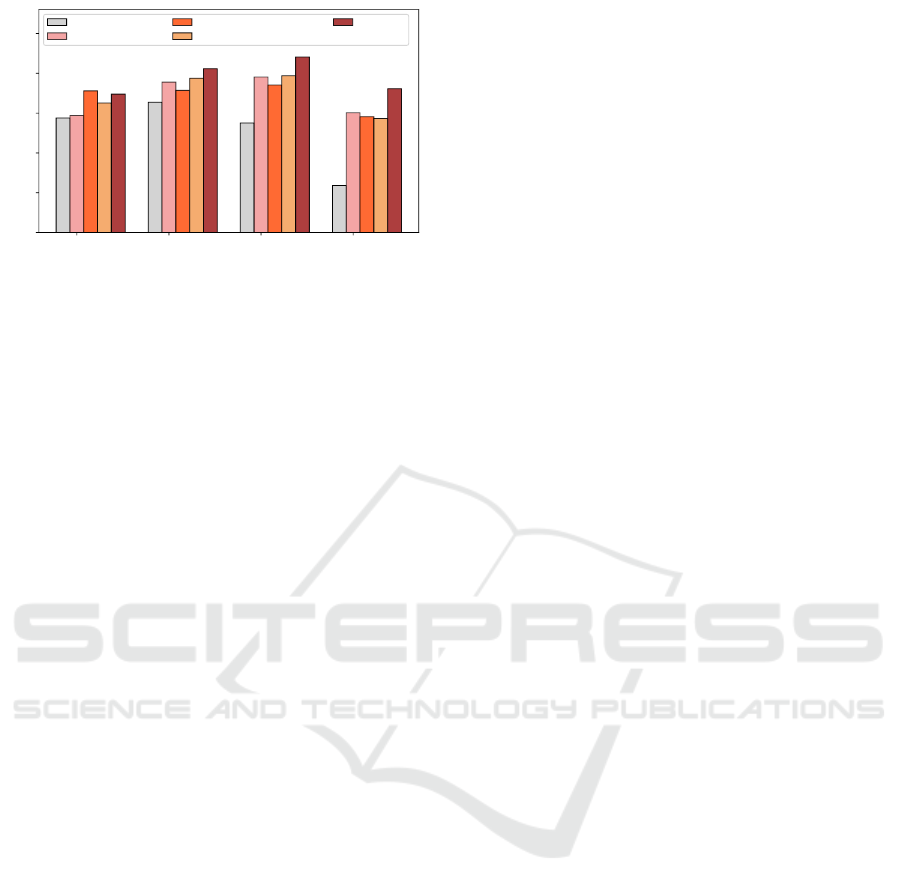
0-200
201-500
501-1000
>1000
Protein Sequence Length Range ---->
30
40
50
60
70
80
Avg. F1-Scores ---->
MLDA
ProtVecGen-Plus
ProtVecGen-Ensemble
ProtVecGen-Plus+MLDA
Proposed
Figure 4: Molecular Function: Length-wise performances
of protein sequences.
4 CONCLUSION
In this work, a sub-sequence based method for pro-
tein function prediction is introduced. The proposed
method takes benefits from information collected for
multiple sequence motifs – captured using the CNN
network – to determine the function for each sub-
sequence. Later, the functional inference for sub-
sequences are used to facilitate the functional annota-
tion of full-length protein sequence. Overall, the pro-
posed method showed great potential, especially for
long protein sequences. The research focused on pro-
tein sub-sequence is still an open research area, and
remarkably, can be great asset to improve the protein
studies. Future work will focus on merging additional
features and putting different deep learning models to
the test.
REFERENCES
Ashburner, M., Ball, C. A., Blake, J. A., Botstein, D.,
Butler, H., Cherry, J. M., Davis, A. P., Dolinski, K.,
Dwight, S. S., Eppig, J. T., et al. (2000). Gene ontol-
ogy: tool for the unification of biology. Nature genet-
ics, 25(1):25–29.
Cao, R., Freitas, C., Chan, L., Sun, M., Jiang, H., and Chen,
Z. (2017). Prolango: protein function prediction using
neural machine translation based on a recurrent neural
network. Molecules, 22(10):1732.
Consortium, U. (2015). Uniprot: a hub for protein informa-
tion. Nucleic acids research, 43(D1):D204–D212.
Fa, R., Cozzetto, D., Wan, C., and Jones, D. T. (2018). Pre-
dicting human protein function with multi-task deep
neural networks. PloS one, 13(6):e0198216.
Gligorijevi
´
c, V., Renfrew, P. D., Kosciolek, T., Leman,
J. K., Berenberg, D., Vatanen, T., Chandler, C.,
Taylor, B. C., Fisk, I. M., Vlamakis, H., et al.
(2021). Structure-based protein function prediction
using graph convolutional networks. Nature commu-
nications, 12(1):1–14.
Ioffe, S. and Szegedy, C. (2015). Batch normalization: Ac-
celerating deep network training by reducing internal
covariate shift. In International conference on ma-
chine learning, pages 448–456. PMLR.
Jiang, Y., Oron, T. R., Clark, W. T., Bankapur, A. R.,
D’Andrea, D., Lepore, R., Funk, C. S., Kahanda, I.,
Verspoor, K. M., Ben-Hur, A., et al. (2016). An
expanded evaluation of protein function prediction
methods shows an improvement in accuracy. Genome
biology, 17(1):1–19.
Kingma, D. P. and Ba, J. (2014). Adam: A
method for stochastic optimization. arXiv preprint
arXiv:1412.6980.
Kulmanov, M. and Hoehndorf, R. (2020). Deepgoplus:
improved protein function prediction from sequence.
Bioinformatics, 36(2):422–429.
Kulmanov, M., Khan, M. A., and Hoehndorf, R. (2018).
Deepgo: predicting protein functions from sequence
and interactions using a deep ontology-aware classi-
fier. Bioinformatics, 34(4):660–668.
Kumari, D., Ranjan, A., and Deepak, A. (2019). Pro-
tein function prediction: Combining statistical fea-
tures with deep learning. In Proceedings of 2nd In-
ternational Conference on Advanced Computing and
Software Engineering (ICACSE).
Maas, A. L., Hannun, A. Y., Ng, A. Y., et al. (2013). Rec-
tifier nonlinearities improve neural network acoustic
models. In Proc. icml, volume 30, page 3. Citeseer.
Makrodimitris, S., van Ham, R. C., and Reinders, M. J.
(2019). Improving protein function prediction using
protein sequence and go-term similarities. Bioinfor-
matics, 35(7):1116–1124.
¨
Ozt
¨
urk, H.,
¨
Ozg
¨
ur, A., and Ozkirimli, E. (2018). Deepdta:
deep drug–target binding affinity prediction. Bioinfor-
matics, 34(17):i821–i829.
¨
Ozt
¨
urk, H., Ozkirimli, E., and
¨
Ozg
¨
ur, A. (2019). Wid-
edta: prediction of drug-target binding affinity. arXiv
preprint arXiv:1902.04166.
Radivojac, P., Clark, W. T., Oron, T. R., Schnoes, A. M.,
Wittkop, T., Sokolov, A., Graim, K., Funk, C., Ver-
spoor, K., Ben-Hur, A., et al. (2013). A large-scale
evaluation of computational protein function predic-
tion. Nature methods, 10(3):221–227.
Ranjan, A., Fahad, M. S., Fern
´
andez-Baca, D., Deepak, A.,
and Tripathi, S. (2019). Deep robust framework for
protein function prediction using variable-length pro-
tein sequences. IEEE/ACM Transactions on Computa-
tional Biology and Bioinformatics, 17(5):1648–1659.
Ranjan, A., Fernandez-Baca, D., Tripathi, S., and Deepak,
A. (2021). An ensemble tf-idf based approach to pro-
tein function prediction via sequence segmentation.
IEEE/ACM Transactions on Computational Biology
and Bioinformatics.
Wang, H., Yan, L., Huang, H., and Ding, C. (2016).
From protein sequence to protein function via multi-
label linear discriminant analysis. IEEE/ACM trans-
actions on computational biology and bioinformatics,
14(3):503–513.
ICAART 2023 - 15th International Conference on Agents and Artificial Intelligence
250