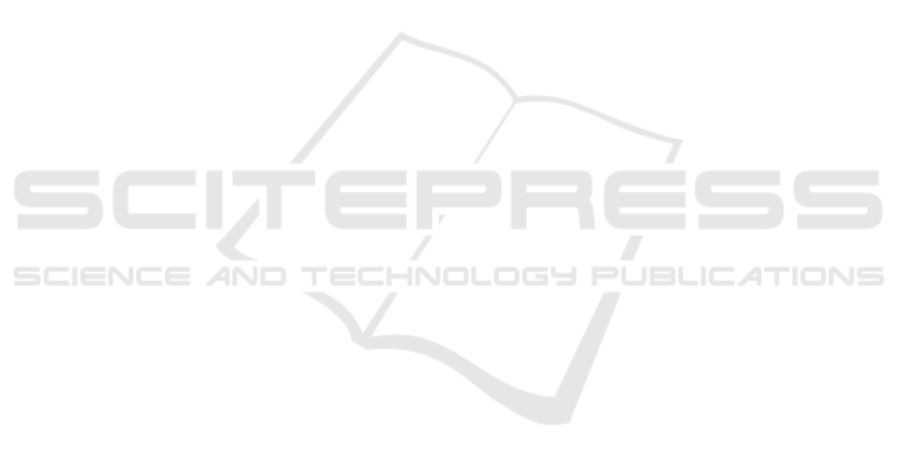
5.5 Behaviors of Active and Deactivated
Agents
We analyzed the behaviors of active and deactivated
agents by investigating the transition of K
i
. We plot-
ted, in Fig. 11, the relationship between K
i
and the
total pausing time of i in one independent trial that
was randomly selected from Exp. 3, where K
i
is the
value at 5,000,000 steps, and the total pausing time is
the sum of the pause times during the last 1,000,000
steps of the experiment. Therefore, K
i
of a deacti-
vated agent i was the value when it was deactivated,
and the total pausing time of i must be zero. Seven red
plots correspond to deactivated agents. This figure in-
dicates that deactivated agents had relatively high val-
ues of K
i
and active agents continued patrolling with
a very short pausing time. This implies that thirteen
agents were sufficient to maintain the required quality
set in this experiment.
Figures 13 and 13 present plots of the transition
of K
i
for agents that were deactivated at 250,000 and
500,000 steps (Fig. 13) and active agents (Fig. 13).
We can see from Fig. 13 that the K
i
values of these
agents rapidly increased and were then selected for
deactivation because the sum of its pausing time was
the largest at that time. Subsequently, their K
i
did
not change. Meanwhile, active agents temporally in-
creased their values of K
i
, but after that, the K
i
de-
creased and kept the relatively lower values because
some agents were deactivated. Thus, the active agents
must patrol more in their stead to fulfill the require-
ment.
Our experimental results show that the proposed
method can reduce energy consumption more than the
previous method (Wu et al., 2019) by introducing the
individual learning parameter K
i
. We further reduced
the number of operating agents based on the value of
K
i
while fulfilling the quality requirements.
6 CONCLUSIONS
Although conventional studies for MACPP usually
consider maximizing efficiency, it is often desirable
to reduce the energy used while fulfilling the qual-
ity requirement. Therefore, we proposed a method
that can save energy while meeting the requirement
in MACPP. The basic idea of our method is that each
agent individually foresees the state of the environ-
ment and assesses its own behavior from individual
viewpoints. Then, it returns to the charging base
or pauses for a while to save energy on determin-
ing that it is not necessary to start patrolling immedi-
ately. Moreover, we found that the proposed individ-
ual learning clusters the agents into two groups, and
by using these findings, we could successfully deacti-
vate redundant agents to reduce the number of work-
ing agents required for the current requirement.
This study assumed that the event probability
(EOP) p(v) in the environment is known, but this is
not always possible. Therefore, our future work is
to propose a method to reduce energy consumption
while agents learn the EOP in the environment.
ACKNOWLEDGEMENTS
This work was supported by JSPS KAKENHI Grant
Number 20H04245.
REFERENCES
Benkrid, A., Benallegue, A., and Achour, N. (2019). Multi-
robot coordination for energy-efficient exploration.
Journal of Control, Automation and Electrical Sys-
tems, 30(6):911–920.
Hattori, K. and Sugawara, T. (2021). Effective Area Parti-
tioning in a Multi-Agent Patrolling Domain for Bet-
ter Efficiency. In Proceedings of the 13th Inter-
national Conference on Agents and Artificial Intel-
ligence - Volume 1: ICAART,, pages 281–288. IN-
STICC, SciTePress.
Kim, J., Dietz, J. E., and Matson, E. T. (2016). Modeling of
a multi-robot energy saving system to increase oper-
ating time of a firefighting robot. In 2016 IEEE Symp.
on Technologies for Homeland Security (HST), pages
1–6.
Latif, E., Gui, Y., Munir, A., and Parasuraman, R. (2021).
Energy-aware multi-robot task allocation in persistent
tasks.
Ma, H., Li, J., Kumar, T. S., and Koenig, S. (2017). Life-
long multi-agent path finding for online pickup and
delivery tasks. In Proc. of the 16th Conference on Au-
tonomous Agents and MultiAgent Systems, AAMAS
’17, pages 837–845, Richland, SC. IFAAMAS.
Notomista, G., Mayya, S., Emam, Y., Kroninger, C., Bo-
hannon, A., Hutchinson, S., and Egerstedt, M. (2022).
A resilient and energy-aware task allocation frame-
work for heterogeneous multirobot systems. IEEE
Transactions on Robotics, 38(1):159–179.
Othmani-Guibourg, M., El Fallah-Seghrouchni, A., Farges,
J.-L., and Potop-Butucaru, M. (2017). Multi-agent pa-
trolling in dynamic environments. In 2017 IEEE In-
ternational Conference on Agents (ICA), pages 72–77.
IEEE.
Othmani-Guibourg, M., Fallah-Seghrouchni, A. E., and
Farges, J.-L. (2018). Decentralized multi-agent pa-
trolling strategies using global idleness estimation. In
International Conference on Principles and Practice
of Multi-Agent Systems, pages 603–611. Springer.
ICAART 2023 - 15th International Conference on Agents and Artificial Intelligence
46