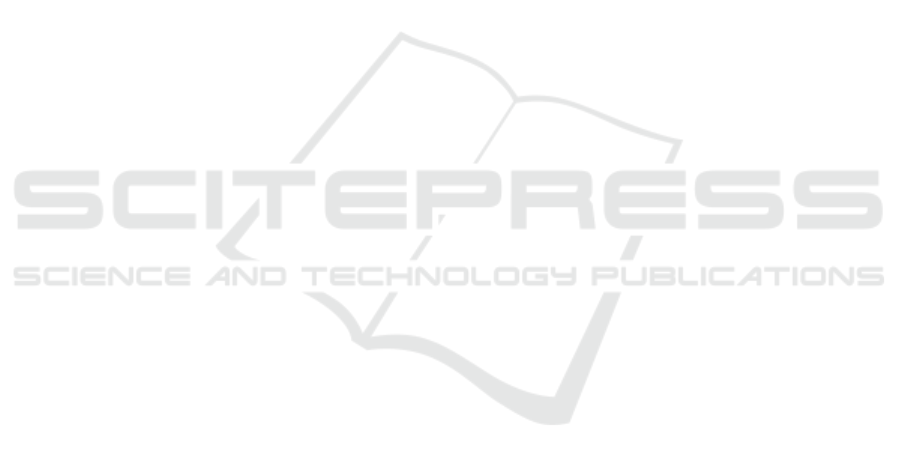
ent patient presentations together with their identified
templates and extracted regions.
The forehead tracking proceeded frame-by-frame.
Once we matched the template in an initial frame,
we automatically track the region across subsequent
frames measuring similarity mostly with no need
for human intervention. When a significant scene
change occurs such as nurse intervention or heavy
baby movement, the tracking fails. We remedy this by
restarting the forehead detection and skipping frames
where detection fails. We manually inspected the
videos to make sure that forehead tracking failure did
not result in identifying facial features leaking into the
published videos.
2.5 Video Organization and Annotation
We organized the videos of each patient into a sepa-
rate directory. The videos of patient 01 and the as-
sociated annotation files go into directory P01. Each
patient directory contains a number of videos with an
associated annotation file in XML format.
File RecodingList includes metadata records
about all patient videos. Each record has the name
of the video file, the number of frames per second,
the day of the recording in preserved order, the length
of the video, the age of the baby at recording since
inception, and the status of the incubator.
It might also have temporal annotations indicating
when the baby was in states such as deep sleep, light
sleep, agitated, bradycardia, apnea and rest. Other
temporal annotations indicate when the region of in-
terest was detected, re-detected or lost.
Each video in a patient directory comes with an
associated xml video annotation file. The video anno-
tation file includes two sections. The first section pro-
vides metadata about the file including its name, dura-
tion, number of frames per second, width and height
of the captured region of interest, the status of the in-
cubator, feeding method, whether an event happened
during the recording and whether a nutritive feed hap-
pened during the recording.
The second section provides a time stamp from
the start of recording, the heart and respiratory rates.
For this purpose, we configured a dual MIB/RS232
serial cord to extract realtime vital signs from the
MP40-70 Philips Intellivue monitor. The second sec-
tion also has the status of the baby, whether an action
happened, and the location of the captured ROI with
respect to the video frame. It might also have some
notes taken by the healthcare providers. Table 3 de-
scribes the entire annotation scheme with examples
for each field.
3 RELATED WORK
Recently, several methods for extracting HR data
from video recordings of adult subjects have been
published. Eulerian Video Magnification (Wu et al.,
2012) (EVM) utilizes minute skin color variations and
low amplitude motion that are magnified to reveal sig-
nals of interest which reflect physiologic changes at
the cellular level such as changes in skin perfusion,
temperature and heart rate. Poh et al. (Poh et al.,
2010) used Blind Source Separation (BSS) based on
independent component analysis (ICA) of the red,
green, and blue (RGB) intensity channels in facial
videos for HR measurement. Alghoul et al. (Alghoul
et al., 2017) compared between EVM and ICA and
found approaches based on ICA to deal better with
lighting-related noise; however, approaches based on
EVM performed better with motion-related noise. A
comparison of three BSS-ICA based methods is found
in (Christinaki et al., 2014) where different statistical
transformations aimed at enhancing component sepa-
rability for extracting the HR.
EVM and BSS only require video recordings from
a close distance without any close contact with the pa-
tient hence they are non-invasive. In (Wu et al., 2012),
colour changes are tracked over time, thus permit-
ting analysis of physiological state changes such as
heart rate and subsequently perfusion. Those changes
would then be correlated with particular condition or
disease states for the purpose of automatic diagnosis
and alarm issuance.
4 UTILITY AND DISCUSSION
We performed video analysis to extract vital signs us-
ing two methods reported in the literature to validate
the sanity of our data set and establish its utility. The
video analysis proceeds in two steps: face detection
and heart rate extraction.
Face Detection: Baby faces maybe often partially
covered with medical equipment and dressing while
in the incubator. That led to failure of off-the-shelf
face detection algorithms. Thus for each video, and
in the first frame, we selected an initial region of in-
terest (ROI) containing the face. We set this as a face
template and used ROI tracking to capture it in the
rest of the video. This resulted in successful detection
throughout the recordings.
HR Extraction: We tested two methods to recover
the PPG signal from the detected faces. One is based
on analyzing the frequency content of the green chan-
nel(Wu et al., 2012), and the other is based on in-
dependent component analysis (ICA)(Alghoul et al.,
ICPRAM 2023 - 12th International Conference on Pattern Recognition Applications and Methods
770