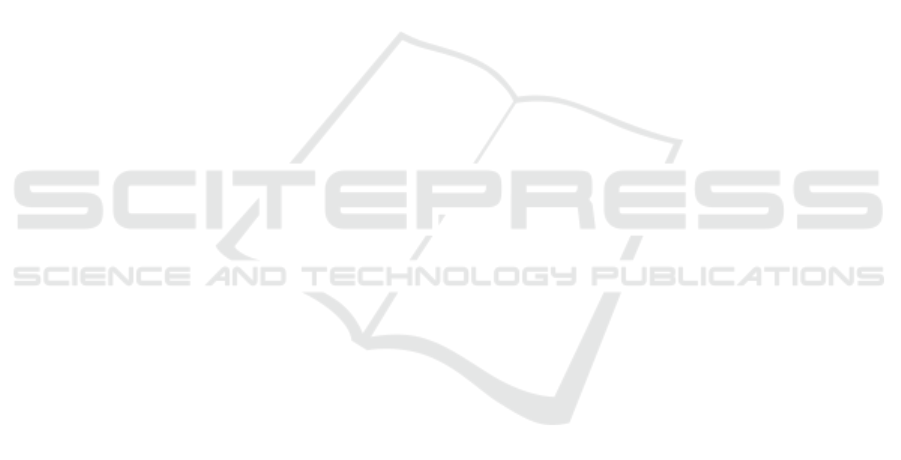
He, K., Zhang, X., Ren, S., and Sun, J. (2016). Deep resid-
ual learning for image recognition. In Proceedings of
the IEEE conference on computer vision and pattern
recognition, pages 770–778.
Herzenberg, L. A., De Rosa, S. C., and Herzenberg, L. A.
(2000). Monoclonal antibodies and the facs: comple-
mentary tools for immunobiology and medicine. Im-
munology today, 21(8):383–390.
Jesty, J., Yin, W., Perrotta, P., and Bluestein, D. (2003).
Platelet activation in a circulating flow loop: com-
bined effects of shear stress and exposure time.
Platelets, 14(3):143–149.
Kamath, S., Blann, A., and Lip, G. (2001). Platelet activa-
tion: assessment and quantification. European heart
journal, 22(17):1561–1571.
Klenk, C., Heim, D., Ugele, M., and Hayden, O. (2019).
Impact of sample preparation on holographic imaging
of leukocytes. Optical Engineering, 59(10):102403.
Lazaridis, D., Leung, S., Kohler, L., Smith, C. H., Kearson,
M. L., and Eraikhuemen, N. (2021). The impact of
anticoagulation on covid-19 (sars cov-2) patient out-
comes: a systematic review. Journal of Pharmacy
Practice, page 08971900211015055.
Lazo-Langner, A., Goss, G., Spaans, J., and Rodger, M.
(2007). The effect of low-molecular-weight heparin
on cancer survival. a systematic review and meta-
analysis of randomized trials. Journal of Thrombosis
and Haemostasis, 5(4):729–737.
Leytin, V., Mody, M., Semple, J. W., Garvey, B., and Freed-
man, J. (2000). Flow cytometric parameters for char-
acterizing platelet activation by measuring p-selectin
(cd62) expression: theoretical consideration and eval-
uation in thrombin-treated platelet populations. Bio-
chemical and biophysical research communications,
269(1):85–90.
Michelson, A. D., Barnard, M. R., Krueger, L. A., Va-
leri, C. R., and Furman, M. I. (2001). Circulat-
ing monocyte-platelet aggregates are a more sensitive
marker of in vivo platelet activation than platelet sur-
face p-selectin: studies in baboons, human coronary
intervention, and human acute myocardial infarction.
Circulation, 104(13):1533–1537.
Peerschke, E. I. and Zucker, M. B. (1981). Fibrino-
gen receptor exposure and aggregation of human
blood platelets produced by adp and chilling. Blood,
57(4):663–670.
Rampotas, A. and Pavord, S. (2021). Platelet aggregates, a
marker of severe covid-19 disease. Journal of Clinical
Pathology, 74(11):750–751.
Ramstack, J., Zuckerman, L., and Mockros, L. (1979).
Shear-induced activation of platelets. Journal of
biomechanics, 12(2):113–125.
Ren, S., He, K., Girshick, R., and Sun, J. (2015). Faster
r-cnn: Towards real-time object detection with region
proposal networks. Advances in neural information
processing systems, 28.
Riedl, J., Ay, C., and Pabinger, I. (2017). Platelets and
hemophilia: A review of the literature. Thrombosis
research, 155:131–139.
Sibbing, D., Braun, S., Jawansky, S., Vogt, W., Mehilli,
J., Sch
¨
omig, A., Kastrati, A., and von Beckerath, N.
(2008). Assessment of adp-induced platelet aggrega-
tion with light transmission aggregometry and multi-
ple electrode platelet aggregometry before and after
clopidogrel treatment. Thrombosis and haemostasis,
99(01):121–126.
Suzuki, S. et al. (1985). Topological structural analy-
sis of digitized binary images by border following.
Computer vision, graphics, and image processing,
30(1):32–46.
Ugele, M., Weniger, M., Stanzel, M., Bassler, M., Krause,
S. W., Friedrich, O., Hayden, O., and Richter, L.
(2018). Label-free high-throughput leukemia detec-
tion by holographic microscopy. Advanced Science,
5(12):1800761.
Wall
´
en, N. H., Ladjevardi, M., Albert, J., and Br
¨
oijers
´
en,
A. (1997). Influence of different anticoagulants on
platelet aggregation in whole blood; a comparison
between citrate, low molecular mass heparin and
hirudin. Thrombosis research, 87(1):151–157.
Whiting, D. and DiNardo, J. A. (2014). Teg and rotem:
technology and clinical applications. American jour-
nal of hematology, 89(2):228–232.
Measurement of Platelet Aggregation in Ageing Samples and After in-Vitro Activation
65