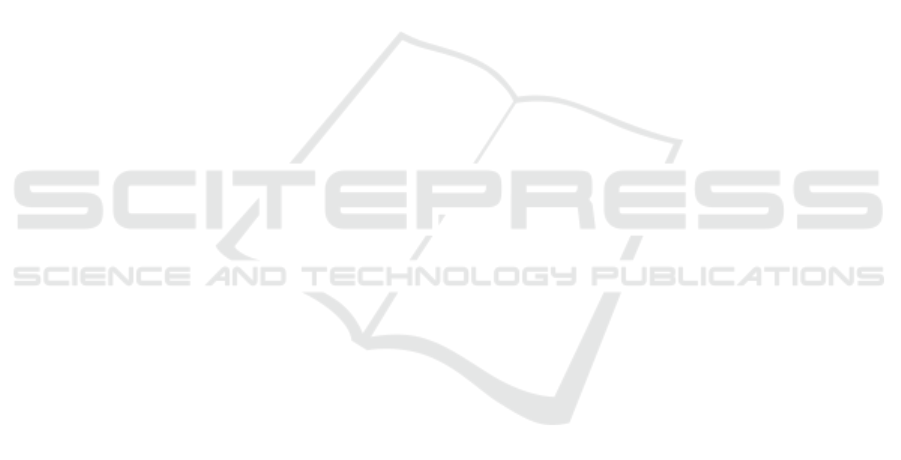
Hazard, J. and Haapio, H. (2017). Wise contracts: smart
contracts that work for people and machines. In
Trends and communities of legal informatics. Pro-
ceedings of the 20th international legal informatics
symposium IRIS, pages 425–432.
Huizing, A., Veenman, C., Neerincx, M., and Dijk, J.
(2020). Hybrid ai: The way forward in ai by develop-
ing four dimensions. In International Workshop on the
Foundations of Trustworthy AI Integrating Learning,
Optimization and Reasoning, pages 71–76. Springer.
Ian Horrocks, Peter F. Paterl-Scnheider, H. B. S. T. B. G.
M. D. Swrl: A semantic web rule language combining
owl and ruleml. https://www.w3.org/Submission/SW
RL/. Accessed: 1-8-2022.
ISO (2020). Iso 31022 risk management – guidelines for
the management of legal risk. http://www.iso.org.
Iversen, J. (2004). Legal risk management. Thomson Gad
Jura.
Kaltenboeck, M., Boil, P., Verhoeven, P., Sageder, C.,
Montiel-Ponsoda, E., and Calleja-Ib
´
a
˜
nez, P. (2022).
Using a legal knowledge graph for multilingual com-
pliance services in labor law, contract management,
and geothermal energy. In Technologies and Applica-
tions for Big Data Value, pages 253–271. Springer.
Kolvart, M., Poola, M., and Rull, A. (2016). Smart con-
tracts. In The Future of Law and etechnologies, pages
133–147. Springer.
Kozlova, M. Y. and Aleksandrina, M. (2020). “smart
contracts” vs legal technology in contract practice.
In Institute of Scientific Communications Conference,
pages 1204–1212. Springer.
Kruijff, J. d. and Weigand, H. (2017). Ontologies for
commitment-based smart contracts. In OTM Con-
federated International Conferences” On the Move
to Meaningful Internet Systems”, pages 383–398.
Springer.
Larson, E. J. (2021). The myth of artificial intelligence. In
The Myth of Artificial Intelligence. Harvard University
Press.
Macmullen, W. J. (2005). Annotation as process, thing, and
knowledge: Multi-domain studies of structured data
annotation. In ASIST Annual Meeting. Citeseer.
Mahler, T. (2007). Defining legal risk. SSRN, page 28.
Mason, J. (2017). Intelligent contracts and the construction
industry. Journal of Legal Affairs and Dispute Resolu-
tion in Engineering and Construction, 9(3):04517012.
McCarthy, J. (2004). What is artificial intelligence. URL:
http://www-formal. stanford. edu/jmc/whatisai. html.
McNamara, A. (2020). Automating the chaos: Intelligent
construction contracts. In Smart Cities and Construc-
tion Technologies. IntechOpen.
McNamara, A. and Sepasgozar, S. (2018). Barriers and
drivers of intelligent contract implementation in con-
struction. Management, 143:02517006.
McNamara, A. J. and Sepasgozar, S. M. (2020). Devel-
oping a theoretical framework for intelligent contract
acceptance. Construction innovation.
McNamara, A. J. and Sepasgozar, S. M. (2021). Intelli-
gent contract adoption in the construction industry:
Concept development. Automation in construction,
122:103452.
Montiel-Ponsoda, E., Gracia, J., and Rodr
´
ıguez-Doncel, V.
(2018). Building the legal knowledge graph for smart
compliance services in multilingual europe. In CEUR
workshop proc., number ART-2018-105821.
Paulheim, H. (2017). Knowledge graph refinement: A sur-
vey of approaches and evaluation methods. Semantic
web, 8(3):489–508.
Pillai, M. and Adavi, P. (2013). Intelligent contract manage-
ment. International Journal of Scientific and Research
Publications, 3(1).
Sarker, M. K., Schwartz, J., Hitzler, P., Zhou, L., Nadella,
S., Minnery, B., Juvina, I., Raymer, M. L., and
Aue, W. R. (2020). Wikipedia knowledge graph
for explainable ai. In Iberoamerican Knowledge
Graphs and Semantic Web Conference, pages 72–87.
Springer.
Schneider, J. M., Rehm, G., Montiel-Ponsoda, E.,
Rodr
´
ıguez-Doncel, V., Mart
´
ın-Chozas, P., Navas-
Loro, M., Kaltenb
¨
ock, M., Revenko, A., Karam-
patakis, S., Sageder, C., et al. (2022). Lynx: A
knowledge-based ai service platform for content pro-
cessing, enrichment and analysis for the legal domain.
Information Systems, 106:101966.
Smits, J. M. (2017). Contract law: a comparative introduc-
tion. Edward Elgar Publishing.
Sovrano, F., Palmirani, M., and Vitali, F. (2020). Le-
gal knowledge extraction for knowledge graph based
question-answering. In Legal Knowledge and Infor-
mation Systems, pages 143–153. IOS Press.
Stark, T. L. (2013). Drafting contracts: How and why
lawyers do what they do. Wolters Kluwer.
Szabo, N. et al. (1994). Smart contracts.
Timmer, I. (2019). Contract automation: Experiences from
dutch legal practice. In Legal Tech, Smart Contracts
and Blockchain, pages 147–171. Springer.
Vinay K. Chaudhri, Naren Chittar, M. G. An introduction
to knowledge graphs. http://ai.stanford.edu/blog/intro
duction-to-knowledge-graphs/. Accessed: 6-7-2022.
Wu, Y. (2020). Summary of research on contract risk man-
agement of epc general contracting project—based on
vosviewer knowledge graph analysis. In International
Symposium on Advancement of Construction Manage-
ment and Real Estate, pages 1043–1058. Springer.
Yu, H., Li, H., et al. (2021). A knowledge graph con-
struction approach for legal domain. Tehni
ˇ
cki vjesnik,
28(2):357–362.
Zheng, K., Zhang, Z., and Gauthier, J. (2020). Blockchain-
based intelligent contract for factoring business in
supply chains. Annals of Operations Research, pages
1–21.
Zhou, X., Lim, M. Q., and Kraft, M. (2020). A
smart contract-based agent marketplace for the j-park
simulator-a knowledge graph for the process industry.
Computers & Chemical Engineering, 139:106896.
ICAART 2023 - 15th International Conference on Agents and Artificial Intelligence
98