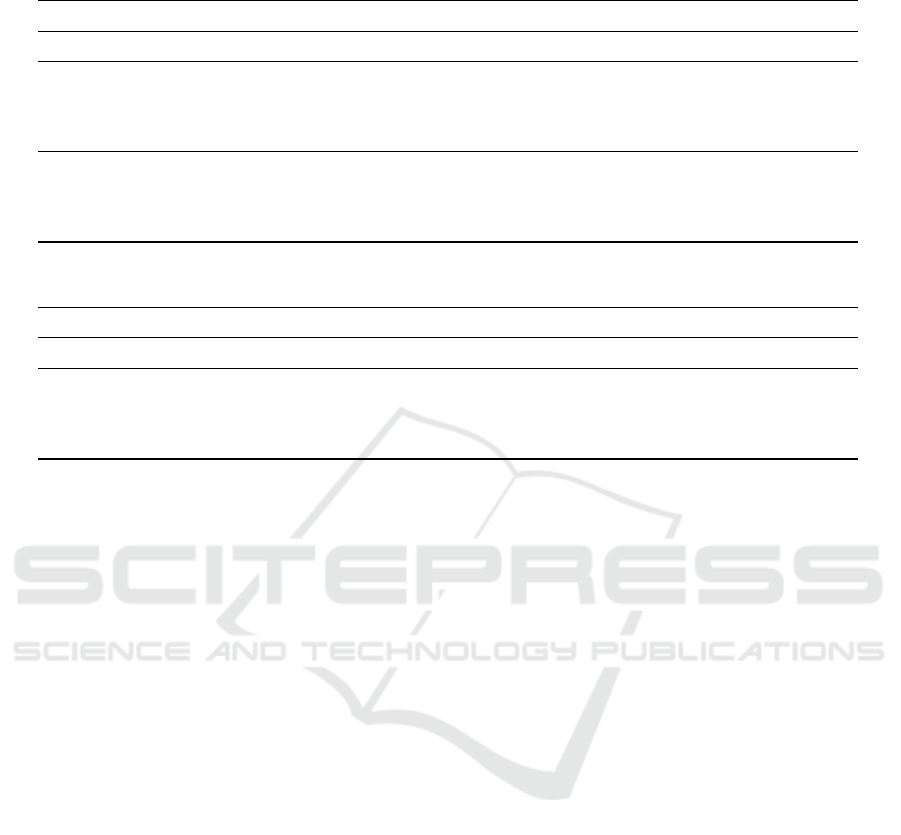
Table 3: Pearson correlation coefficient of Arabic STS systems on the STS dataset (the higher, the better). Para stands
for MSR-Paraphrase, Vid stands for MSR-Video, euro stands for SMT europarl datasets, n/a stands not applied.
With attention Without attention
Model Para Vid euro Para Vid euro
ArabicBERT 0.851 0.773 0.760 0.823 0.755 0.738
CAMeL-BERT 0.690 0.651 0.804 0.671 0.584 0.666
AraBERT 0.925 0.457 0.782 0.906 0.438 0.757
mBERT 0.771 0.502 0.714 0.755 0.452 0.690
(Alian and Awajan,
2021)
n/a n/a n/a 0.354 0.743 0.467
(Nagoudi and
Schwab, 2017)
n/a n/a n/a 0.182 0.691 0.206
Table 4: MSE of Arabic STS systems on the STS dataset (the lower, the better). Para stands for MSR-Paraphrase, Vid
stands for MSR-Video, euro stands for SMT europarl datasets.
With attention Without attention
Model Para Vid euro Para Vid euro
ArabicBERT 0.188 0.238 0.371 0.251 0.289 0.302
CAMeL-BERT 0.325 0.372 0.201 0.332 0.0.386 0.339
AraBERT 0.154 0.550 0.220 0.186 0.559 0.251
mBERT 0.285 0.489 0.382 0.302 0.498 0.395
Quranic verses semantic relatedness using arabert. In
Proceedings of the Sixth Arabic Natural Language
Processing Workshop, pages 185–190. Leeds.
Alzahrani, S. (2016). Cross-language semantic similarity of
arabic-english short phrases and sentences. J. Comput.
Sci., 12(1):1–18.
Antoun, W., Baly, F., and Hajj, H. (2020). Arabert:
Transformer-based model for arabic language under-
standing. arXiv preprint arXiv:2003.00104.
Chouikhi, H., Chniter, H., and Jarray, F. (2021). Arabic
sentiment analysis using bert model. In International
Conference on Computational Collective Intelligence,
pages 621–632. Springer.
Chouikhi., H., Chniter., H., and Jarray., F. (2021). Stack-
ing bert based models for arabic sentiment analysis.
In Proceedings of the 13th International Joint Con-
ference on Knowledge Discovery, Knowledge Engi-
neering and Knowledge Management - KEOD,, pages
144–150. INSTICC, SciTePress.
Devlin, J., Chang, M.-W., Lee, K., and Toutanova, K.
(2018). Bert: Pre-training of deep bidirectional trans-
formers for language understanding. arXiv preprint
arXiv:1810.04805.
Feifei, X., Shuting, Z., and Yu, T. (2020). Bert-based
siamese network for semantic similarity. In Journal
of Physics: Conference Series, volume 1684, page
012074. IOP Publishing.
H. Al-Bataineh, W. Farhan, A. M. H. S. and Al-Natsheh,
H. T. (2019). Deep contextualized pairwise semantic
similarity for arabic language questions. In 2019 IEEE
31st International Conference on Tools with Artificial
Intelligence (ICTAI), pages 1586–1591. IEEE.
Kingma, D. P. and Ba, J. (2014). Adam: A
method for stochastic optimization. arXiv preprint
arXiv:1412.6980.
Li, Y., McLean, D., Bandar, Z. A., O’shea, J. D., and Crock-
ett, K. (2006). Sentence similarity based on seman-
tic nets and corpus statistics. IEEE transactions on
knowledge and data engineering, 18(8):1138–1150.
Libovick
`
y, J., Rosa, R., and Fraser, A. (2019). How
language-neutral is multilingual bert? arXiv preprint
arXiv:1911.03310.
M. Hammad, M. Al-Smadi, Q. B. B. and Al-Zboon, S. A. A.
(2021). Using deep learning models for learning se-
mantic text similarity of arabic questions. 11(4):3519.
Nagoudi, E. and Schwab, D. (2017). Semantic similarity
of arabic sentences with word embeddings. In Third
arabic natural language processing workshop, pages
18–24.
Neculoiu, P., Versteegh, M., and Rotaru, M. (2016). Learn-
ing text similarity with siamese recurrent networks. In
Proceedings of the 1st Workshop on Representation
Learning for NLP, pages 148–157.
Safaya, A., Abdullatif, M., and Yuret, D. (2020). Bert-
cnn for offensive speech identification in social me-
dia. In Proceedings of the Fourteenth Workshop on
Semantic Evaluation”, Barcelona (online)”, Interna-
tional Committee for Computational Linguistics.
Saidi, R. and Jarray, F. (2022). Combining bert repre-
sentation and pos tagger for arabic word sense dis-
ambiguation. In International Conference on Intel-
ligent Systems Design and Applications, pages 676–
685. Springer.
Saidi, R., Jarray, F., and Alsuhaibani, M. (2022). Compara-
tive analysis of recurrent neural network architectures
for arabic word sense disambiguation. In Proceed-
ings of the 18th International Conference on Web In-
ICAART 2023 - 15th International Conference on Agents and Artificial Intelligence
150