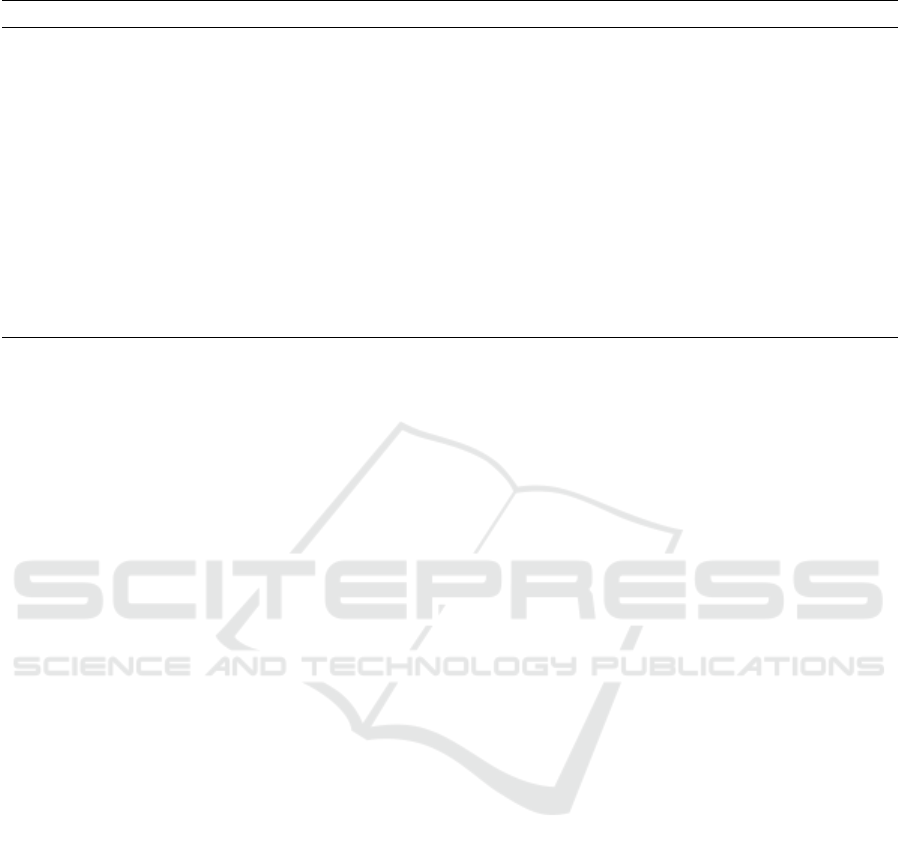
Table 8: Comparative Analysis.
Recent Works Breast IRIS Heart Diabetes Liver Hepatitis Sonar
(Denoeux, 2008) 76.3 80.57 75.81
(Xu et al., 2013) 82.59 79.4 72.57
(Xu et al., 2014) 95.6 85 85.5
(Xu et al., 2016) 83.7 79.88 68.26
(Liu et al., 2017) 85.56 83.85 76.02
(Qin and Xiao, 2018) 97.07 96.7 86.7 89.03
(Jiang et al., 2019) 69.91 95.33 75.19 70.96 76.48 81.29
(Pe
˜
nafiel et al., 2020) 93.85 95.9 72.7
(Song et al., 2021) 97.07 86.7 76.82 78.04
(Zhu et al., 2021) 97.15 99.33 91.48 81.61
(Ranjbar and Effati, 2022) 95.22 79.48 70.96 81.62
Proposed Method 98.24 100 85.36 81.81 67.52 89.65 76.19
of overlapping among the classes is the only factor
that has significant impact on the membership degrees
in probabilistic labels. Since identifying neighbours
for each instance is computationally complex, density
models or fuzzy approaches may be considered as the
prototype to convert hard labels to probabilistic labels
in future.
REFERENCES
Christopher, J. (2019). The science of rule-based classifiers.
In 2019 9th International Conference on Cloud Com-
puting, Data Science & Engineering (Confluence),
pages 299–303. IEEE.
Dempster, A. P. (2008). Upper and lower probabilities in-
duced by a multivalued mapping. In Classic works of
the Dempster-Shafer theory of belief functions, pages
57–72. Springer.
Denoeux, T. (2008). A k-nearest neighbor classification rule
based on dempster-shafer theory. In Classic works of
the Dempster-Shafer theory of belief functions, pages
737–760. Springer.
Gayar, N. E., Schwenker, F., and Palm, G. (2006). A study
of the robustness of knn classifiers trained using soft
labels. In IAPR Workshop on Artificial Neural Net-
works in Pattern Recognition, pages 67–80. Springer.
Hinton, G., Vinyals, O., Dean, J., et al. (2015). Distilling
the knowledge in a neural network. arXiv preprint
arXiv:1503.02531, 2(7).
Jiang, W., Huang, C., and Deng, X. (2019). A new prob-
ability transformation method based on a correlation
coefficient of belief functions. International Journal
of Intelligent Systems, 34(6):1337–1347.
Kavya, R. and Christopher, J. (2022). Interpretable sys-
tems based on evidential prospect theory for decision-
making. Applied Intelligence, pages 1–26.
Liu, Y.-T., Pal, N. R., Marathe, A. R., and Lin, C.-T. (2017).
Weighted fuzzy dempster–shafer framework for mul-
timodal information integration. IEEE Transactions
on Fuzzy Systems, 26(1):338–352.
Nguyen, Q., Valizadegan, H., and Hauskrecht, M. (2011).
Learning classification with auxiliary probabilistic in-
formation. In 2011 IEEE 11th International Confer-
ence on Data Mining, pages 477–486. IEEE.
Norouzi, M., Bengio, S., Jaitly, N., Schuster, M., Wu, Y.,
Schuurmans, D., et al. (2016). Reward augmented
maximum likelihood for neural structured prediction.
Advances In Neural Information Processing Systems,
29.
Pe
˜
nafiel, S., Baloian, N., Sanson, H., and Pino, J. A. (2020).
Applying dempster–shafer theory for developing a
flexible, accurate and interpretable classifier. Expert
Systems with Applications, 148:113262.
Qin, B. and Xiao, F. (2018). A non-parametric method to
determine basic probability assignment based on ker-
nel density estimation. IEEE Access, 6:73509–73519.
Ranjbar, M. and Effati, S. (2022). A new approach for fuzzy
classification by a multiple-attribute decision-making
model. Soft Computing, 26(9):4249–4260.
Song, X., Qin, B., and Xiao, F. (2021). Fr–kde: a hybrid
fuzzy rule-based information fusion method with its
application in biomedical classification. International
Journal of Fuzzy Systems, 23(2):392–404.
Szegedy, C., Vanhoucke, V., Ioffe, S., Shlens, J., and Wo-
jna, Z. (2016). Rethinking the inception architecture
for computer vision. In Proceedings of the IEEE con-
ference on computer vision and pattern recognition,
pages 2818–2826.
Vega, R., Gorji, P., Zhang, Z., Qin, X., Rakkunedeth, A.,
Kapur, J., Jaremko, J., and Greiner, R. (2021). Sam-
ple efficient learning of image-based diagnostic clas-
sifiers via probabilistic labels. In International Con-
ference on Artificial Intelligence and Statistics, pages
739–747. PMLR.
Xu, P., Davoine, F., Zha, H., and Denoeux, T. (2016). Evi-
dential calibration of binary svm classifiers. Interna-
tional Journal of Approximate Reasoning, 72:55–70.
Xu, P., Deng, Y., Su, X., and Mahadevan, S. (2013). A
new method to determine basic probability assign-
Nearest Neighbours and XAI Based Approach for Soft Labelling
115