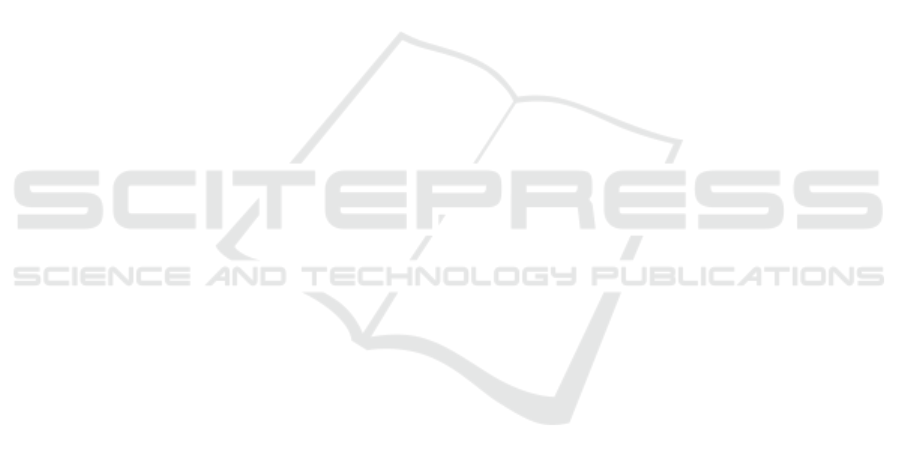
weighted algorithm and knn. In International Con-
ference on Sustainable Computing and Data Commu-
nication Systems, ICSCDS 2022 - Proceedings, pages
479–484. Cited By :2.
Padhiar, I., Seneviratne, O., Chari, S., Gruen, D., and
McGuinness, D. L. (2021). Semantic modeling for
food recommendation explanations.
Peake, G. and Wang, J. (2018). Explanation mining: Post
hoc interpretability of latent factor models for rec-
ommendation systems. In Proceedings of the 24th
ACM SIGKDD International Conference on Knowl-
edge Discovery & Data Mining, KDD ’18, page
2060–2069, New York, NY, USA. Association for
Computing Machinery.
Pecune, F., Callebert, L., and Marsella, S. (2022). Design-
ing persuasive food conversational recommender sys-
tems with nudging and socially-aware conversational
strategies. Frontiers in Robotics and AI, page 390.
Rehman, F., Khalid, O., Haq, N., Khan, A. u. R., Bilal, K.,
and Madani, S. (2017). Diet-right: A smart food rec-
ommendation system. KSII Transactions on Internet
and Information Systems, 11:2910–2925.
Ren, Y., Shi, Y., Zhang, K., Wang, X., Chen, Z., and
Li, H. (2022). A drug recommendation model based
on message propagation and ddi gating mechanism.
IEEE Journal of Biomedical and Health Informatics,
26(7):3478–3485.
Ricci, F., Rokach, L., and Shapira, B. (2015). Recommender
Systems Handbook, volume 32.
Rustam, F., Imtiaz, Z., Mehmood, A., Rupapara, V., Choi,
G. S., Din, S., and Ashraf, I. (2022). Automated dis-
ease diagnosis and precaution recommender system
using supervised machine learning. Multimedia Tools
and Applications, 81(22):31929–31952.
Saeedi, P., Petersohn, I., Salpea, P., Malanda, B., Karu-
ranga, S., Unwin, N., Colagiuri, S., Guariguata, L.,
Motala, A. A., Ogurtsova, K., Shaw, J. E., Bright,
D., Williams, R., and behalf of the IDF Diabetes At-
las Committee, O. (2019). Global and regional di-
abetes prevalence estimates for 2019 and projections
for 2030 and 2045: Results from the international di-
abetes federation diabetes atlas, 9th edition. Diabetes
research and clinical practice, 157. Cited By :3061.
Sajde, M., Malek, H., and Mohsenzadeh, M. (2022). Re-
comed: A knowledge-aware recommender system for
hypertension medications. Informatics in Medicine
Unlocked, 30:100950.
Strack, B., DeShazo, J. P., Gennings, C., Olmo, J. L., Ven-
tura, S., Cios, K. J., and Clore, J. N. (2014). Impact of
HbA1c Measurement on Hospital Readmission Rates:
Analysis of 70,000 Clinical Database Patient Records.
BioMed Research International, 2014:781670.
Tran, T. N. T., Atas, M., Felfernig, A., Le, V. M., Samer,
R., and Stettinger, M. (2019). Towards social choice-
based explanations in group recommender systems.
In Proceedings of the 27th ACM Conference on User
Modeling, Adaptation and Personalization, UMAP
’19, page 13–21, New York, NY, USA. Association
for Computing Machinery.
Tran, T. N. T., Felfernig, A., Trattner, C., and Holzinger,
A. (2021). Recommender systems in the healthcare
domain: state-of-the-art and research issues. Journal
of Intelligent Information Systems, 57:1–31.
Valdiviezo-D
´
ıaz, P. and Bobadilla, J. (2019). A hybrid ap-
proach of recommendation via extended matrix based
on collaborative filtering with demographics informa-
tion, volume 895 of Communications in Computer
and Information Science. Cited By :5.
Valdiviezo-Diaz, P., Ortega, F., Cobos, E., and Lara-
Cabrera, R. (2019). A collaborative filtering ap-
proach based on na
¨
ıve bayes classifier. IEEE Access,
7:108581–108592. Cited By :27.
Wang, N., Cai, X., Yang, L., and Mei, X. (2022).
Safe medicine recommendation via star interactive
enhanced-based transformer model. Computers in Bi-
ology and Medicine, 141:105159.
Wang, W. and Lu, Y. (2018). Analysis of the mean absolute
error (MAE) and the root mean square error (RMSE)
in assessing rounding model. IOP Conference Series:
Materials Science and Engineering, 324:012049.
Wang, Z., Huang, H., Cui, L., Chen, J., An, J., Duan, H.,
Ge, H., and Deng, N. (2020). Using natural language
processing techniques to provide personalized educa-
tional materials for chronic disease patients in china:
Development and assessment of a knowledge-based
health recommender system. JMIR Medical Informat-
ics, 8(4). Cited By :8.
Wedagu, M. A., Chen, D., Hussain, M. A. I., Gebremeskel,
T., Orlando, M. T., and Manzoor, A. (2020). Medicine
recommendation system for diabetes using prior med-
ical knowledge. In Proceedings of the 2020 4th In-
ternational Conference on Vision, Image and Signal
Processing, ICVISP 2020, New York, NY, USA. As-
sociation for Computing Machinery.
Yang, C. C. (2022). Explainable Artificial Intelligence for
Predictive Modeling in Healthcare. Journal of Health-
care Informatics Research, 6(2):228–239.
Yang, Z., Wu, B., Zheng, K., Wang, X., and Lei, L.
(2016). A survey of collaborative filtering-based rec-
ommender systems for mobile internet applications.
IEEE Access, 4:3273–3287.
Zeng, Y., Liu, X., Wang, Y., Shen, F., Liu, S., Rastegar-
Mojarad, M., Wang, L., and Liu, H. (2017). Rec-
ommending education materials for diabetic questions
using information retrieval approaches. Journal of
Medical Internet Research, 19:e342.
Zhang, Y., Chen, R., Tang, J., Stewart, W. F., and Sun,
J. (2017). Leap: Learning to prescribe effective and
safe treatment combinations for multimorbidity. In
Proceedings of the 23rd ACM SIGKDD International
Conference on Knowledge Discovery and Data Min-
ing, KDD ’17, page 1315–1324, New York, NY, USA.
Association for Computing Machinery.
Zhang, Y. and Chen, X. (2018). Explainable recommenda-
tion: A survey and new perspectives. Found. Trends
Inf. Retr., 14:1–101.
Zoppis, I., Manzoni, S., and Mauri, G. (2019). A com-
putational model for promoting targeted communica-
tion and supplying social explainable recommenda-
tions. In 2019 IEEE 32nd International Symposium
on Computer-Based Medical Systems (CBMS), pages
429–434.
ICAART 2023 - 15th International Conference on Agents and Artificial Intelligence
80