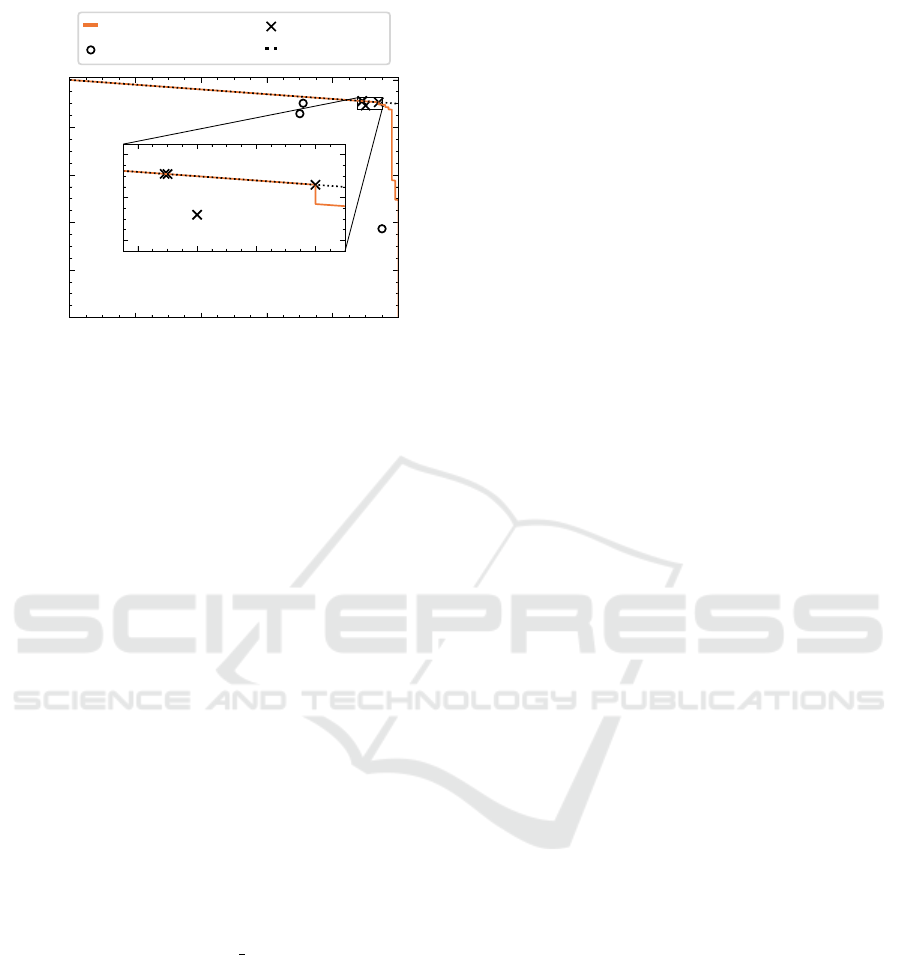
0.0 0.2 0.4 0.6 0.8 1.0
True Positive Rate
0.0
0.2
0.4
0.6
0.8
1.0
Saved Time
Ensemble
Beginner
Expert
Upper Bound
0.88 0.90 0.92 0.94
0.88
0.90
0.92
Figure 13: Human-machine performance comparison. Re-
sults on a randomly selected set of 100 positive and 100 neg-
ative samples. Medical experts perform significantly better
than beginner humans, who are outperformed by the auto-
mated model by a significant margin.
5 CONCLUSION
The results indicate that the detection of microscopic
yeast and fungi in clinical samples can be tackled by
standard deep-learning methods, employing an en-
semble of convolutional neural networks. The de-
veloped model consistently performs on par or better
than a human expert and, if deployed, should reduce
the amount of manual labor by approximately 87%
when operating at a true positive rate of 99%. The re-
sults are achieved with annotations only on the image
level, i.e., the network was not instructed what part of
the image is responsible for the classification.
ACKNOWLEDGEMENTS
The authors acknowledge the support of the OP VVV
project CZ.02.1.01/0.0/0.0/16 019/0000765 Research
Center for Informatics. The paper also acknowledges
project No. VJ02010041 supported by the Ministry
of the Interior of the Czech Republic. Special thanks
belongs to MUDr. Daniela L
ˇ
zi
ˇ
ca
ˇ
rov
´
a for collecting
the dataset and to MUDr. Kamila Dundrov
´
a, MUDr.
Vanda Chrenkov
´
a, Bc. Karla Ka
ˇ
nkov
´
a, Vladim
´
ır
Kryll, RNDr. Pavl
´
ına Lyskov
´
a, Ph. D. for filling out
the human-machine comparison survey.
REFERENCES
Dosovitskiy, A., Beyer, L., Kolesnikov, A., Weissenborn,
D., Zhai, X., Unterthiner, T., Dehghani, M., Min-
derer, M., Heigold, G., Gelly, S., Uszkoreit, J., and
Houlsby, N. (2021). An image is worth 16x16 words:
Transformers for image recognition at scale. ArXiv,
abs/2010.11929.
Gao, W., Li, M., Wu, R., Du, W., Zhang, S., Yin, S., Chen,
Z., and Huang, H. (2021). The design and appli-
cation of an automated microscope developed based
on deep learning for fungal detection in dermatology.
Mycoses, 64(3):245–251.
He, K., Zhang, X., Ren, S., and Sun, J. (2016). Identity
mappings in deep residual networks.
Mathison, B. A., Kohan, J. L., Walker, J. F., Smith,
R. B., Ardon, O., Couturier, M. R., and Pritt, B. S.
(2020). Detection of intestinal protozoa in trichrome-
stained stool specimens by use of a deep convolutional
neural network. Journal of Clinical Microbiology,
58(6):e02053–19.
P
´
erez, P., Gangnet, M., and Blake, A. (2003). Poisson im-
age editing. ACM Trans. Graph., 22(3):313–318.
Selvaraju, R. R., Cogswell, M., Das, A., Vedantam, R.,
Parikh, D., and Batra, D. (2017). Grad-cam: Visual
explanations from deep networks via gradient-based
localization. In 2017 IEEE International Conference
on Computer Vision (ICCV), pages 618–626.
Smith, K. P., Kang, A. D., and Kirby, J. E. (2018). Auto-
mated interpretation of blood culture gram stains by
use of a deep convolutional neural network. Journal
of Clinical Microbiology, 56(3):e01521–17.
Tan, M. and Le, Q. (2019). EfficientNet: Rethinking model
scaling for convolutional neural networks. In Chaud-
huri, K. and Salakhutdinov, R., editors, Proceedings of
the 36th International Conference on Machine Learn-
ing, volume 97 of Proceedings of Machine Learning
Research, pages 6105–6114. PMLR.
Tan, M. and Le, Q. V. (2021). Efficientnetv2: Smaller mod-
els and faster training. ArXiv, abs/2104.00298.
Yen, J.-C., Chang, F.-J., and Chang, S. (1995). A new
criterion for automatic multilevel thresholding. IEEE
Transactions on Image Processing, 4(3):370–378.
Zieli
´
nski, B., Sroka-Oleksiak, A., Rymarczyk, D., Piekar-
czyk, A., and Brzychczy-Włoch, M. (2020). Deep
learning approach to describe and classify fungi mi-
croscopic images. PLOS ONE, 15(6):1–16.
VISAPP 2023 - 18th International Conference on Computer Vision Theory and Applications
784