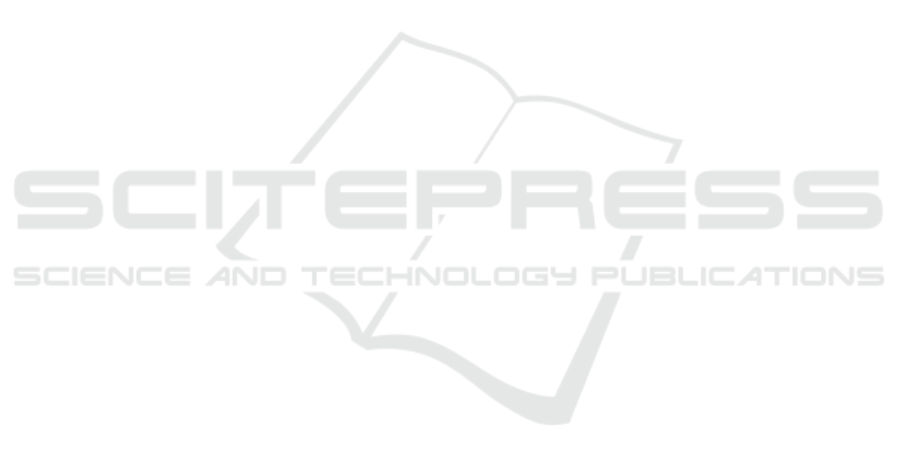
Tsai, Y.-H. H., Bai, S., Liang, P. P., Kolter, J. Z., Morency,
L.-P., and Salakhutdinov, R. (2019). Multimodal
transformer for unaligned multimodal language se-
quences. In The 57th Annual Meeting of the Associa-
tion for Computational Linguistics, pages 6558–6569.
Van Horn, G., Branson, S., Farrell, R., Haber, S., Barry,
J., Ipeirotis, P., Perona, P., and Belongie, S. (2015).
Building a bird recognition app and large scale dataset
with citizen scientists. In Computer Vision and Pattern
Recognition (CVPR).
Vaswani, A., Shazeer, N., Parmar, N., Uszkoreit, J., Jones,
L., Gomez, A. N., Kaiser, L. u., and Polosukhin, I.
(2017). Attention is all you need. In Advances in
Neural Information Processing Systems, volume 30.
Wang, H., Zhu, Y., Adam, H., Yuille, A., and Chen, L.
(2021a). Max-deeplab: End-to-end panoptic segmen-
tation with mask transformers. In 2021 IEEE/CVF
Conference on Computer Vision and Pattern Recog-
nition (CVPR), pages 5459–5470.
Wang, J., Yu, X., and Gao, Y. (2021b). Feature fusion vision
transformer for fine-grained visual categorization. In
2021 British Machine Vision Conference (BMVC).
Wang, J., Yu, X., and Gao, Y. (2021c). Mask guided atten-
tion for fine-grained patchy image classification. In
2021 IEEE International Conference on Image Pro-
cessing (ICIP). IEEE.
Wang, L., Lu, H., Wang, Y., Feng, M., Wang, D., Yin, B.,
and Ruan, X. (2017). Learning to detect salient objects
with image-level supervision. In IEEE Conference on
Computer Vision and Pattern Recognition.
Wang, Y., Morariu, V. I., and Davis, L. S. (2018). Learning a
discriminative filter bank within a cnn for fine-grained
recognition. In 2018 Conference on Computer Vision
and Pattern Recognition, pages 4148–4157.
Wang, Z., Wang, S., Yang, S., Li, H., Li, J., and Li, Z.
(2020). Weakly supervised fine-grained image classi-
fication via guassian mixture model oriented discrim-
inative learning. In 2020 Conference on Computer Vi-
sion and Pattern Recognition, pages 9746–9755.
Welinder, P., Branson, S., Mita, T., Wah, C., Schroff, F., Be-
longie, S., and Perona, P. (2010). Caltech-ucsd birds
200. Technical Report CNS-TR-201, Caltech.
Xiao, T., Xu, Y., Yang, K., Zhang, J., Peng, Y., and
Zhang, Z. (2015). The application of two-level atten-
tion models in deep convolutional neural network for
fine-grained image classification. In 2015 IEEE Con-
ference on Computer Vision and Pattern Recognition
(CVPR), pages 842–850.
Xie, E., Wang, W., Wang, W., Sun, P., Xu, H., Liang,
D., and Luo, P. (2021a). Segmenting transparent ob-
ject in the wild with transformer. arXiv pre-print
arXiv:2101.0846.
Xie, J., Pang, Y., Khan, M. H., Anwer, R. M., Khan, F. S.,
and Shao, L. (2021b). Mask-guided attention network
and occlusion-sensitive hard example mining for oc-
cluded pedestrian detection. IEEE Transactions on
Image Processing, 30:3872–3884.
Xie, L., Tian, Q., Hong, R., Yan, S., and Zhang, B. (2013).
Hierarchical part matching for fine-grained visual cat-
egorization. In 2013 IEEE International Conference
on Computer Vision, pages 1641–1648.
Yang, S., Liu, S., Yang, C., and Wang, C. (2021). Re-rank
coarse classification with local region enhanced fea-
tures for fine-grained image recognition. arXiv pre-
print arXiv:2102.09875.
Yu, C., Zhao, X., Zheng, Q., Zhang, P., and You, X. (2018).
Hierarchical bilinear pooling for fine-grained visual
recognition. In 2018 European Conference on Com-
puter Vision (ECCV), pages 595–610.
Yu, F., Huang, K., Wang, M., Cheng, Y., Chu, W., and Cui,
L. (2022). Width & depth pruning for vision trans-
formers. Proceedings of the AAAI Conference on Ar-
tificial Intelligence, 36(3):3143–3151.
Yu, X., Zhao, Y., Gao, Y., Yuan, X., and Xiong, S. (2021).
Benchmark platform for ultra-fine-grained visual cat-
egorization beyond human performance. In Proceed-
ings of the IEEE/CVF International Conference on
Computer Vision (ICCV), pages 10285–10295.
Zhang, Y., Cao, J., Zhang, L., Liu, X., Wang, Z., Ling, F.,
and Chen, W. (2022). A free lunch from vit: adap-
tive attention multi-scale fusion transformer for fine-
grained visual recognition. In 2022 IEEE Interna-
tional Conference on Acoustics, Speech and Signal
Processing, pages 3234–3238.
Zhao, B., Wu, X., Feng, J., Peng, Q., and Yan, S. (2017).
Diversified visual attention networks for fine-grained
object classification. IEEE Transactions on Multime-
dia, 19(6):1245–1256.
Zhao, Y., Yu, X., Gao, Y., and Shen, C. (2022). Learn-
ing discriminative region representation for person re-
trieval. Pattern Recognition, 121:108229.
Zheng, H., Fu, J., Zha, Z., and Luo, J. (2019a). Looking
for the devil in the details: Learning trilinear attention
sampling network for fine-grained image recognition.
In 2019 IEEE/CVF Conference on Computer Vision
and Pattern Recognition (CVPR), pages 5007–5016.
Zheng, H., Fu, J., Zha, Z.-J., and Luo, J. (2019b). Learn-
ing deep bilinear transformation for fine-grained im-
age representation. In Advances in Neural Information
Processing Systems, volume 32.
Zheng, S., Lu, J., Zhao, H., Zhu, X., Luo, Z., Wang, Y.,
Fu, Y., Feng, J., Xiang, T., Torr, P. H., and Zhang,
L. (2021). Rethinking semantic segmentation from a
sequence-to-sequence perspective with transformers.
In 2021 IEEE/CVF Conference on Computer Vision
and Pattern Recognition (CVPR), pages 6877–6886.
Zhou, B., Lapedriza, A., Xiao, J., Torralba, A., and Oliva,
A. (2014). Learning deep features for scene recog-
nition using places database. In Advances in Neural
Information Processing Systems, page 487–495.
Zhu, H., Ke, W., Li, D., Liu, J., Tian, L., and Shan, Y.
(2022). Dual cross-attention learning for fine-grained
visual categorization and object re-identification. In
2022 IEEE/CVF Conference on Computer Vision and
Pattern Recognition (CVPR), pages 4682–4692.
Zhu, X., Su, W., Lu, L., Li, B., Wang, X., and Dai, J. (2021).
Deformable DETR: Deformable transformers for end-
to-end object detection. In International Conference
on Learning Representations.
VISAPP 2023 - 18th International Conference on Computer Vision Theory and Applications
38