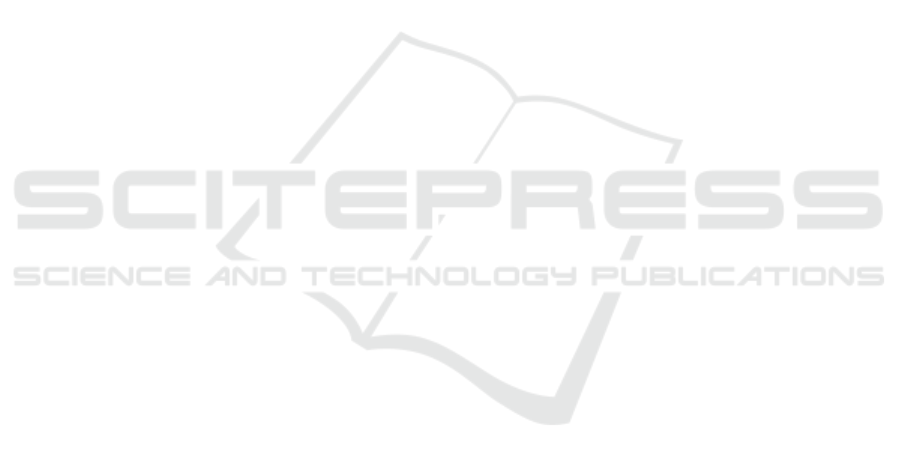
an agent separate three partial reward functions for
self and the other agents, and an interaction with the
agents approximately, and estimates a reward func-
tion for self based on only acquired rewards to learn
without factors of the other agent and an interaction
with the other agent. The experiments compared the
ICL method with A3C and the SOM as baseline meth-
ods. The results show (1) the ICL method outper-
formed all of the other methods; and (2) the ICL
method can avoid unstable policy which causes a bad
effect to an interaction between agents and keeps a
new valuable equilibrium.
This paper showed the ICL method has two kinds
of limitation: learning stability and premise of dis-
tribution. To overcome the limitation, we will apply
the MEIRL method for the ICL method in the future.
After that, we will examine a suitable distribution for
the reward function. We premised a distribution com-
bined two distributions for two agents, and each dis-
tribution is enough to be a normal distribution.
ACKNOWLEDGEMENTS
This research was supported by JSPS Grant on
JP21K17807 and Azbil Yamatake General Founda-
tion.
REFERENCES
Du, Y., Liu, B., Moens, V., Liu, Z., Ren, Z., Wang, J.,
Chen, X., and Zhang, H. (2021). Learning Correlated
Communication Topology in Multi-Agent Reinforce-
ment Learning, page 456–464. International Founda-
tion for Autonomous Agents and Multiagent Systems,
Richland, SC.
Fujita, Y., Kataoka, T., Nagarajan, P., and Ishikawa, T.
(2019). Chainerrl: A deep reinforcement learning li-
brary. In Workshop on Deep Reinforcement Learning
at the 33rd Conference on Neural Information Pro-
cessing Systems, Vancouver, Canada.
Ghosh, A., Tschiatschek, S., Mahdavi, H., and Singla, A.
(2020). Towards Deployment of Robust Cooperative
AI Agents: An Algorithmic Framework for Learning
Adaptive Policies, page 447–455. International Foun-
dation for Autonomous Agents and Multiagent Sys-
tems, Richland, SC.
Kim, D., Moon, S., Hostallero, D., Kang, W. J., Lee, T.,
Son, K., and Yi, Y. (2019). Learning to schedule com-
munication in multi-agent reinforcement learning.
Kingma, D. P. and Ba, J. (2017). Adam: A method for
stochastic optimization. CoRR, abs/1412.6980.
Mnih, V., Badia, A. P., Mirza, M., Graves, A., Lillicrap,
T. P., Harley, T., Silver, D., and Kavukcuoglu, K.
(2016). Asynchronous methods for deep reinforce-
ment learning. CoRR, abs/1602.01783.
Raileanu, R., Denton, E., Szlam, A., and Fergus, R. (2018).
Modeling others using oneself in multi-agent rein-
forcement learning. In Proceedings of the 35th Inter-
national Conference on Machine Learning, volume 80
of Proceedings of Machine Learning Research, pages
4257–4266, Stockholmsmassan, Stockholm Sweden.
Ziebart, B. D., Maas, A., Bagnell, J., and Dey, A. K. (2008).
Maximum entropy inverse reinforcement learning. In
Proceedings of the 23rd AAAI Conference on Artificial
Intelligence (AAAI2008), pages 1433–1438, Chicago,
USA. AAAI.
Implicit Cooperative Learning on Distribution of Received Reward in Multi-Agent System
153