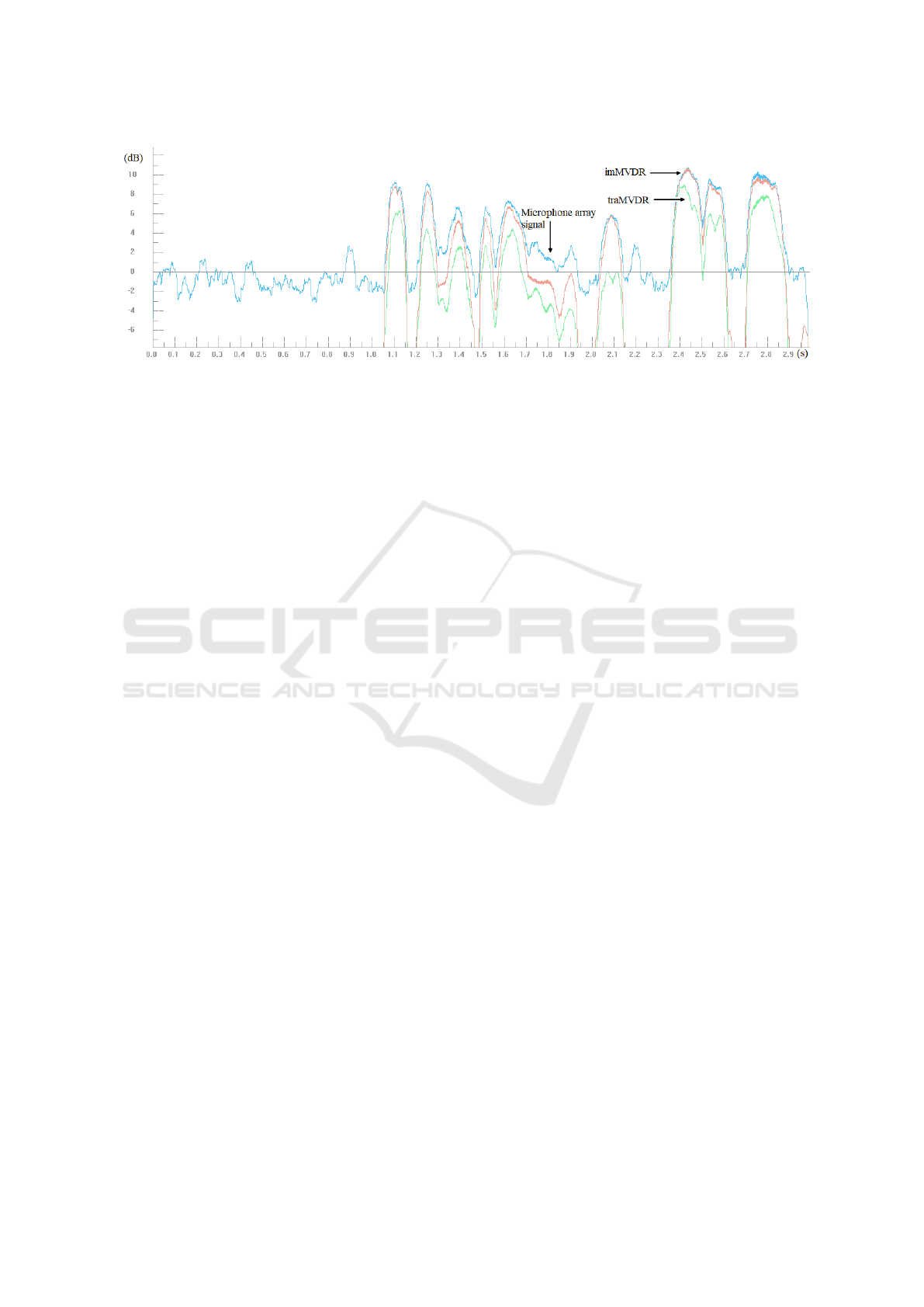
Figure 9: The illustrated energy of microphone array signal and traMVDR, imMVDR.
timation of speech presence probability can be more
applied into several approaches to enhance the perfor-
mance of speech enhancement system.
ACKNOWLEDGEMENTS
This research was supported supported by Digital
Agriculture Cooperative. The author thank our col-
leagues from Digital Agriculture Cooperative, who
provided insight and expertise that greatly assisted the
research.
REFERENCES
Ba, D. E., Florencio, D., and Zhang, C. (2007). Enhanced
MVDR Beamforming for Arrays of Directional Mi-
crophones. In 2007 IEEE International Conference
on Multimedia and Expo, pages 1307–1310. https:
//doi.org/10.1109/ICME.2007.4284898.
Benesty, J., Chen, J., and Huang, Y. (2008). Microphone
Array Signal Processing, volume 1 of Springer Top-
ics in Signal Processing. Springer Berlin, Heidelberg.
https://doi.org/10.1007/978-3-540-78612-2.
Benesty, J., Chen, J., and Pan, C. (2016). Fundamentals
of Differential Beamforming. SpringerBriefs in Elec-
trical and Computer Engineering. Springer Singapore.
https://doi.org/10.1007/978-981-10-1046-0.
Benesty, J., Cohen, I., and Chen, J. (2017). Fundamen-
tals of Signal Enhancement and Array Signal Process-
ing. John Wiley & Sons Singapore. https://doi.org/10.
1002/9781119293132.
Brandstein, M. and Ward, D., editors (2001). Microphone
Arrays: Signal Processing Techniques and Applica-
tions. Digital Signal Processing. Springer Berlin, Hei-
delberg. https://doi.org/10.1007/978-3-662-04619-7.
Chen, C.-Y. and Vaidyanathan, P. P. (2007). Quadratically
Constrained Beamforming Robust Against Direction-
of-Arrival Mismatch. IEEE Transactions on Sig-
nal Processing, 55(8):4139–4150. https://doi.org/10.
1109/TSP.2007.894402.
Ellis, D. (2011). Objective measures of speech qual-
ity/SNR. https://labrosa.ee.columbia.edu/projects/
snreval/.
Erdogan, H., Hershey, J. R., Watanabe, S., Mandel, M. I.,
and Roux, J. L. (2016). Improved MVDR Beam-
forming Using Single-Channel Mask Prediction Net-
works. In Proc. Interspeech 2016, pages 1981–1985.
https://doi.org/10.21437/Interspeech.2016-552.
Gannot, S., Burshtein, D., and Weinstein, E. (2001a). Signal
enhancement using beamforming and nonstationarity
with applications to speech. IEEE Transactions on
Signal Processing, 49(8):1614–1626. https://doi.org/
10.1109/78.934132.
Gannot, S., Burshtein, D., and Weinstein, E. (2001b). The-
oretical Performance Analysis of the General Trans-
fer Function GSC. In Proc. Int. Workshop Acous-
tic Echo Noise Control. https://www.eng.biu.ac.il/
∼
gannot/articles/Perf.pdf.
Gerkmann, T. and Hendriks, R. C. (2012a). Unbiased
MMSE-Based Noise Power Estimation With Low
Complexity and Low Tracking Delay. IEEE Trans-
actions on Audio, Speech, and Language Process-
ing, 20(4):1383–1393. https://doi.org/10.1109/TASL.
2011.2180896.
Gerkmann, T. and Hendriks, R. C. (2012b). Unbiased
MMSE-Based Noise Power Estimation With Low
Complexity and Low Tracking Delay. IEEE Trans-
actions on Audio, Speech, and Language Process-
ing, 20(4):1383–1393. https://doi.org/10.1109/TASL.
2011.2180896.
Lockwood, M. E., Jones, D. L., Bilger, R. C., Lansing,
C. R., O’Brien, W. D., Wheeler, B. C., and Feng, A. S.
(2004). Performance of time- and frequency-domain
binaural beamformers based on recorded signals from
real rooms. The Journal of the Acoustical Society of
America, 115(1):379–391. https://doi.org/10.1121/1.
1624064.
Lorenz, R. G. and Boyd, S. P. (2005). Robust minimum
variance beamforming. IEEE Transactions on Sig-
nal Processing, 53(5):1684–1696. https://doi.org/10.
1109/TSP.2005.845436.
Pan, C., Chen, J., and Benesty, J. (2014). On the noisere-
duction performance of the MVDR beamformer in-
noisy and reverberant environments. In 2014 IEEE
International Conference on Acoustics, Speech and
An Improved Diagonal Loading-Based Minimum Variance Distortionless Response Beamformer
25