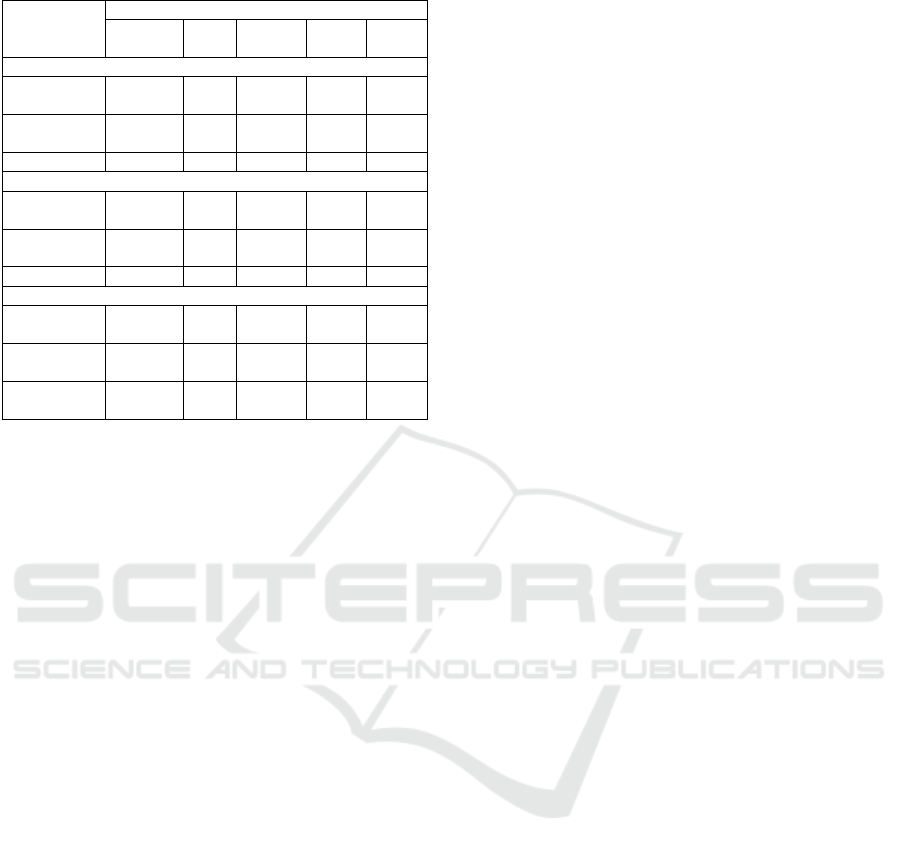
Table 4: Chi-Squared Result of FAHP Method.
Observed Number Cell (Oi)
Expected Number Cell (Ei)
4 CONCLUSIONS
This study can determine the 318 Ponorogo village's
vulnerability to landslides using the Fuzzy Analytic
Hierarchy Process method based on five criteria,
including rainfall, land height, land slope, land use,
and soil type. The mapping results from the
calculation of the FAHP method can be classified into
four levels of vulnerability: areas with a level of
vulnerability, landslides are high, medium, low, and
very low.
The results of the landslide-prone areas are
validated using natural-break calculation with data
obtained when calculating FAHP and AHP. So the
results of Fuzzy AHP give the best value compared to
AHP, which shows a value of 0.75 rather than 0.69.
ACKNOWLEDGEMENTS
The authors would like to thank to Politeknik
Elektronika Negeri Surabaya for supporting this
research.
REFERENCES
Basofi, A., Fariza, A., Ahsan, A. S., Kamal, I. M. (2015). A
comparison between natural and Head/tail breaks in
LSI (Landslide Susceptibility Index) classification for
landslide susceptibility mapping: A case study in
Ponorogo, East Java, Indonesia. International
Conference on Science in Information Technology
(ICSITech).
Basofi, A., Fariza, A., Kamal, I. M. (2019). Mitigation and
Emergency Management System of Landslide in
Ponorogo District, Indonesia. JOIV: International
Journal on Informatics Visualization. Vol. 3 No. 2.
pp.100-107.
Basofi, A., Nailussa'ada, Fariza, A. (2017). Landslide
susceptibility mapping using ensemble fuzzy
clustering: A case study in Ponorogo, East Java,
Indonesia". 2nd International conferences on
Information Technology, Information Systems and
Electrical Engineering (ICITISEE).
Dzulkarnain, M.R., Fariza, A., Basofi, A. (2016). Mobile
based of mitigation and emergency system for landslide
in ponorogo, East Java, Indonesia, International
Electronics Symposium (IES).
Basofi, A., Rahmana, R., Fariza, A. (2019). Pencarian Jalur
Alternatif Sebagai Sistem Evakuasi Bencana Tanah
Longsor di Kabupaten Ponorogo Dengan Algoritma A-
Star Berbasis Perangkat Bergerak. SCAN-Jurnal
Teknologi Informasi dan Komunikasi. Vol. 13 No. 3.
pp.1-8.
Hardiyatno, C.H. (2012). Tanah Longsor dan Erosi
Kejadian dan Penanganan. Gajah Mada University
Press.
Intan, A., Shofie, A., Widi, S. (2018). Analytical Hierarchy
Process(AHP), Fuzzy AHP, and TOPSIS for
Determining Bridge Maintenance Priority Scale in
Banjarsari. Surakarta.
Sujatha, E. R., Rajamanickam, G. V., Kumaravel, P.
(2012). Landslide susceptibility analysis using
Probabilistic Certainty Factor Approach: A case study
on Tevankarai stream watershed, India. School of Civil
Engineering, SASTRA University.
Xiong, T., Indrawan, I.G.B., Prakasa, B. D. (2017).
Landslide Susceptibility Mapping Using AHP,
Statistical Index, Index of Enthropy, and Logistic
Regression Approaces In the Tinalah Watershed.
Yuniarta, H., Saido, A.P., Purwana, Y.M. (2015).
Kerawanan Bencana Tanah Longsor Kabupaten
Ponorogo. Universitas Sebelas Maret Surakarta.
The Assessment of Landslide Vulnerability Levels in Ponorogo, Indonesia, Using Fuzzy Analytical Hierarchy Process: Natural Breaks
199