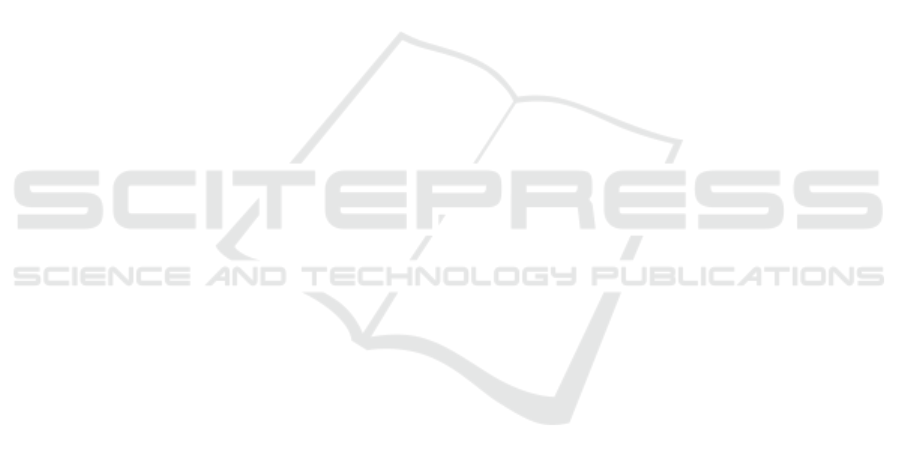
formation, and COVID, Chandos Digital Information
Review, pages 169–182. Chandos Publishing.
Azeroual, O., Sch
¨
opfel, J., Ivanovic, D., and Nikiforova,
A. (2022). Combining Data Lake and Data Wran-
gling for Ensuring Data Quality in CRIS. In In
CRIS2022: 15th International Conference on Current
Research Information Systems, In CRIS2022: 15th In-
ternational Conference on Current Research Informa-
tion Systems.
Bryant, R., Jan Fransen, P. d. C., Helmstutler, B., and
Scherer, D. (2021). Research Information Manage-
ment in the United States: Part 1—Findings and Rec-
ommendations.
Commission, E. (2020a). Exploitation & Open Science in
Horizon Europe.
Commission, E. (2020b). Facts and Figures for open re-
search data.
Commission, E. (2020c). Research and innovation.
Commission, E. (2021). Open Science.
Corte-Real, N., Ruivo, P., and Oliveira, T. (2020). Lever-
aging internet of things and big data analytics initia-
tives in European and American firms: Is data quality
a way to extract business value? Information & Man-
agement, 57:103141.
Danowski, P., Ferus, A., Hikl, A.-L., McNeill, G.,
Miniberger, C., Reding, S., Zarka, T., and Zojer,
M. (2020). ”Empfehlung” f
¨
ur die weitere Vor-
gangsweise f
¨
ur das Open-Access-Monitoring. Deliv-
erable des AT2OA-Teilprojekts TP1-B. Mitteilun-
gen der Vereinigung
¨
Osterreichischer Bibliothekarin-
nen und Bibliothekare, 73:278–284.
Donohue, T., Mennielli, M., and Wilcox, D. (2018). DuraS-
pace for FAIRness and Data Protection: DSpace and
Fedora. In CRIS2018: 14th International Conference
on Current Research Information Systems (Ume
˚
a,
June 13-16, 2018).
Engelman, A., Enkvist, C., and Pettersson, K. (2019). A
FAIR archive based on the CERIF model. Procedia
Computer Science, 146:190–200.
Europe, S. (2016). Science Europe Position Statement on
Research Information Systems.
European Commission, D.-G. f. R. and Innovation (2019).
Cost-benefit analysis for FAIR research data : cost of
not having FAIR research data.
European Commission, D.-G. f. R. and Innovation (2021).
Towards a reform of the research assessment system :
scoping report. Publications Office.
FAIRsFAIR (2022). Practical solutions for the use of FAIR
principles throughout the data life cycle.
Ferraris, A., Mazzoleni, A., Devalle, A., and Couturier, J.
(2018). Big data analytics capabilities and knowl-
edge management: impact on firm performance. Man-
agement Decision. Management Decision, 57:1923–
1936.
Flores, J. R., Brodeur, J. J., Daniels, M. G., Nicholls, N.,
and Turnator, E. (2015). Libraries and the research
data management landscape. The process of discov-
ery: The CLIR postdoctoral fellowship program and
the future of the academy, 2010:82–102.
FOSTER (2020). Assessing the FAIRness of data .
Guba, E. and Lincoln, Y. (1989). Fourth generation evalu-
ation. SAGE Publications, Inc.
Hasselbring, W., Carr, L., Hettrick, S., Packer, H., and
Tiropanis, T. (2020). From FAIR research data toward
FAIR and open research software. it - Information
Technology, 62(1):39–47.
Insider, L. (2022). Research Information definition.
Ivanovi
´
c, D., Ivanovi
´
c, L., and Layfield, C. (2019). FAIR-
ness at University of Novi Sad - Discoverability of
PhD research results for Non-Serbian scientific com-
munity–. Procedia Computer Science, 146:3–10.
Jetten, M. and Simons, E. (2019). Research data manage-
ment incorporated in a Research Information Manage-
ment system. A case study on archiving data sets and
writing Data Management Plans at Radboud Univer-
sity, the Netherlands. In EUNIS19: 25th EUNIS An-
nual Congress (June 5-7, 2019, NTNU, Trondheim,
Norway).
Jetten, M., Simons, E., and Rijnders, J. (2018). The role
of CRIS’s in the research life cycle. A case study on
implementing a FAIR RDM policy at Radboud Uni-
versity, the Netherlands. In Sicilia, M., Simons, E.,
Clements, A., de Castro, P., and Bergstr
¨
om, J., ed-
itors, 14th International Conference on Current Re-
search Information Systems, CRIS 2018, FAIRness
of Research Information, Ume
˚
a, Sweden, June 14-
16, 2018, volume 146 of Procedia Computer Science,
pages 156–165. Elsevier.
Landi, A., Thompson, M., Giannuzzi, V., Bonifazi, F.,
Labastida, I., da Silva Santos, L. O. B., and Roos, M.
(2020). The “A” of FAIR – As Open as Possible, as
Closed as Necessary. Data Intelligence, 2(1-2):47–55.
Lindel
¨
ow, C. (2019). FAIR already? Principles of reusabil-
ity and research output – evaluation at a national level.
In Spring 2019 euroCRIS Membership Meeting (CSC,
Espoo-Helsinki, Finland, May 27-29, 2019).
Masuzzo, P. C. (2022). Open Science & FAIR data: infinite
possibilities.
Mayer, G., M
¨
uller, W., Schork, K., Uszkoreit, J., Weide-
mann, A., Wittig, U., Rey, M., Quast, C., Felden,
J., Gl
¨
ockner, F. O., Lange, M., Arend, D., Beier, S.,
Junker, A., Scholz, U., Sch
¨
uler, D., Kestler, H. A.,
Wibberg, D., P
¨
uhler, A., Twardziok, S., Eils, J., Eils,
R., Hoffmann, S., Eisenacher, M., and Turewicz, M.
(2021). Implementing FAIR data management within
the German Network for Bioinformatics Infrastructure
(de.NBI) exemplified by selected use cases. Briefings
in Bioinformatics, 22(5).
Miniberger, C. and Reding, S. (2017). From data collec-
tion to FAIR use in CRIS. The case of University of
Vienna. In euroCRIS Strategic Membership Meeting
Autumn 2017 (CVTI SR, Bratislava, Slovakia, Nov 20-
22, 2017).
Mornati, S. (2019). Enhancing interoperability: the imple-
mentation of OpenAIRE Guidelines and COAR NGR
Recommendations in CRIS/RIMS. In OR19 Work-
shop on Repository/CRIS Interoperability.
Mornati, S., Bollini, A., and Pascarelli, L. (2018). How
to make research information FAIR: DSpace-CRIS
KMIS 2022 - 14th International Conference on Knowledge Management and Information Systems
70