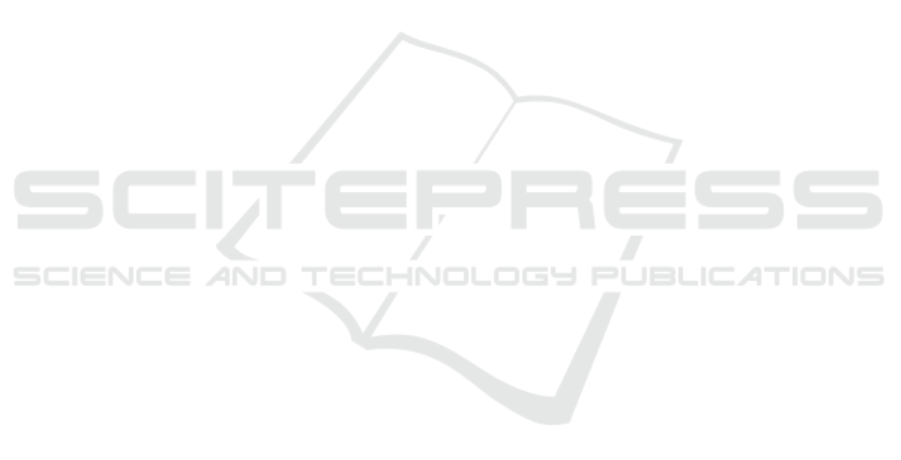
Flament, C. (1963). Applications of Graph Theory to Group
Structure. Prentice-Hall Series in Mathematical Anal-
ysis of Social Behavior.
Freeman, L. C. (1978). Centrality in social networks con-
ceptual clarification. Social Networks, 1(3):215 – 239.
Gronau, N. (2016). Handbuch der ERP-Auswahl, volume 2.
GITO mbH.
Gronau, N. and Theuer, H. (2016). Determination of the op-
timal degree of autonomy in a cyber-physical produc-
tion system. In Proceedings of 49th CIRP Conference
on Manufacturing Systems (CIRP-CMS 2016).
Hichert, R., Roos, A., Ziegler, J., Reichwald, R., M
¨
oslein,
K., Meitner, H., Schweiggert, F., and Ebersp
¨
acher, J.
(1996). Information und kommunikation in dezen-
tralen strukturen. In Bullinger, H.-J. and Warnecke,
H.-J., editors, Neue Organisationsformen im Un-
ternehmen, pages 657–752. Springer-Verlag.
Huber, W. (2018). Industrie 4.0 kompakt–Wie Tech-
nologien unsere Wirtschaft und unsere Unternehmen
ver
¨
andern. Springer.
Kluth, R. and Storr, A. (1997). Hohe produktivit
¨
at durch
werkergerechtes, situationsorientiertes informations-
management. In f
¨
ur Fertigungstechnik, G., editor,
FTK’97: Fertigungstechnisches Kolloquium, pages
325–349.
K
¨
ohler, A., L
¨
ammle, C., and Wiendahl, H.-H. (1997).
Dezentralisierung und vernetzung der produktionspla-
nung und -steuerung. In f
¨
ur Fertigungstechnik, G.,
editor, FTK’97: Fertigungstechnisches Kolloquium,
pages 199–217.
LSWI (2022). Center industry 4.0 potdam – a worldwide
unique opportunity for an encounter with the future.
Webseite.
Milberg, J. (1991). Wettbewerbsfaktor zeit in produktion-
sunternehmen. In Wettbewerbsfaktor Zeit in Produk-
tionsunternehmen, pages 11–31. Springer.
Mussbach-Winter, U. (1997). Dezentrale produktionspla-
nung und -steuerung. In f
¨
ur Fertigungstechnik, G.,
editor, FTK’97: Fertigungstechnisches Kolloquium,
pages 420–431.
Petschow, U., Ferdinand, J.-P., Diekel, S., and Fl
¨
amig, H.
(2014). Dezentrale produktion, 3d-druck und nach-
haltigkeit. Schriftenreihe des I
¨
OW, 206:14.
Ramsauer, C. (1997). Dezentrale PPS-Systeme Neue Struk-
turen bei hoher Innovationsdynamik. Gabler Verlag.
Sabidussi, G. (1966). The centrality index of a graph. Psy-
chometrika, 31(4):581–603.
Sundermeier, J., Gehlhoff, F., and Fay, A. (2020). Devel-
opment of a simulation model to analyze the perfor-
mance of decentral rescheduling algorithms in pro-
duction systems. Simul. Notes Eur., 30(1):15–22.
Ten Hompel, M. and Henke, M. (2014). Logistik 4.0. In
Industrie 4.0 in Produktion, Automatisierung und Lo-
gistik, pages 615–624. Springer.
Theuer, H. (2011). Extension of value stream design for
the simulation of autonomous production systems. In
ElMaraghy, H., editor, Proceedings of the 4th Inter-
national Conference on Changeable, Agile, Reconfig-
urable and Virtual production (CARV 2011), pages
586–591. Springer Verlag.
Theuer, H. (2018). Autonome akteure in der dezentralen
produktionssteuerung. Industrie 4.0 Management,
34(6):41–44.
Theuer, H. (2022). Communication in decentralized pro-
duction system (accepted). In Proceedings of 31rd
International Conference on Flexible Automation and
Intelligent Manufacturing.
Wang, Y., Anokhin, O., and Anderl, R. (2017). Concept
and use case driven approach for mapping it security
requirements on system assets and processes in indus-
trie 4.0. Procedia CIRP, 63:207–212.
Westk
¨
amper, E., Wiendahl, H.-H., and Balve, P. (1998).
Dezentralisierung und autonomie in der produktion.
ZWF – Zeitschrift f
¨
ur wirtschaftlichen Fabrikbetrieb,
9(93):407–410.
Windt, K. (2006). Selbststeuerung intelligenter objekte in
der logistik. In Vec, M., H
¨
utt, M., and Freund, A., ed-
itors, Selbstorganisation – Ein Denksystem f
¨
ur Natur
und Gesellschaft. B
¨
ohlau Verlag, K
¨
oln.
Windt, K. (2008). Ermittlung des angemessenen selbsts-
teuerungsgrades in der logistik – grenzen der selbst-
steuerung. In Nyhuis, P., editor, Beitr
¨
age zur Theo-
rie einer Logistik, pages 349 – 372. Springer-Verlag
Berlin Heidelberg.
Zeidler, F., M
¨
uller, D., Ten Hompel, M., and Henke, M.
(2019). Wip-balancing by autonomous control using
flexibility oriented decision-making. In Proceedings
of 48th International Conference on Computers and
Industrial Engineering, CIE.
IN4PL 2022 - 3rd International Conference on Innovative Intelligent Industrial Production and Logistics
74