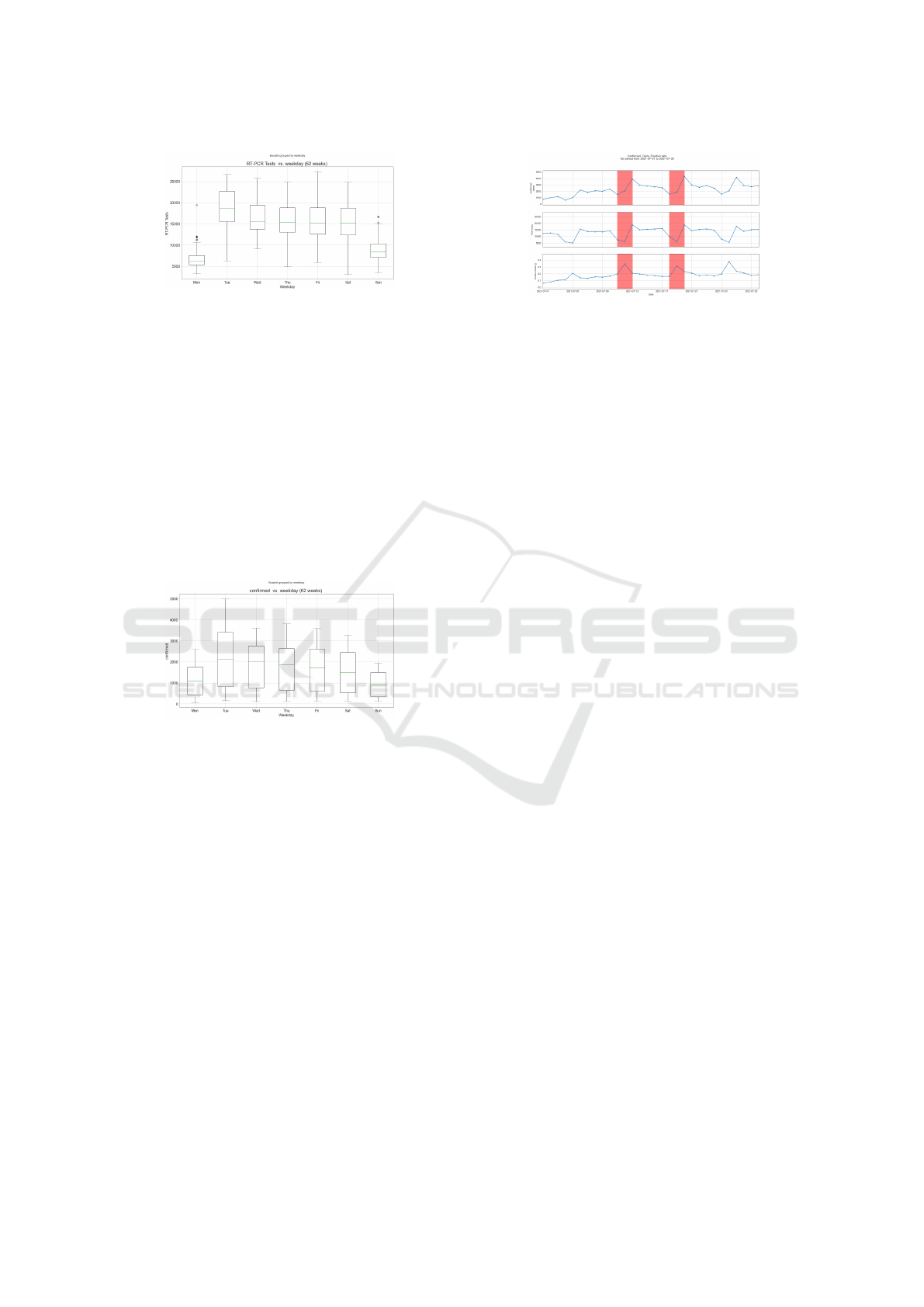
Figure 1: Absolute number of RT-PCR tests grouped per
weekday.
day have comparably lower max values. Also, the
100 percentile for Monday and Sunday is approxi-
mately equal or less than the median of the other
days). So, the reported number of tests in Greece is
usually greater at days following the working week-
days (Tuesday to Saturday), and falls on the days fol-
lowing weekend days (Sunday and Monday).
Figure 2 presents the absolute number of con-
firmed cases grouped per weekday. It can be seen
that Tuesday has the highest max value. Wednesday
to Saturday appear to have similar max values, while
Monday and Sunday appear to have similar max val-
ues which are lower than the rest.
Figure 2: Absolute number of confirmed cases grouped per
weekday.
Similarly to the pattern observed in tests, the ab-
solute number of confirmed cases is usually greater
in days following working weekdays, and falls during
the days following weekend days.
Figure 3 presents a representative part of the time
series for confirmed cases, RT-PCR tests and posi-
tive rate which corresponds to July of 2021. The red
shaded areas correspond to weekend, where the num-
ber of tests is smaller, and it can be seen that the pos-
itive rate increases significantly on Sundays.
1.2 Normalising Confirmed Cases and
Tests with Respect to Weekly
Average
When examining a quantity (e.g. the confirmed cases
or the number of tests) to determine the influence of
the weekdays, the absolute numbers contain two dif-
ferent sources of variability. I.e. any variation within
Figure 3: Detail of time series for confirmed cases, RT-PCR
tests and Positive rate, the red areas center around a Sunday.
the week (short term) and also the current state of the
pandemic which has a greater time scale (long term).
For example, if the confirmed cases are consid-
ered, then a value of 10 in a week when there are only
a few cases (e.g. 10) can have a totally different mean-
ing within the context of a week with a rash of cases
(e.g. 1000).
Therefore, in order to isolate the effect of the
weekday on the quantity, a normalisation is consid-
ered. Each daily value within a week is normalised
with respect to the week’s average. The aim of this
normalisation is to report the number of confirmed
cases relative to the week’s average. Reporting the
daily quantity as a percentage of the weeks average al-
lows a more meaningful intra-week comparison (i.e.
independently of the disease state). The duration of
the week is selected because it is considered as a time
unit.
The fact that the week can be considered a good
time unit can be evidenced by applying an Auto Cor-
relation Function (ACF) (Park, 2018), (Papoulis and
Pillai, 2002), (Box et al., 2015), (Percival, 1993), ,
or measure the Allan Variance (Allan, 1966),(Scharf
et al., 1995), (Percival and Mondal, 2012),(Lu et al.,
2013), (Malkin, 2012). In the ACF case, there is a
higher correlation value every 7th day (see Figure 4
top). The Allan variance shows that the minimum
standard deviation is when the samples are 6 days
apart (so every 7th day) — see Figure 4 bottom.
Figure 5 presents the tests per weekday nor-
malised with respect to the average tests for the cor-
responding week. On Monday and Sunday the cases
are significantly lower (less than 50%).
Figure 6 presents the confirmed cases per week-
day normalised with respect to the average of the cor-
responding week. It can be seen that on Monday
and Sunday the cases are significantly lower (close
to 50%)
From Figures 5 and 6 it can be seen that although
the normalised values of Sundays and Mondays are
lower than the other days, the median of the nor-
malised values are in general the same between tests
and confirmed cases. E.g. although the normalised
COVID-ex 2022 - Special Session on "COVID-19 epidemic data mining and EXploration"
778