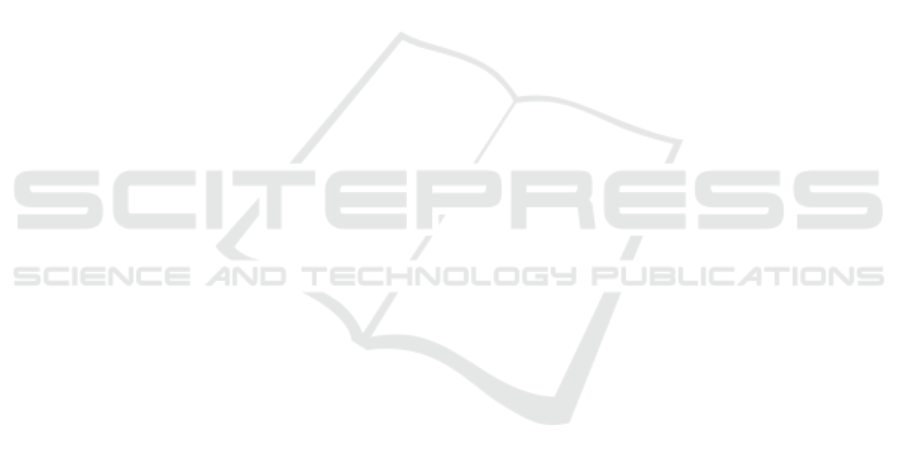
replicate experimentally known data, and how useful
they might be for better understanding of biological
mechanism driving circadian cycle rhythm.
The proposed models can be regarded as suc-
cessful at these aspects. The models 3 gene Circa-
dian3 and 5 gene Circadian5B core oscillator mod-
els demonstrate cyclic activity switching for all the
included genes as the only type of behaviour that is
allowed under assumptions of these models. More-
over, the models allow only strictly synchronised ac-
tivity oscillations for all the involved genes. Such re-
sult might be less surprising for Circadian3 model,
where the potential of cyclic oscillations of all the 3
genes can be deduced from gene regulation ’wiring
diagram’ (Figure 1), still the fact that oscillations in-
volving only 1 or 2 genes can be excluded by simple
priority constraints was not immediately obvious.
For 5 gene model we can derive a concrete pre-
diction regarding gene regulatory functions – namely,
that to obtain biologically feasible model behaviour
genes Per2 and Cry1 should act in collaboration for
their repressory activity. Such collaborative action of
these genes is well supported by biologically known
facts about circadian cycle gene regulatory mecha-
nism. The corresponding model Circadian5B also
predicts synchronised oscillation of all 5 genes of
core oscillator as the only behaviour that is possi-
ble according to the model. There are several dif-
ferent sequences of transition orders that can lead
to this behaviour, with one of them in reasonably
good agreement with simulation results obtained from
ODE based models. There is a also good potential to
refine this model further (i.e. by explicitly including
in the model several different binding site affinities
for the same protein, introducing more complex reg-
ulatory functions, or additional priority constraints),
provided that there are some experimental/biological
data supporting specific modifications.
In a broader context HSM models for circadian
cycle that we describe here are the first attempt to ap-
ply HSM framework for modelling gene regulation in
more complex organisms, since all the previous mod-
els have been designed for viruses. As a use case
of modelling more complex organisms our proposed
models appear to be successful. They also allow to
identify challenges and research priorities that need to
be considered for development of such type of mod-
els.
REFERENCES
Brazma, A., Cerans, K., Ruklisa, D., Schlitt, T., and Viksna,
J. (2015). Modeling and analysis of qualitative be-
havior of gene regulatory networks. Lecture Notes in
Computer Science, 7699:51–66.
Brazma, A. and Schlitt, T. (2003). Reverse engineering of
gene regulatory networks: a finite state linear model.
Genome Biol., 4:P5:1–31.
Brazma, R., Cerans, K., Ruklisa, D., Schlitt, T., and Viksna,
J. (2013). HSM – a hybrid system based approach for
modelling intracellular networks. Gene, 518:70–77.
Ko, C. and Takahashi, J. (2006). Molecular components
of the mammalian circadian clock. Human Molecular
Genetics, 15(S2):R271–R277.
Korencic, A., Kasir, R., Bordyugov, G., Lehmann, R., Roz-
man, D., and Herzel, H. (2014). Timing of circa-
dian genes in mammalian tissues. Scientific Reports,
4:5782.
Leloup, C. and Goldbetter, A. (2003). Toward a detailed
computational model for the mammalian circadian
clock. PNAS, 100:7051–7056.
Morris, A., Stanton, D., Roman, D., and Liu, A. (2020).
Systems level understanding of circadian integration
with cell physiology. J Mol. Biol., 432(12):3547–
3564.
Pett, J., Korencic, A., Wessener, F., and Herzel, H. (2016).
Feedback loops of the mammalian circadian clock
constitute repressilator. PLOS Comp. Biol., 12(12).
Relogio, A., Westermark, P., Wallach, T., Schellenberg, K.,
Kramer, A., and Herzel, H. (2011). Tuning the mam-
malian circadian clock: robust synergy of two loops.
PLOS Comp. Biol., 7(12).
Ruklisa, D., Brazma, A., Cerans, K., T., S., and Viksna, J.
(2019). Dynamics of gene regulatory networks and
their dependence on network topology and quantita-
tive parameters – the case of phage lambda. BMC
Bioinformatics, 20:296.
Schlitt, T. and Brazma, A. (2006). Modelling in molec-
ular biology: describing transcription regulatory net-
works at different scales. Philos. Trans. R. Soc. Lond.
B, 361:483–494.
Schmal, C., Ono, D., Myung, J., Pett, J., Honma, S., and
Herzel, H. (2019). Weak coupling between intracellu-
lar feedback loops explains dissociation of clock gene
dynamics. PLOS Comp. Biol., 15(9):e1007330.
Ueda, H., Hayashi, S., Chen, W., Sano, M., Machida, M.,
Shigeyoshi, Y., Iino, M., and Hashimoto, S. (2005).
System-level identification of transcriptional circuits
underlying mammalian circadian clocks. Nature Ge-
netics, 37(2):187–192.
Ukai, H. and Ueda, H. (2010). Systems biology of
mammalian circadian clocks. Ann. Rev. Physiology,
72(1):579–603.
Yoshitane, H., Asano, Y., Sagami, A., Sakai, S., Suzuki, Y.,
Okamura, H., Iwasaki, W., Ozaki, H., and Fukada, Y.
(2015). Functional D-box sequences reset the circa-
dian clock and drive mRNA rhythms. Communica-
tions Biology, 2(1):300.
Hybrid Gene Regulation Models of Mammalian Circadian Cycles
137