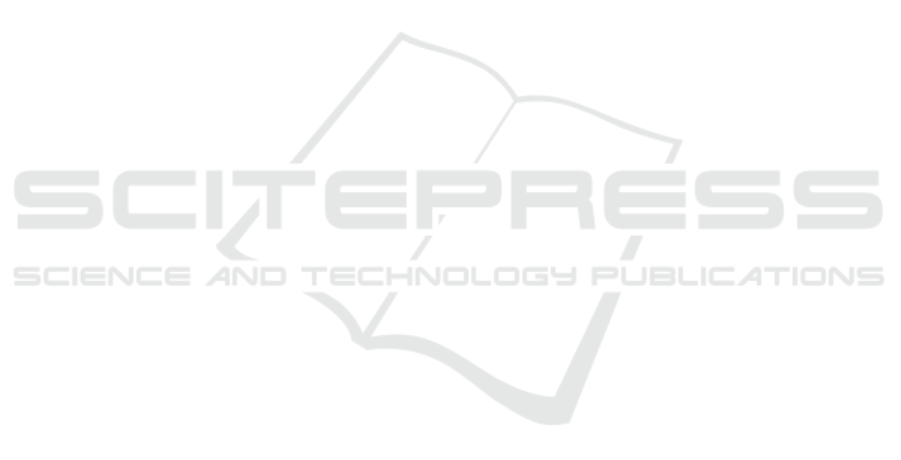
REFERENCES
Association, A. L. et al. (1989). American li-
brary association presidential committee
on information literacy. http://www. ala.
org/ala/acrl/acrlpubs/whitepapers/presidential.
htm.
Banchs, R. E. (2016). Expert-generated vs. crowd-sourced
annotations for evaluating chatting sessions at the turn
level. In WOCHAT: Second Work-shop on Chatbots
and Conversational Agent Technologies, IVA 2016.
Braccini, A. M. and Federici, T. (2013). A measurement
model for investigating digital natives and their organ-
isational behaviour.
Bruun, A. and Stage, J. (2015). New approaches to us-
ability evaluation in software development: Barefoot
and crowdsourcing. Journal of Systems and Software,
105:40–53.
Cao, Y., Sanchez Carmona, V. I., Liu, X., Hu, C., Iskender,
N., Beyer, A., M
¨
oller, S., and Polzehl, T. (2021). On
the impact of self-efficacy on assessment of user ex-
perience in customer service chatbot conversations. In
IWSDS 2021.
Deriu, J., Rodrigo, A., Otegi, A., Echegoyen, G., Rosset,
S., Agirre, E., and Cieliebak, M. (2021). Survey on
evaluation methods for dialogue systems. Artificial
Intelligence Review, 54(1):755–810.
Devlin, J., Chang, M.-W., Lee, K., and Toutanova, K.
(2018). Bert: Pre-training of deep bidirectional trans-
formers for language understanding. arXiv preprint
arXiv:1810.04805.
Finstad, K. (2010). The usability metric for user experience.
Interacting with Computers, 22(5):323–327.
Geyer, R. W. (2009). Developing the internet-savviness (is)
scale: Investigating the relationships between internet
use and academically talented middle school youth.
RMLE Online, 32(5):1–20.
Gomide, V. H., Valle, P. A., Ferreira, J. O., Barbosa, J. R.,
Da Rocha, A. F., and Barbosa, T. (2014). Affective
crowdsourcing applied to usability testing. Interna-
tional Journal of Computer Scienceand Information
Technologies, 5(1):575–579.
Hillmann, S. (2017). Simulation-Based Usability Evalua-
tion of Spoken and Multimodal Dialogue Systems. T-
Labs Series in Telecommunication Services. Springer
International Publishing, Cham.
Hillmann, S. and Engelbrecht, K.-P. (2015). Modelling
Goal Modifications in User Simulation. In Future
and Emerging Trends in Language Technology, vol-
ume 9577 of LNAI, pages 149–159, Sevilla, Spain.
Hoßfeld, T., Keimel, C., Hirth, M., Gardlo, B., Habigt,
J., Diepold, K., and Tran-Gia, P. (2013). Best prac-
tices for qoe crowdtesting: Qoe assessment with
crowdsourcing. IEEE Transactions on Multimedia,
16(2):541–558.
Iskender, N., Polzehl, T., and M
¨
oller, S. (2020a). Crowd-
sourcing versus the laboratory: towards crowd-based
linguistic text quality assessment of query-based ex-
tractive summarization. In Proc. of the Conference on
Digital Curation Technologies (Qurator 2020), pages
1–16. CEUR.
Iskender, N., Polzehl, T., and M
¨
oller, S. (2020b). Towards a
reliable and robust methodology for crowd-based sub-
jective quality assessment of query-based extractive
text summarization. In Proceedings of the 12th LREC,
pages 245–253. European Language Resources Asso-
ciation.
ISO (2010). Ergonomics of human system interaction-part
210: Human-centred design for interactive systems
(formerly known as 13407). Standard ISO DIS 9241-
210, International Organization for Standardization,
Switzerland.
ITU-T (2003). Subjective quality evaluation of telephone
services based on spoken dialogue systems. ITU-T
Rec. P.851, International Telecommunication Union,
Geneva.
Jain, A., Pecune, F., Matsuyama, Y., and Cassell, J. (2018).
A user simulator architecture for socially-aware con-
versational agents. IVA ’18, page 133–140, New
York, NY, USA. Association for Computing Machin-
ery.
Jurc
´
ıcek, F., Keizer, S., Gasic, M., Mairesse, F., Thomson,
B., Yu, K., and Young, S. (2011). Real user evaluation
of spoken dialogue systems using amazon mechanical
turk. pages 3061–3064.
Kittur, A., Chi, E., and Suh, B. (2008). Crowdsourcing for
usability: Using micro-task markets for rapid, remote,
and low-cost user measurements. Proc. CHI 2008.
Lai, A. (2016). The rise of the empowered customer. Tech-
nical report, Forrester Research, Inc., 60 Acorn Park
Drive, Cambridge, MA 02140 USA.
Law, E. L.-C., Roto, V., Hassenzahl, M., Vermeeren, A. P.,
and Kort, J. (2009). Understanding, scoping and defin-
ing user experience: a survey approach. In Proceed-
ings of the SIGCHI conference on human factors in
computing systems, pages 719–728.
Liu, D., Bias, R. G., Lease, M., and Kuipers, R. (2012).
Crowdsourcing for usability testing. Proceedings of
the American Society for Information Science and
Technology, 49(1):1–10.
M
¨
oller, S., Smeele, P., Boland, H., and Krebber, J. (2007).
Evaluating spoken dialogue systems according to de-
facto standards: A case study. Computer Speech &
Language, 21(1):26–53.
Nebeling, M., Speicher, M., and Norrie, M. C. (2013).
Crowdstudy: General toolkit for crowdsourced eval-
uation of web interfaces. In Proceedings of the 5th
ACM SIGCHI symposium on Engineering interactive
computing systems, pages 255–264.
Owen, T. (2003). Chartered institute of library and infor-
mation professionals. Encyclopedia of Library and
Information Science, 490:499.
Pfeiffer, J., Kamath, A., R
¨
uckl
´
e, A., Cho, K., and Gurevych,
I. (2020). Adapterfusion: Non-destructive task
composition for transfer learning. arXiv preprint
arXiv:2005.00247.
Polzehl, T. (2014). Personality in Speech - Assessment and
Automatic Classification. T-Labs Series in Telecom-
munication Services. Springer.
HUCAPP 2022 - 6th International Conference on Human Computer Interaction Theory and Applications
46