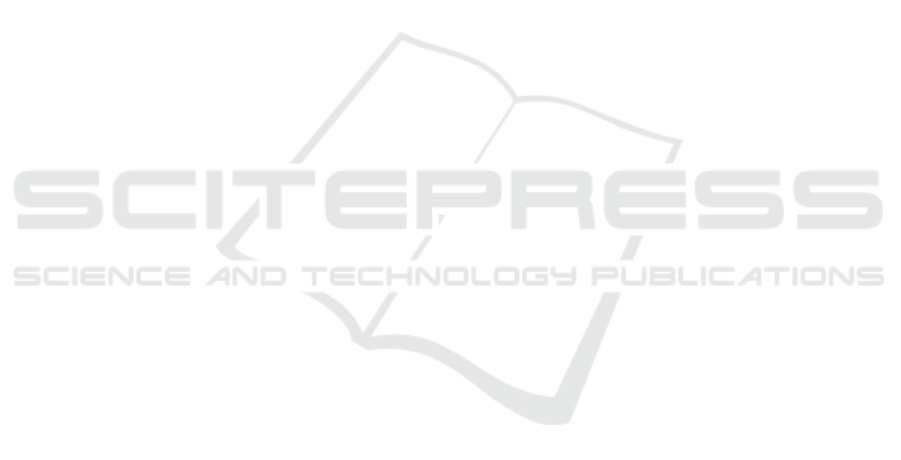
IoHT studies, mainly focused on the elderly. In gen-
eral, there is still room for more partnerships with the
industry to perform validations in practice. Finally,
several solutions for monitoring and diagnosing dis-
eases have been proposed, but an increase in machine
learning solutions for early diagnosis is expected.
Regarding the challenges, there is a desire for per-
sonalized and intelligent services that provide contin-
uous, fast, secure, and effective health data monitor-
ing. Regarding our last research question, the results
show that few studies seek a general approach to deal-
ing with QoL, and the works closer to this proposal
still use questionnaires to collect data. Probably, this
happens due to the difficulty in developing this kind of
approach since a large amount of user data is needed,
in addition to the validation complexity, which is done
through longitudinal studies. Thus, this question can
validate the beginning of a more in-depth investiga-
tion towards a semantic structure of the QoL domains
and facets and an intelligent model for capturing and
inferring this metric.
Concerning the gaps, we realized a need for
collaborative-aware and ubiquitous services able to
anticipate events and to act in the environment to im-
prove the living conditions; partnerships with the in-
dustry to conduct validations in practice; methods and
protocols capable of guaranteeing data security and
privacy even on restricted devices; network designs
to ensure low latency and high reliability; techniques
for validation and verification of fitness tracking apps;
approaches to the development of intelligent systems
integrated with the domain experts; and studies fo-
cused on user experience.
DATA AVAILABILITY
Our data are public. It is also important to highlight
that the papers selected in this mapping were linked
using the study ID due to space restrictions.
- Protocol: bit.ly/3B6jqth
- Selected Papers: /bit.ly/3FeXzCr
- Images (higher resolution): bit.ly/3D3nP0Q
- Raw Data (codes and tables): bit.ly/3Fe79FV
REFERENCES
Al-Fuqaha, A., Guizani, M., Mohammadi, M., Aledhari,
M., and Ayyash, M. (2015). Internet of things: A
survey on enabling technologies, protocols, and ap-
plications. IEEE communications surveys & tutorials,
17(4):2347–2376.
Araujo, I. L., Andrade, R. M. C., Costa Junior, E., Oliveira,
P. A. M., Oliveira, B. S. ., and Aguilar, P. A. (2020).
Lessons learned from the development of mobile ap-
plications for fall detection. In GLOBAL HEALTH
2020: The Ninth International Conference on Global
Health Challenges (GLOBAL HEALTH 2020).
Blei, D. M., Ng, A. Y., and Jordan, M. I. (2003). Latent
dirichlet allocation. Journal of machine Learning re-
search, 3(Jan):993–1022.
Breivold, H. P. (2017). Internet-of-things and cloud com-
puting for smart industry: A systematic mapping
study. In 2017 5th International Conference on En-
terprise Systems (ES), pages 299–304. IEEE.
Islam, S. R., Kwak, D., Kabir, M. H., Hossain, M., and
Kwak, K.-S. (2015). The internet of things for health
care: a comprehensive survey. IEEE Access.
Oliveira, P. A. M., Andrade, R. M., and Neto, P. A. S.
(2021). Iot-health platform to monitor and improve
quality of life in smart environments. In 2021 IEEE
45th Annual Computers, Software, and Applications
Conference (COMPSAC), pages 1909–1912. IEEE.
Pai, M., McCulloch, M., Gorman, J. D., Pai, N., Enanoria,
W., Kennedy, G., Tharyan, P., and Colford Jr, J. M.
(2004). Systematic reviews and meta-analyses: an il-
lustrated, step-by-step guide. The National medical
journal of India, 17(2):86–95.
Petersen, K., Feldt, R., Mujtaba, S., and Mattsson, M.
(2008). Systematic mapping studies in software en-
gineering. In 12th International Conference on Eval-
uation and Assessment in Software Engineering.
Rodrigues, J. J., Segundo, D. B. D. R., Junqueira, H. A.,
Sabino, M. H., Prince, R. M., Al-Muhtadi, J., and
De Albuquerque, V. H. C. (2018). Enabling technolo-
gies for the internet of health things. IEEE Access,
6:13129–13141.
Sanchez, W., Martinez, A., Campos, W., Estrada, H., and
Pelechano, V. (2015). Inferring loneliness levels in
older adults from smartphones. Journal of Ambient
Intelligence and Smart Environments, 7(1):85–98.
WHO (1998). Whoqol: Measuring quality of life.
https://www.who.int/healthinfo/survey/whoqol-
qualityoflife/en/index3.html. Accessed on 11/5/2020.
WHOQoL Group (1994). The development of the world
health organization quality of life assessment instru-
ment (the whoqol). In Quality of life assessment: In-
ternational perspectives, pages 41–57. Springer.
Wieringa, R., Maiden, N., Mead, N., and Rolland, C.
(2006). Requirements engineering paper classification
and evaluation criteria: a proposal and a discussion.
Requirements engineering, 11(1):102–107.
Wohlin, C., Runeson, P., H
¨
ost, M., Ohlsson, M. C., Reg-
nell, B., and Wessl
´
en, A. (2012). Experimentation in
software engineering. Springer Science.
HEALTHINF 2022 - 15th International Conference on Health Informatics
404