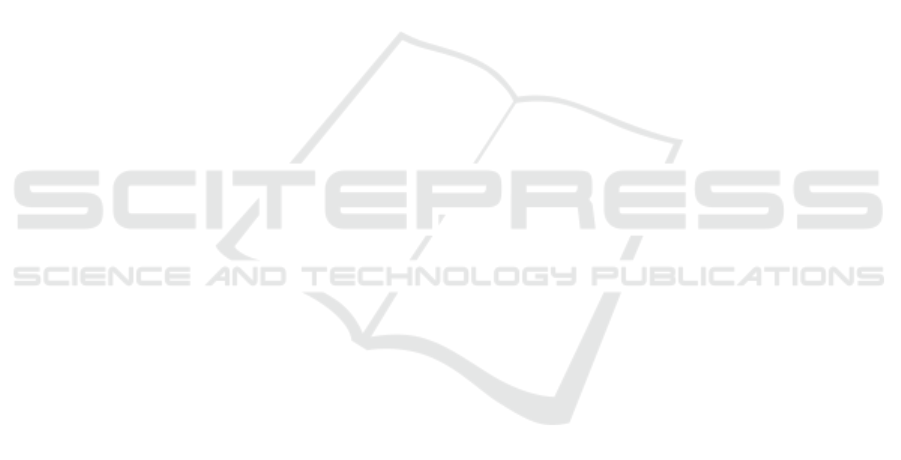
1992), there appeared many serious works in which
concept lattices were used. Concept analysis has
found a very wide range of applications in medical
research and teaching of medical students (see, e.g.
(Hu et al., 2019), (Keller et al., 2012), (Liu et al.,
2010), (Yazdani and Hoseini, 2018)), in particlar in
the process of basic studies of nurses (see e.g. (Rod-
hers et al., 2018)). Methods of concept analysis are
used also in the research of problems related to bi-
ology (Raza, 2017), (Hashikami et al., 2013), geol-
ogy (B
˘
elohl
´
avek, 2004, p. 214), social type prob-
lems (Missaoui et al., 2017), software engineering
(Tonella, 2004) and in other applied sciences. On the
other hand, we found only a few works, where fuzzy
concept analysis is used in the research of any practi-
cal problems. In our opinion, the problem to use the
fuzzy concept lattices in case when the scale value L
is continuous (like L = [0, 1]) or lattice having many,
probably incomparable elements, is that the request
in the concept analysis of the precise correspondence
between a fuzzy set A of objects and a fuzzy set B
of attributes in ”real-world” situations is (almost) im-
practicable. In this case one sooner has to deal with
the weaker request asking that the correspondence be-
tween A and B must hold up to a certain degree. In
order to provide a theoretical basis for the research in
this situation, in (
ˇ
Sostak et al., 2021), we introduced
the notion of a graded preconcept lattice, more flex-
ible than the notion of a concept lattice and initiated
the study of the properties of graded preconcept lat-
tices. In this paper we provide a further insight in the
practical application of the theoretical models in the
assessment of risks related to spread of pandemic cri-
sis.
This paper is organized as follows. In the second
section we briefly remind the notions related to
lattices, residuated lattices or quantales, fuzzy sets
and fuzzy relations. Besides, we remind the concept
of a fuzzy inclusion of fuzzy sets that lies in the base
of gradation of fuzzy preconcepts. In the third section
we define fuzzy preconcepts, introduce partial order
relation on the family of all fuzzy preconcepts of
a given fuzzy context (X ,Y, L, R) and show that the
resulting structure (P(X ,Y, L, R), ) is a lattice. Fur-
ther in this section we propose a method allowing to
determine the grade of the distinction of a fuzzy pre-
concept from “being a real fuzzy concept” and study
the corresponding graded preconcept lattices. In the
fourth section we explain the possible application of
fuzzy preconcept lattices in the analysis of pandemic
scenario and proceed with practical calculations in
modelling the spread of Covid-19 pandemics. In the
last, conclusion section, we briefly summarize main
results obtained and survey some directions for
the future work.
2 BACKGROUND
2.1 Lattices, Quantales and Residuated
Lattices
We use the standard terminology accepted in the-
ory of lattices, see, e.g. (Birkhoff, 1995), (Gierz et
al., 2003), (Morgan and Dilworth, 1939) for details.
For the reader convenience, we make clarification of
some, possibly less known terms.
A complete lattice L = (L, ≤, ∧, ∨) is called in-
finitely distributive if a ∧ (
W
i∈I
b
i
) =
W
i∈I
(a ∧ b
i
) for
every a ∈ L and every {b
i
| i ∈ I} ⊆ L. A com-
plete lattice L is called infinitely co-distributive if
a ∨ (
V
i∈I
b
i
) =
V
i∈I
(a ∨ b
i
) for every a ∈ L and every
{b
i
| i ∈ I} ⊆ L. A complete lattice is called infinitely
bi-distributive if it is infinitely and co-infinitely dis-
tributive. It is known, that every completely distribu-
tive lattice is infinitely bi-distributive.
Let L be a complete lattice and ∗ : L × L → L be a
binary associative commutative monotone operation.
Then the tuple (L, ≤, ∧, ∨, ∗) is called a (commuta-
tive) quantale (Rosenthal, 1990) if ∗ distributes over
arbitrary joins: a ∗ (
W
i∈I
b
i
) =
W
i∈I
(a ∗ b
i
).
A quantale is called integral if the top element 1
acts as the unit, that is 1∗a = a∗1 = a for every a ∈ L.
In what follows saying quantale we mean a com-
mutative integral quantale. A typical example of a
quantale is the unit interval endowed with a lower
semi-continuous t-norm, see, e.g. (Klement et al.,
2000).
In a quantale a further binary operation 7→: L ×
L → L, the residuum, can be introduced as associ-
ated with operation ∗ of the quantale (L, ≤, ∧, ∨, ∗)
via the Galois connection, that is a ∗ b ≤ c ⇐⇒ a ≤
b 7→ c for all a, b, c ∈ L. A quantale (L, ≤, ∧, ∨, ∗)
provided with the derived operation 7→, that is the tu-
ple (L, ≤, ∧, ∨, ∗, 7→), is known also as a (complete)
residuated lattice (Morgan and Dilworth, 1939).
2.2 Fuzzy Sets and Fuzzy Relations
Given a set X, its L-fuzzy subset is a mapping A : X →
L. The lattice and the quantale structure of L is ex-
tended point-wise to the L-exponent of X, that is to
the set L
X
of all L-fuzzy subsets of X. Specifically,
the union and intersection of a family of L-fuzzy sets
{A
i
|i ∈ I} ⊆ L are defined by their join
W
i∈I
A
i
and
FCTA 2021 - 13th International Conference on Fuzzy Computation Theory and Applications
178