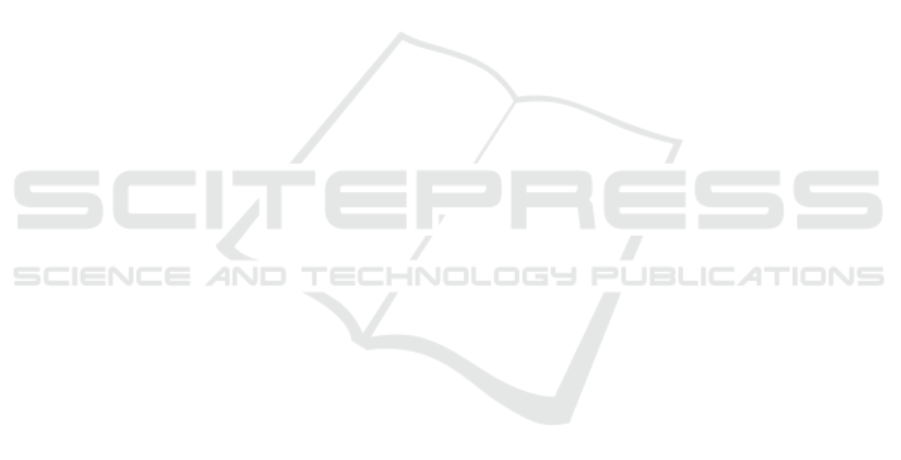
synthesised speech that poses a threat to the personal
security of Internet users.
Many websites and communities in social
networks can use deep fake audios to glorify fascism
and nationalism, xenophobia, religious extremism,
promote ideological terrorism, popularize ‘suicide
clubs’; promote drugs, underground culture, the cult
of violence and cruelty; incite hatred and enmity,
humiliate people on the grounds of their social
affiliation; harassment and bullying, slander and
insults, spread fakes, child pornography and other
prohibited information (Galyashina and Nikishin,
2020).
To expose voice fakes, prosecute the attacker, and
protect innocent people whose voices are illegally
used to commit speech offenses, it is necessary to
equip law enforcement agencies with an objective
criteria that allow them to detect, identify and prevent
the illegal use of other people's voices to commit
crimes in the digital media environment (Galyashina,
2021).
3 DISCUSSION OF RESEARCH
RESULTS
Our research showed that a significant part of the
disseminated criminal information is expressed
verbally. Fake audios are designed mainly for the
average user’s perception. The difference between an
AI generated voice clone and the real speaker sample
can be detected by professionally trained expert-
listener. The main distinctive features are reflected in
the prosodic structure of speech as well as in
discursive and intellectual speech skills that are
difficult to be imitated (Ladd, 1996). Thus, the first
theoretical conclusion is that special phonetic-
linguistic knowledge and integrated approach to
speaker identification is needed in order to expose
faked audio and detect the illegally used cloned
voices (Galyashina, 2015).
The second conclusion is connected with the
determination of security risks of AI generated speech
as these issues have not received sufficient attention
from law enforcements. It became obvious that the
processes taking place in the information space lead
to the emergence of new speech communication
phenomena that are represented by unpermitted use
of personal voice and speech ideotype that is also not
reflected in the legislation. We propose to supplement
the current legislation of Article 151.1. of the Civil
Code of the Russian Federation with the norm on the
protection of not only the image but also the voice of
a citizen (Article 151.1). The publication and further
use of recordings of a citizen's voice and speech are
allowed only with the consent of this citizen. After the
death of a citizen, their voice samples can only be
used with the consent of the children and a spouse or
with the consent of parents. Such consent is not
required in cases where: 1) the use of the voice sample
is carried out in the state, public or other public
interests; 2) the voice sample of a citizen is obtained
when audio recording is carried out in places open to
the public, or at public events (meetings, congresses,
conferences, concerts, performances, sports
competitions and similar events), except in cases
where such a voice sample is the main object of use;
3) a citizen’s voice sample is recorded for a fee.
The audio recordings of the citizen’s voice,
obtained or used in violation of the above-mentioned
conditions, shall be removed on the basis of a court
decision.
If a recording with a citizen’s voice sample
obtained or used in violation of the above-mentioned
conditions is distributed on the Internet, the citizen
has the right to demand the removal of this voice
recording as well as the prohibition of its further
distribution.
It is worth noting that until now, there has been no
holistic approach to the analysis of fake audio threats
arising in the digital media environment, the
development of measures to prevent and counteract
the spread of destructive information produced by
synthetic voice audibly undistinguished from the
voice of the real person.
All of this leads to the problem of qualitative
changes in the voice during the implementation of
measures for the comprehensive protection of speech
and voice as biometric data.
This is quite a difficult task, since each person's
voice is individual and recognizable (Yarmey, 2001).
Moreover, the trained auditory perception helps to
identify the most subtle shades of the speech signal.
The average human hearing is not accurate in
detecting the signs of artificiality or naturalness of AI
generated speech. Therefore, in order to solve the
problem of fake voice detecting with the preservation
the individual features of a natural sound according to
a given voice sample, it is necessary to dwell in more
detail on the concept of AI generated speech and its
main features, to systemize factors that determine
audial differentiation of real and faked voices.
The main factor is associated with the acoustic-
phonetic structure, that is, with the prosodic similarity
of the sound of the AI synthesized speech signal with
natural speech. Speech signals can be considered as a
physical implementation of a complex hierarchically
AI Generated Fake Audio as a New Threat to Information Security: Legal and Forensic Aspects
19