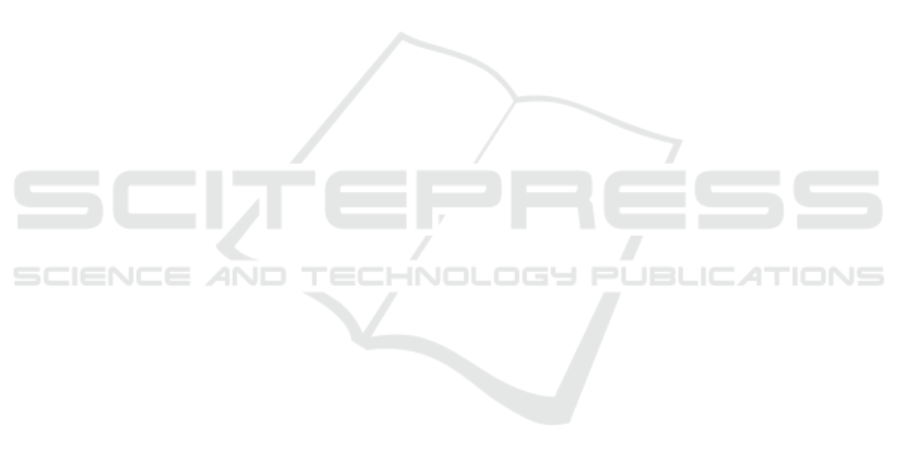
Huion, A., Decalf, V., Kumps, C., Witte, N. D., and Ev-
eraert, K. (2019). Smart diapers for nursing home
residents with dementia: a pilot study. Acta Clinica
Belgica, 74(4):258–262.
Katz, S., Ford, A. B., Moskowitz, R. W., Jackson, B. A.,
and Jaffe, M. W. (1963). Studies of Illness in the
Aged: The Index of ADL: A Standardized Measure
of Biological and Psychosocial Function. JAMA,
185(12):914–919.
Lin, S. H., Kajiyama, K., and Wu, T. (2017). Smart Di-
aper: How It Works. In Proceedings of the 2017
ACM International Joint Conference on Pervasive and
Ubiquitous Computing and Proceedings of the 2017
ACM International Symposium on Wearable Comput-
ers, UbiComp ’17, pages 129–132, New York, NY,
USA. ACM. event-place: Maui, Hawaii.
Mather, K. F. and Bakas, T. (2002). Nursing assistants’
perceptions of their ability to provide continence care.
Geriatric Nursing, 23(2):76–81.
O’Dell, Katharine, K., Jacelon, C., and Morse, A. N.
(2008). ’I’d Rather Just Go On as I Am’ - Pelvic Floor
Care Preferences Of Frail, Elderly Women in Residen-
tial Care. Urologic Nursing, 28(1):36–47.
Offermans, M. P. W., Moulin, M. F. M. T. D., Hamers, J.
P. H., Dassen, T., and Halfens, R. J. G. (2009). Preva-
lence of urinary incontinence and associated risk fac-
tors in nursing home residents: A systematic review.
Neurourology and Urodynamics, 28(4):288–294.
Ouslander, J. G., Buxton, W. G., Al-Samarrai, N. R., Cruise,
P. A., Alessi, C., and Schnelle, J. F. (1998). Nighttime
Urinary Incontinence and Sleep Disruption Among
Nursing Home Residents. Journal of the American
Geriatrics Society, 46(4):463–466.
Ouslander, J. G. and Kane, R. L. (1984). The Costs of Uri-
nary Incontinence in Nursing Homes. Medical Care,
22(1):69–79.
Plotz, T., Chen, C., Hammerla, N. Y., and Abowd,
G. D. (2012). Automatic Synchronization of Wear-
able Sensors and Video-Cameras for Ground Truth
Annotation–A Practical Approach. pages 100–103.
IEEE.
Rantz, M., Skubic, M., Alexander, G., Aud, M., Wake-
field, B., Galambos, C., Koopman, R., and Miller, S.
(2010a). Improving Nurse Care Coordination With
Technology. Computers, informatics, nursing : CIN,
28:325–32.
Rantz, M., Skubic, M., Alexander, G., Popescu, M., A Aud,
M., J Wakefield, B., Koopman, R., and J Miller, S.
(2010b). Developing a Comprehensive Electronic
Health Record to Enhance Nursing Care Coordina-
tion, Use of Technology, and Research. Journal of
gerontological nursing, 36:13–7.
Strauven, H., Hallez, H., Vanden Abeele, V., and Vanrum-
ste, b. (2019). The Design and Development of an
Ambient Sensor System to Detect Incontinence.
Suzuki, R., Otake, S., Izutsu, T., Yoshida, M., and Iwaya,
T. (2006). Monitoring Daily Living Activities of
Elderly People in a Nursing Home Using an In-
frared Motion-Detection System. Telemedicine and
e-Health, 12(2):146–155.
T’Jonck, K., Strauven, H., Vankeirsbilck, J., Hallez, H., and
Boydens, J. (2019). Design and implementation of
an unobtrusive sensor system to support incontinence
care of elderly in nursing homes. Sozopol, Bulgaria.
Traynor, V., Yu, P., Hailey, D., and Fleming, R. (2014). An
exploration of the effects of introducing a telemoni-
toring system for continence assessment in a nursing
home. Journal of Clinical Nursing, 23:3069–3076.
Wagg, A., Kung Chen, L., Johnson II, T., Kirschner-
Hermanns, R., Kuchel, G., Markland, A., Orme, S.,
Ostaszkiewicz, J., Szonyi, G., and Wyman, J. (2017).
Incontinence in frail older persons. Incontinence : 6th
International Consultation on Incontinence, Tokyo,
September 2016, pages 1309–1442.
Wai, A. A. P., Foo, S. F., Jayachandran, M., Biswas, J., Nu-
gent, C., Mulvenna, M., Zhang, D., Craig, D., Pass-
more, P., and Lee, J.-E. (2010a). Towards develop-
ing effective Continence Management through wet-
ness alert diaper: Experiences, lessons learned, chal-
lenges and future directions. pages 1–8. IEEE.
Wai, A. A. P., Foo, S. F. V., Jayachandran, M., Biswas, J.,
Nugent, C., Mulvenna, M., Zhang, D., Craig, D., Pass-
more, P., and Lee, J.-E. (2010b). Technical develop-
ment and clinical evaluation of intelligent continence
management system at nursing home. pages 345–352.
IEEE.
Wai, A. A. P., Fook, F. S., Jayachandran, M., Song, Z.,
Biswas, J., Nugent, C., Mulvenna, M., Lee, J.-E., and
Yap, L. K. P. (2008). Smart wireless continence man-
agement system for elderly with dementia. pages 33–
34. IEEE.
Wai, A. P., Foo, S., Biswas, J., Donnelly, M., Parente, G.,
Nugent, C., and Yap, P. (2011). Smart phone reminder
system for managing incontinence at nursing home.
pages 254–259. IEEE.
Wilson, L., Brown, J. S., Shin, G. P., Luc, K.-O., and Subak,
L. L. (2001). Annual direct cost of urinary inconti-
nence. Obstetrics & Gynecology, 98(3):398–406.
HEALTHINF 2020 - 13th International Conference on Health Informatics
446