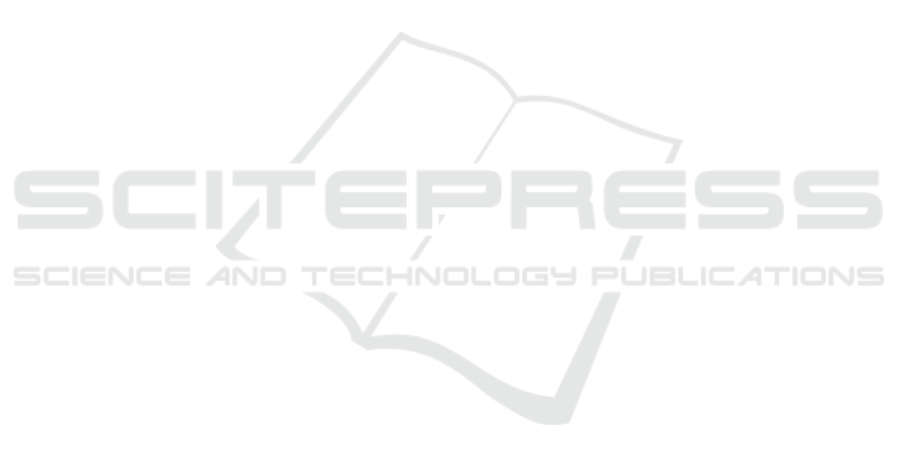
Following all given five steps after the application of
this measurement model, a company gets a structured
overview of their more and less knowledge-intensive
business processes to get an idea with which pro-
cesses to start the improvements according to any pro-
cess management initiatives or the application of a
quality management norm.
7 LIMITATION AND OUTLOOK
There is one obvious limitation to this presented
study: the missing application of the measurement
model in practice. The author tried to implement all
the necessary knowledge from practitioners and com-
bined it with a scientific background, but there is a
lack of practical evidence which should be improved
by the next step in this research project.
The next goal is to specify the lack of calibration
of the factors/restrictions and to substantiate this uti-
lizing additional studies. In this context, the diagnos-
tic model should be applied in companies, and a sec-
ond measurement should be carried out using other
methods. The two results can then be compared with
each other, and new insights can be gained to improve
the measurement ability and prove the whole concept.
REFERENCES
Allweyer, T. (1998). Modellbasiertes wissensmanagement.
Information Management, 13(1):37–45.
Carroll, J. M. and Swatman, P. A. (2000). Structured-case:
a methodological framework for building theory in in-
formation systems research. European journal of in-
formation systems, 9(4):235–242.
Davenport, T. and Prusak, L. (2000). Working knowledge:
How organizations manage what they know. Ubiquity,
2000(August):6.
Delahaye, B. (2003). Knowledge management in an sme.
International Journal of Organisational Behaviour,
9(3):604–614.
Desouza, K. C. and Awazu, Y. (2006). Knowledge manage-
ment at smes: five peculiarities. Journal of knowledge
management.
Dumas, M., La Rosa, M., Mendling, J., and Reijers, H. A.
(2013). Business process management. Springer.
Dunkelberg, W. and Wade, H. (2007). Overview–small
business optimism. Small Business Economic Trends,
pages 1–21.
Durst, S. and Edvardsson, I. (2012). Knowledge manage-
ment in smes: a literature review. Journal of Knowl-
edge Management.
Edwards, J. S. and Kidd, J. B. (2003). Bridging the gap
from the general to the specific by linking knowledge
management to business processes. In Knowledge and
business process management, pages 118–136. IGI
Global.
Eppler, M. J., Seifried, P., and R
¨
opnack, A. (2008). Improv-
ing knowledge intensive processes through an enter-
prise knowledge medium (1999). In Kommunikation-
smanagement im Wandel, pages 371–389. Springer.
Goesmann, T. and Hoffmann, M. (2000). Unterst
¨
utzung
wissensintensiver gesch
¨
aftsprozesse durch workflow-
management-systeme. Verteiltes Arbeiten–Arbeit der
Zukunft. Tagungsband der D-CSCW 2000.
Gronau, N., M
¨
uller, C., and Korf, R. (2005). Kmdl-
capturing, analysing and improving knowledge-
intensive business processes. J. UCS, 11(4):452–472.
Heinrich, L. J., Heinzl, A., and Roithmayr, F. (2014).
Wirtschaftsinformatik Lexikon. Walter de Gruyter
GmbH & Co KG.
Helfferich, C. (2011). Die qualit
¨
at qualitativer daten.
Maier, R. and Thalmann, S. (2007). Describing learning
objects for situation-oriented knowledge management
applications. In 4th Conference on Professional Kon-
wledge Management Experiences and Visions, vol-
ume 2, pages 343–351.
McAdam, R. and Reid, R. (2001). Sme and large organisa-
tion perceptions of knowledge management: compar-
isons and contrasts. Journal of knowledge manage-
ment.
Mentzas, G., Apostolou, D., Young, R., and Abecker, A.
(2001). Knowledge networking: a holistic solution for
leveraging corporate knowledge. Journal of knowl-
edge management.
Mertins, K., Heisig, P., Vorbeck, J., Mertins, K., Heisig,
P., and Vorbeck, J. (2001). Knowledge management:
Best practices in europe.
Nonaka, I. and Takeuchi, H. (1998). A theory of the firm’s
knowledge-creation dynamics.
Oesterle, H. and Winter, R. (2000). Business engineering.
In Business Engineering, pages 3–20. Springer.
Ploder, C. and Kohlegger, M. (2018). A model for data
analysis in smes based on process importance. In In-
ternational Conference on Knowledge Management in
Organizations, pages 26–35. Springer.
Probst, G., Raub, S., and Romhardt, K. (2006). Wissen man-
agen. Springer.
Remus, U. (2002). Prozessorientiertes Wissensmanage-
ment. Konzepte und Modellierung. PhD thesis.
Richter-von Hagen, C., Ratz, D., and Povalej, R. (2005). A
genetic algorithm approach to self-organizing knowl-
edge intensive processes. In Proceedings of I-KNOW,
volume 5. Citeseer.
Saloj
¨
arvi, S., Furu, P., and Sveiby, K.-E. (2005). Knowledge
management and growth in finnish smes. Journal of
knowledge management.
Senge, P. M. (1996). Die fuenfte disziplin: Kunst und praxis
der lernenden organisation, 2. Aufl., Stuttgart.
Skyrme, D. J. (1998). Measuring the value of knowledge.
Business Intelligence.
A Measurement Model to Identify Knowledge-intensive Business Processes in SMEs
139